Integrative analysis of long extracellular RNAs reveals a detection panel of noncoding RNAs for liver cancer
- PMID: 33391469
- PMCID: PMC7681086
- DOI: 10.7150/thno.48206
Integrative analysis of long extracellular RNAs reveals a detection panel of noncoding RNAs for liver cancer
Abstract
Rationale: Long extracellular RNAs (exRNAs) in plasma can be profiled by new sequencing technologies, even with low abundance. However, cancer-related exRNAs and their variations remain understudied. Methods: We investigated different variations (i.e. differential expression, alternative splicing, alternative polyadenylation, and differential editing) in diverse long exRNA species (e.g. long noncoding RNAs and circular RNAs) using 79 plasma exosomal RNA-seq (exoRNA-seq) datasets of multiple cancer types. We then integrated 53 exoRNA-seq datasets and 65 self-profiled cell-free RNA-seq (cfRNA-seq) datasets to identify recurrent variations in liver cancer patients. We further combined TCGA tissue RNA-seq datasets and validated biomarker candidates by RT-qPCR in an individual cohort of more than 100 plasma samples. Finally, we used machine learning models to identify a signature of 3 noncoding RNAs for the detection of liver cancer. Results: We found that different types of RNA variations identified from exoRNA-seq data were enriched in pathways related to tumorigenesis and metastasis, immune, and metabolism, suggesting that cancer signals can be detected from long exRNAs. Subsequently, we identified more than 100 recurrent variations in plasma from liver cancer patients by integrating exoRNA-seq and cfRNA-seq datasets. From these datasets, 5 significantly up-regulated long exRNAs were confirmed by TCGA data and validated by RT-qPCR in an independent cohort. When using machine learning models to combine two of these validated circular and structured RNAs (SNORD3B-1, circ-0080695) with a miRNA (miR-122) as a panel to classify liver cancer patients from healthy donors, the average AUROC of the cross-validation was 89.4%. The selected 3-RNA panel successfully detected 79.2% AFP-negative samples and 77.1% early-stage liver cancer samples in the testing and validation sets. Conclusions: Our study revealed that different types of RNA variations related to cancer can be detected in plasma and identified a 3-RNA detection panel for liver cancer, especially for AFP-negative and early-stage patients.
Keywords: RNA biomarker; cancer; circular RNA; extracellular RNA; liquid biopsy; noncoding RNA.
© The author(s).
Conflict of interest statement
Competing Interests: The authors have declared that no competing interest exists.
Figures
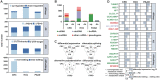
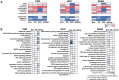
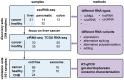
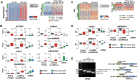
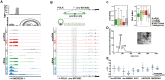
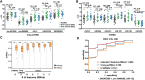
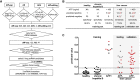
Similar articles
-
A comprehensive evaluation of full-spectrum cell-free RNAs highlights cell-free RNA fragments for early-stage hepatocellular carcinoma detection.EBioMedicine. 2023 Jul;93:104645. doi: 10.1016/j.ebiom.2023.104645. Epub 2023 Jun 12. EBioMedicine. 2023. PMID: 37315449 Free PMC article.
-
Extracellular RNA in a single droplet of human serum reflects physiologic and disease states.Proc Natl Acad Sci U S A. 2019 Sep 17;116(38):19200-19208. doi: 10.1073/pnas.1908252116. Epub 2019 Sep 3. Proc Natl Acad Sci U S A. 2019. PMID: 31481608 Free PMC article.
-
Noncoding RNAs Serve as Diagnosis and Prognosis Biomarkers for Hepatocellular Carcinoma.Clin Chem. 2019 Jul;65(7):905-915. doi: 10.1373/clinchem.2018.301150. Epub 2019 Apr 17. Clin Chem. 2019. PMID: 30996051
-
The potential of circulating exosomal RNA biomarkers in cancer.Expert Rev Mol Diagn. 2020 Jul;20(7):665-678. doi: 10.1080/14737159.2020.1745064. Epub 2020 Apr 2. Expert Rev Mol Diagn. 2020. PMID: 32188269 Review.
-
Circulating RNAs in prostate cancer patients.Cancer Lett. 2022 Jan 1;524:57-69. doi: 10.1016/j.canlet.2021.10.011. Epub 2021 Oct 14. Cancer Lett. 2022. PMID: 34656688 Review.
Cited by
-
The development and validation of a novel senescence-related long-chain non-coding RNA (lncRNA) signature that predicts prognosis and the tumor microenvironment of patients with hepatocellular carcinoma.Ann Transl Med. 2022 Jul;10(14):766. doi: 10.21037/atm-22-3348. Ann Transl Med. 2022. PMID: 35965795 Free PMC article.
-
A novel cuproptosis-related lncRNA signature predicts prognosis and therapeutic response in bladder cancer.Front Genet. 2023 Jan 4;13:1082691. doi: 10.3389/fgene.2022.1082691. eCollection 2022. Front Genet. 2023. PMID: 36685947 Free PMC article.
-
(In)Distinctive Role of Long Non-Coding RNAs in Common and Rare Ovarian Cancers.Cancers (Basel). 2021 Oct 9;13(20):5040. doi: 10.3390/cancers13205040. Cancers (Basel). 2021. PMID: 34680193 Free PMC article. Review.
-
Construction of a Ferroptosis-Related Nine-lncRNA Signature for Predicting Prognosis and Immune Response in Hepatocellular Carcinoma.Front Immunol. 2021 Sep 17;12:719175. doi: 10.3389/fimmu.2021.719175. eCollection 2021. Front Immunol. 2021. PMID: 34603293 Free PMC article.
-
Exploring Unique Extracellular Vesicles Associated Signatures: Prognostic Insights, Immune Microenvironment Dynamics, and Therapeutic Responses in Pancreatic Adenocarcinoma.Mediators Inflamm. 2024 Aug 24;2024:2825971. doi: 10.1155/2024/2825971. eCollection 2024. Mediators Inflamm. 2024. PMID: 39220187 Free PMC article.
References
-
- Valadi H, Ekstrom K, Bossios A, Sjostrand M, Lee JJ, Lotvall JO. Exosome-mediated transfer of mRNAs and microRNAs is a novel mechanism of genetic exchange between cells. Nat Cell Biol. 2007;9:654–9. - PubMed
Publication types
MeSH terms
Substances
LinkOut - more resources
Full Text Sources
Other Literature Sources
Medical