Posttranslational modifications in proteins: resources, tools and prediction methods
- PMID: 33826699
- PMCID: PMC8040245
- DOI: 10.1093/database/baab012
Posttranslational modifications in proteins: resources, tools and prediction methods
Abstract
Posttranslational modifications (PTMs) refer to amino acid side chain modification in some proteins after their biosynthesis. There are more than 400 different types of PTMs affecting many aspects of protein functions. Such modifications happen as crucial molecular regulatory mechanisms to regulate diverse cellular processes. These processes have a significant impact on the structure and function of proteins. Disruption in PTMs can lead to the dysfunction of vital biological processes and hence to various diseases. High-throughput experimental methods for discovery of PTMs are very laborious and time-consuming. Therefore, there is an urgent need for computational methods and powerful tools to predict PTMs. There are vast amounts of PTMs data, which are publicly accessible through many online databases. In this survey, we comprehensively reviewed the major online databases and related tools. The current challenges of computational methods were reviewed in detail as well.
© The Author(s) 2021. Published by Oxford University Press. All rights reserved. For permissions, please e-mail: journals.permissions@oup.com.
Figures
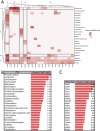
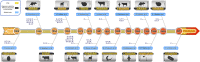

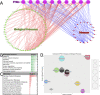
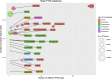
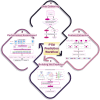
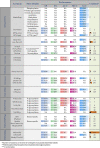
Similar articles
-
A Systematic Review on Posttranslational Modification in Proteins: Feature Construction, Algorithm and Webserver.Protein Pept Lett. 2018;25(9):807-814. doi: 10.2174/0929866525666180925151720. Protein Pept Lett. 2018. PMID: 30255739
-
Web-Based Computational Tools for the Prediction and Analysis of Posttranslational Modifications of Proteins.Methods Mol Biol. 2019;1934:1-20. doi: 10.1007/978-1-4939-9055-9_1. Methods Mol Biol. 2019. PMID: 31256369
-
dbPTM in 2022: an updated database for exploring regulatory networks and functional associations of protein post-translational modifications.Nucleic Acids Res. 2022 Jan 7;50(D1):D471-D479. doi: 10.1093/nar/gkab1017. Nucleic Acids Res. 2022. PMID: 34788852 Free PMC article.
-
Software eyes for protein post-translational modifications.Mass Spectrom Rev. 2015 Mar-Apr;34(2):133-47. doi: 10.1002/mas.21425. Epub 2014 Jun 2. Mass Spectrom Rev. 2015. PMID: 24889695 Review.
-
Proteomic analysis and prediction of amino acid variations that influence protein posttranslational modifications.Brief Bioinform. 2019 Sep 27;20(5):1597-1606. doi: 10.1093/bib/bby036. Brief Bioinform. 2019. PMID: 29788276 Review.
Cited by
-
Druggable Metabolic Vulnerabilities Are Exposed and Masked during Progression to Castration Resistant Prostate Cancer.Biomolecules. 2022 Oct 28;12(11):1590. doi: 10.3390/biom12111590. Biomolecules. 2022. PMID: 36358940 Free PMC article. Review.
-
Post-Translational Modifications of ATG4B in the Regulation of Autophagy.Cells. 2022 Apr 13;11(8):1330. doi: 10.3390/cells11081330. Cells. 2022. PMID: 35456009 Free PMC article. Review.
-
Recombinant Spider Silk: Promises and Bottlenecks.Front Bioeng Biotechnol. 2022 Mar 8;10:835637. doi: 10.3389/fbioe.2022.835637. eCollection 2022. Front Bioeng Biotechnol. 2022. PMID: 35350182 Free PMC article. Review.
-
Exploring the Role of Posttranslational Modifications in Spinal and Bulbar Muscular Atrophy.Front Mol Neurosci. 2022 Jun 3;15:931301. doi: 10.3389/fnmol.2022.931301. eCollection 2022. Front Mol Neurosci. 2022. PMID: 35726299 Free PMC article. Review.
-
MILKSHAKE Western blot and Sundae ELISA: We all scream for better antibody validation.J Immunol Methods. 2023 Oct;521:113540. doi: 10.1016/j.jim.2023.113540. Epub 2023 Aug 18. J Immunol Methods. 2023. PMID: 37597727 Free PMC article.
References
-
- Ramazi, S., Allahverdi, A. and Zahiri, J. (2020) Evaluation of post-translational modifications in histone proteins: a review on histone modification defects in developmental and neurological disorders. J. Biosci., 45, 135. - PubMed
-
- Mann, M. and Jensen, O.N. (2003) Proteomic analysis of post-translational modifications. Nat. Biotechnol., 21, 255–261. - PubMed
-
- Xu, Y. and Chou, K.-C. (2016) Recent progress in predicting posttranslational modification sites in proteins. Curr. Top. Med. Chem., 16, 591–603. - PubMed
-
- Blom, N., Sicheritz-Pontén, T., Gupta, R.. et al. (2004) Prediction of post-translational glycosylation and phosphorylation of proteins from the amino acid sequence. Proteomics, 4, 1633–1649. - PubMed
MeSH terms
Substances
LinkOut - more resources
Full Text Sources
Other Literature Sources
Miscellaneous