Progressive immune dysfunction with advancing disease stage in renal cell carcinoma
- PMID: 33711273
- PMCID: PMC8138872
- DOI: 10.1016/j.ccell.2021.02.013
Progressive immune dysfunction with advancing disease stage in renal cell carcinoma
Abstract
The tumor immune microenvironment plays a critical role in cancer progression and response to immunotherapy in clear cell renal cell carcinoma (ccRCC), yet the composition and phenotypic states of immune cells in this tumor are incompletely characterized. We performed single-cell RNA and T cell receptor sequencing on 164,722 individual cells from tumor and adjacent non-tumor tissue in patients with ccRCC across disease stages: early, locally advanced, and advanced/metastatic. Terminally exhausted CD8+ T cells were enriched in metastatic disease and were restricted in T cell receptor diversity. Within the myeloid compartment, pro-inflammatory macrophages were decreased, and suppressive M2-like macrophages were increased in advanced disease. Terminally exhausted CD8+ T cells and M2-like macrophages co-occurred in advanced disease and expressed ligands and receptors that support T cell dysfunction and M2-like polarization. This immune dysfunction circuit is associated with a worse prognosis in external cohorts and identifies potentially targetable immune inhibitory pathways in ccRCC.
Keywords: CD8 T cell exhaustion; cancer immunotherapy; cell-cell interaction; clear cell renal cell carcinoma; immune cell atlas; single-cell RNA sequencing; tumor-associated macrophages.
Copyright © 2021 Elsevier Inc. All rights reserved.
Conflict of interest statement
Declaration of interests D.A.B. reports nonfinancial support from Bristol Myers Squibb, honoraria from LM Education/Exchange Services, and personal fees from Octane Global, Defined Health, Dedham Group, Adept Field Solutions, Slingshot Insights, Blueprint Partnerships, Charles River Associates, Trinity Group, and Insight Strategy, outside of the submitted work. S.H.G. has patents licensed to Novalgen Inc, outside of the submitted work. Z.B. reports nonfinancial support from Bristol Myers Squibb and Genentech/imCore. D.B.K. has acted as an advisor to and has received consulting fees from Neon Therapeutics and owns equity in Agenus, Armata pharmaceuticals, Breakbio, Biomarin Pharmaceutical, Bristol Myers Squibb, Celldex Therapeutics, Chinook Therapeutics, Editas Medicine, Exelixis, Gilead Sciences, IMV, Lexicon Pharmaceuticals, Moderna, and Regeneron Pharmaceuticals. K.M.M. reports research support from Bristol Myers Squibb. B.A.M. reports consulting fees from Bayer, Astellas, Astra Zeneca, Seattle Genetics, Dendreon, Calithera, Exelixis, Nektar, Pfizer, Janssen, Genentech, Eisai, and EMD Serono, and research support to DFCI from Bristol Myers Squibb, Calithera, Exelixis, Seattle Genetics. D.F.M. has received consulting fees from Bristol Myers Squibb, Pfizer, Merck, Alkermes, EMD Serono, Eli Lilly, Iovance, and Eisai, and research support from Bristol Myers Squibb, Merck, Genentech, Pfizer, Exelixis, X4 Pharma, and Alkermes. S.A.S. reports nonfinancial support from Bristol Myers Squibb outside the submitted work. S.A.S. previously advised and has received consulting fees from Neon Therapeutics. S.A.S. reports nonfinancial support from Bristol Myers Squibb, and equity in Agenus Inc., Agios Pharmaceuticals, Breakbio Corp., Bristol Myers Squibb, and Lumos Pharma, outside the submitted work. S.S. reported personal fees from Merck, AstraZeneca, Bristol Myers Squibb, CRISPR Therapeutics AG, AACR, and NCI, grants from Bristol Myers Squibb, AstraZeneca, Novartis, and Exelixis, and royalties from Biogenex. A.H.S. has patents/pending royalties on the PD-1 pathway from Roche and Novartis. A.H.S. is on advisory boards for Bicara, Janssen Immunology, Surface Oncology, Elstar, SQZ Biotechnologies, Elpiscience, Selecta, and Monopteros, and consults for Novartis. A.H.S. has received research funding from AbbVie, Novartis, Roche, UCB, Ipsen, Quark, and Merck. D.F.M. reports personal fees from Bristol Myers Squibb, Pfizer, Merck, Novartis, Exelixis, Array BioPharm, Genentech, Alkermes, Jounce Therapeutics, X4 Pharma, Peloton, EMD Serono, and Eli Lilly, and research support from Bristol Myers Squibb, Prometheus Laboratories, Merck, Genentech, Pfizer, Exelixis, Novartis, X4 Pharma, Alkermes, and Peloton. T.K.C. reports grants and personal fees from Astra Zeneca; personal fees from Bayer; grants and personal fees from Bristol Myers Squibb; personal fees from Cerulean; grants and personal fees from Eisai; personal fees from Foundation Medicine Inc.; grants and personal fees from Exelixis; grants and personal fees from Genentech; personal fees from Roche; grants and personal fees from GlaxoSmithKline; grants and personal fees from Merck, from Novartis, Peloton, and Pfizer; personal fees from Prometheus Labs; grants and personal fees from Corvus; personal fees from Ipsen; grants from Tracon; and grants from Astellas outside the submitted work. The other authors declare no potential conflicts of interest. C.J.W. is an equity holder of BioNTech.
