Weighted minimum feedback vertex sets and implementation in human cancer genes detection
- PMID: 33752597
- PMCID: PMC7986389
- DOI: 10.1186/s12859-021-04062-2
Weighted minimum feedback vertex sets and implementation in human cancer genes detection
Abstract
Background: Recently, many computational methods have been proposed to predict cancer genes. One typical kind of method is to find the differentially expressed genes between tumour and normal samples. However, there are also some genes, for example, 'dark' genes, that play important roles at the network level but are difficult to find by traditional differential gene expression analysis. In addition, network controllability methods, such as the minimum feedback vertex set (MFVS) method, have been used frequently in cancer gene prediction. However, the weights of vertices (or genes) are ignored in the traditional MFVS methods, leading to difficulty in finding the optimal solution because of the existence of many possible MFVSs.
Results: Here, we introduce a novel method, called weighted MFVS (WMFVS), which integrates the gene differential expression value with MFVS to select the maximum-weighted MFVS from all possible MFVSs in a protein interaction network. Our experimental results show that WMFVS achieves better performance than using traditional bio-data or network-data analyses alone.
Conclusion: This method balances the advantage of differential gene expression analyses and network analyses, improves the low accuracy of differential gene expression analyses and decreases the instability of pure network analyses. Furthermore, WMFVS can be easily applied to various kinds of networks, providing a useful framework for data analysis and prediction.
Keywords: Cancer gene; Differential gene expression; Feedback vertex set.
Conflict of interest statement
TA is an Associate Editor of BMC Bioinformatics.
Figures
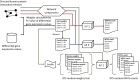
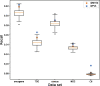
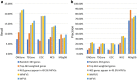
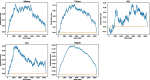
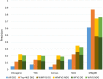
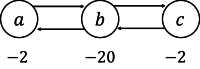
Similar articles
-
Analysis of Critical and Redundant Vertices in Controlling Directed Complex Networks Using Feedback Vertex Sets.J Comput Biol. 2018 Oct;25(10):1071-1090. doi: 10.1089/cmb.2018.0019. Epub 2018 Aug 3. J Comput Biol. 2018. PMID: 30074414
-
BMRF-MI: integrative identification of protein interaction network by modeling the gene dependency.BMC Genomics. 2015;16 Suppl 7(Suppl 7):S10. doi: 10.1186/1471-2164-16-S7-S10. Epub 2015 Jun 11. BMC Genomics. 2015. PMID: 26099273 Free PMC article.
-
DECODE: an integrated differential co-expression and differential expression analysis of gene expression data.BMC Bioinformatics. 2015 May 31;16:182. doi: 10.1186/s12859-015-0582-4. BMC Bioinformatics. 2015. PMID: 26026612 Free PMC article.
-
A novel network control model for identifying personalized driver genes in cancer.PLoS Comput Biol. 2019 Nov 25;15(11):e1007520. doi: 10.1371/journal.pcbi.1007520. eCollection 2019 Nov. PLoS Comput Biol. 2019. PMID: 31765387 Free PMC article.
-
KatzDriver: A network based method to cancer causal genes discovery in gene regulatory network.Biosystems. 2021 Mar;201:104326. doi: 10.1016/j.biosystems.2020.104326. Epub 2020 Dec 10. Biosystems. 2021. PMID: 33309969
Cited by
-
scGET: Predicting Cell Fate Transition During Early Embryonic Development by Single-cell Graph Entropy.Genomics Proteomics Bioinformatics. 2021 Jun;19(3):461-474. doi: 10.1016/j.gpb.2020.11.008. Epub 2021 Dec 24. Genomics Proteomics Bioinformatics. 2021. PMID: 34954425 Free PMC article.
-
Whole-Brain Evaluation of Cortical Microconnectomes.eNeuro. 2023 Oct 30;10(10):ENEURO.0094-23.2023. doi: 10.1523/ENEURO.0094-23.2023. Print 2023 Oct. eNeuro. 2023. PMID: 37903612 Free PMC article.
-
SWEET: a single-sample network inference method for deciphering individual features in disease.Brief Bioinform. 2023 Mar 19;24(2):bbad032. doi: 10.1093/bib/bbad032. Brief Bioinform. 2023. PMID: 36719112 Free PMC article.
References
MeSH terms
Grants and funding
- N/A/Ministry of Education
- 18H04113/Japan Society for the Promotion of Science
- 109-2636-B-009-007/Ministry of Science and Technology, Taiwan
- N/A/Center for Intelligent Drug Systems and Smart Bio-devices
- N/A/International Collaborative Research Program of Institute for Chemical Research, Kyoto University
LinkOut - more resources
Full Text Sources
Other Literature Sources
Molecular Biology Databases