Data-driven detection of subtype-specific differentially expressed genes
- PMID: 33432005
- PMCID: PMC7801594
- DOI: 10.1038/s41598-020-79704-1
Data-driven detection of subtype-specific differentially expressed genes
Abstract
Among multiple subtypes of tissue or cell, subtype-specific differentially-expressed genes (SDEGs) are defined as being most-upregulated in only one subtype but not in any other. Detecting SDEGs plays a critical role in the molecular characterization and deconvolution of multicellular complex tissues. Classic differential analysis assumes a null hypothesis whose test statistic is not subtype-specific, thus can produce a high false positive rate and/or lower detection power. Here we first introduce a One-Versus-Everyone Fold Change (OVE-FC) test for detecting SDEGs. We then propose a scaled test statistic (OVE-sFC) for assessing the statistical significance of SDEGs that applies a mixture null distribution model and a tailored permutation test. The OVE-FC/sFC test was validated on both type 1 error rate and detection power using extensive simulation data sets generated from real gene expression profiles of purified subtype samples. The OVE-FC/sFC test was then applied to two benchmark gene expression data sets of purified subtype samples and detected many known or previously unknown SDEGs. Subsequent supervised deconvolution results on synthesized bulk expression data, obtained using the SDEGs detected from the independent purified expression data by the OVE-FC/sFC test, showed superior performance in deconvolution accuracy when compared with popular peer methods.
Conflict of interest statement
The authors declare no competing interests.
Figures
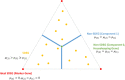
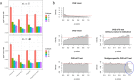
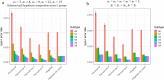
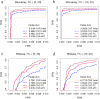
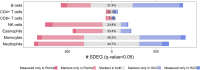
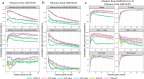
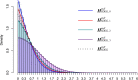
Similar articles
-
Comparison of seven methods for producing Affymetrix expression scores based on False Discovery Rates in disease profiling data.BMC Bioinformatics. 2005 Feb 10;6:26. doi: 10.1186/1471-2105-6-26. BMC Bioinformatics. 2005. PMID: 15705192 Free PMC article.
-
Construction of null statistics in permutation-based multiple testing for multi-factorial microarray experiments.Bioinformatics. 2006 Jun 15;22(12):1486-94. doi: 10.1093/bioinformatics/btl109. Epub 2006 Mar 30. Bioinformatics. 2006. PMID: 16574697
-
Improved cell composition deconvolution method of bulk gene expression profiles to quantify subsets of immune cells.BMC Med Genomics. 2019 Dec 20;12(Suppl 8):169. doi: 10.1186/s12920-019-0613-5. BMC Med Genomics. 2019. PMID: 31856824 Free PMC article.
-
Improving sensitivity of linear regression-based cell type-specific differential expression deconvolution with per-gene vs. global significance threshold.BMC Bioinformatics. 2016 Oct 6;17(Suppl 13):334. doi: 10.1186/s12859-016-1226-z. BMC Bioinformatics. 2016. PMID: 27766949 Free PMC article.
-
A note on using permutation-based false discovery rate estimates to compare different analysis methods for microarray data.Bioinformatics. 2005 Dec 1;21(23):4280-8. doi: 10.1093/bioinformatics/bti685. Epub 2005 Sep 27. Bioinformatics. 2005. PMID: 16188930
Cited by
-
ABDS: a bioinformatics tool suite for analyzing biologically diverse samples.Res Sq [Preprint]. 2024 May 30:rs.3.rs-4419408. doi: 10.21203/rs.3.rs-4419408/v1. Res Sq. 2024. PMID: 38853832 Free PMC article. Preprint.
-
drGAT: Attention-Guided Gene Assessment of Drug Response Utilizing a Drug-Cell-Gene Heterogeneous Network.ArXiv [Preprint]. 2024 May 14:arXiv:2405.08979v1. ArXiv. 2024. PMID: 38800657 Free PMC article. Preprint.
-
Uncertainty Quantification and Interpretability for Clinical Trial Approval Prediction.Health Data Sci. 2024 Apr 15;4:0126. doi: 10.34133/hds.0126. eCollection 2024. Health Data Sci. 2024. PMID: 38645573 Free PMC article.
-
CLINICAL HETEROGENEITY IN THE AGE OF BIG DATA, ADVANCED ANALYTICS, AND COMPLEXITY THEORY.Trans Am Clin Climatol Assoc. 2023;133:56-68. Trans Am Clin Climatol Assoc. 2023. PMID: 37701617 Free PMC article.
-
PASSer2.0: Accurate Prediction of Protein Allosteric Sites Through Automated Machine Learning.Front Mol Biosci. 2022 Jul 11;9:879251. doi: 10.3389/fmolb.2022.879251. eCollection 2022. Front Mol Biosci. 2022. PMID: 35898310 Free PMC article.
References
-
- Yu G, et al. Matched gene selection and committee classifier for molecular classification of heterogeneous diseases. J. Mach. Learn. Res. 2010;11:2141–2167.
Publication types
MeSH terms
Grants and funding
LinkOut - more resources
Full Text Sources
Other Literature Sources