Figures
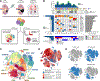
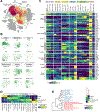
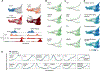
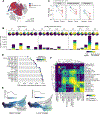
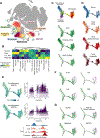
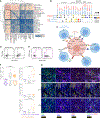
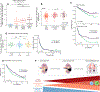
Comment in
-
Seeing the forest for the trees-single-cell atlases link CD8+ T cells and macrophages to disease progression and treatment response in kidney cancer.Cancer Cell. 2021 May 10;39(5):594-596. doi: 10.1016/j.ccell.2021.03.008. Epub 2021 Apr 15. Cancer Cell. 2021. PMID: 33861995
-
Immune dysfunction and reprogramming in RCC.Nat Rev Urol. 2021 Jun;18(6):321. doi: 10.1038/s41585-021-00471-3. Nat Rev Urol. 2021. PMID: 33953372 No abstract available.
Similar articles
-
Renal Cell Carcinoma-Infiltrating CD3low Vγ9Vδ1 T Cells Represent Potentially Novel Anti-Tumor Immune Players.Curr Issues Mol Biol. 2021 May 27;43(1):226-239. doi: 10.3390/cimb43010019. Curr Issues Mol Biol. 2021. PMID: 34071865 Free PMC article.
-
Intratumoral CXCL13+CD8+T cell infiltration determines poor clinical outcomes and immunoevasive contexture in patients with clear cell renal cell carcinoma.J Immunother Cancer. 2021 Feb;9(2):e001823. doi: 10.1136/jitc-2020-001823. J Immunother Cancer. 2021. PMID: 33589528 Free PMC article.
-
Hexokinase 3 dysfunction promotes tumorigenesis and immune escape by upregulating monocyte/macrophage infiltration into the clear cell renal cell carcinoma microenvironment.Int J Biol Sci. 2021 Jun 1;17(9):2205-2222. doi: 10.7150/ijbs.58295. eCollection 2021. Int J Biol Sci. 2021. PMID: 34239350 Free PMC article.
-
Importance of Multiparametric Evaluation of Immune-Related T-Cell Markers in Renal-Cell Carcinoma.Clin Genitourin Cancer. 2019 Dec;17(6):e1147-e1152. doi: 10.1016/j.clgc.2019.07.021. Epub 2019 Aug 5. Clin Genitourin Cancer. 2019. PMID: 31473121 Review.
-
Roles of the Dynamic Tumor Immune Microenvironment in the Individualized Treatment of Advanced Clear Cell Renal Cell Carcinoma.Front Immunol. 2021 Mar 4;12:653358. doi: 10.3389/fimmu.2021.653358. eCollection 2021. Front Immunol. 2021. PMID: 33746989 Free PMC article. Review.
Cited by
-
Fcγ receptors and immunomodulatory antibodies in cancer.Nat Rev Cancer. 2024 Jan;24(1):51-71. doi: 10.1038/s41568-023-00637-8. Epub 2023 Dec 7. Nat Rev Cancer. 2024. PMID: 38062252 Review.
-
Tumor-associated macrophages expressing the transcription factor IRF8 promote T cell exhaustion in cancer.Immunity. 2022 Nov 8;55(11):2044-2058.e5. doi: 10.1016/j.immuni.2022.10.002. Epub 2022 Oct 25. Immunity. 2022. PMID: 36288724 Free PMC article.
-
ADGRE5-centered Tsurv model in T cells recognizes responders to neoadjuvant cancer immunotherapy.Front Immunol. 2024 Jan 26;15:1304183. doi: 10.3389/fimmu.2024.1304183. eCollection 2024. Front Immunol. 2024. PMID: 38343549 Free PMC article.
-
Immunomodulatory response to neoadjuvant nivolumab in non-metastatic clear cell renal cell carcinoma.Sci Rep. 2024 Jan 17;14(1):1458. doi: 10.1038/s41598-024-51889-9. Sci Rep. 2024. PMID: 38228729 Free PMC article.
-
Integrated TCR repertoire analysis and single-cell transcriptomic profiling of tumor-infiltrating T cells in renal cell carcinoma identifies shared and tumor-restricted expanded clones with unique phenotypes.Front Oncol. 2022 Sep 14;12:952252. doi: 10.3389/fonc.2022.952252. eCollection 2022. Front Oncol. 2022. PMID: 36185254 Free PMC article.
References
-
- Amin MB, American Joint Committee on Cancer., and American Cancer Society. (2017). AJCC cancer staging manual, Eight edition / editor-in-chief, Amin Mahul B.; editors, Edge Stephen B. and 16 others; Gress Donna M. - Technical editor; Meyer Laura R. - Managing editor. edn (Chicago IL: American Joint Committee on Cancer, Springer; ).
Publication types
MeSH terms
Substances
Grants and funding
LinkOut - more resources
Full Text Sources
Other Literature Sources
Medical
Research Materials