Longitudinal Multi-omics Analyses Identify Responses of Megakaryocytes, Erythroid Cells, and Plasmablasts as Hallmarks of Severe COVID-19
- PMID: 33296687
- PMCID: PMC7689306
- DOI: 10.1016/j.immuni.2020.11.017
Longitudinal Multi-omics Analyses Identify Responses of Megakaryocytes, Erythroid Cells, and Plasmablasts as Hallmarks of Severe COVID-19
Abstract
Temporal resolution of cellular features associated with a severe COVID-19 disease trajectory is needed for understanding skewed immune responses and defining predictors of outcome. Here, we performed a longitudinal multi-omics study using a two-center cohort of 14 patients. We analyzed the bulk transcriptome, bulk DNA methylome, and single-cell transcriptome (>358,000 cells, including BCR profiles) of peripheral blood samples harvested from up to 5 time points. Validation was performed in two independent cohorts of COVID-19 patients. Severe COVID-19 was characterized by an increase of proliferating, metabolically hyperactive plasmablasts. Coinciding with critical illness, we also identified an expansion of interferon-activated circulating megakaryocytes and increased erythropoiesis with features of hypoxic signaling. Megakaryocyte- and erythroid-cell-derived co-expression modules were predictive of fatal disease outcome. The study demonstrates broad cellular effects of SARS-CoV-2 infection beyond adaptive immune cells and provides an entry point toward developing biomarkers and targeted treatments of patients with COVID-19.
Keywords: COVID-19; RNA-seq; acute respiratory distress; blood; disease trajectory; immune response; infectious disease; methylation; scRNA-seq; virus.
Copyright © 2020 Elsevier Inc. All rights reserved.
Conflict of interest statement
Declaration of Interests The authors declare no conflicting interests.
Figures
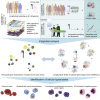
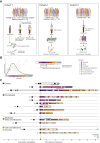
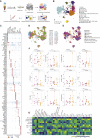
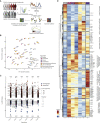
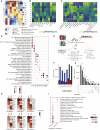
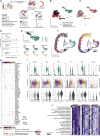
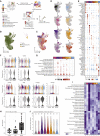
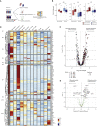
Similar articles
-
Dynamic blood single-cell immune responses in patients with COVID-19.Signal Transduct Target Ther. 2021 Mar 6;6(1):110. doi: 10.1038/s41392-021-00526-2. Signal Transduct Target Ther. 2021. PMID: 33677468 Free PMC article.
-
Immune Response in Severe and Non-Severe Coronavirus Disease 2019 (COVID-19) Infection: A Mechanistic Landscape.Front Immunol. 2021 Oct 13;12:738073. doi: 10.3389/fimmu.2021.738073. eCollection 2021. Front Immunol. 2021. PMID: 34721400 Free PMC article.
-
COVID-19 immune features revealed by a large-scale single-cell transcriptome atlas.Cell. 2021 Apr 1;184(7):1895-1913.e19. doi: 10.1016/j.cell.2021.01.053. Epub 2021 Feb 3. Cell. 2021. PMID: 33657410 Free PMC article.
-
Dysregulated Immune Responses in COVID-19 Patients Correlating With Disease Severity and Invasive Oxygen Requirements.Front Immunol. 2021 Oct 21;12:769059. doi: 10.3389/fimmu.2021.769059. eCollection 2021. Front Immunol. 2021. PMID: 34745145 Free PMC article.
-
Multiomics: unraveling the panoramic landscapes of SARS-CoV-2 infection.Cell Mol Immunol. 2021 Oct;18(10):2313-2324. doi: 10.1038/s41423-021-00754-0. Epub 2021 Sep 1. Cell Mol Immunol. 2021. PMID: 34471261 Free PMC article. Review.
Cited by
-
Longitudinal transcriptional changes reveal genes from the natural killer cell-mediated cytotoxicity pathway as critical players underlying COVID-19 progression.Elife. 2024 Oct 29;13:RP94242. doi: 10.7554/eLife.94242. Elife. 2024. PMID: 39470726 Free PMC article.
-
Ozone: complicated effects in central nervous system diseases.Med Gas Res. 2025 Mar 1;15(1):44-57. doi: 10.4103/mgr.MEDGASRES-D-24-00005. Epub 2024 Oct 2. Med Gas Res. 2025. PMID: 39436168 Free PMC article. Review.
-
Human-augmented large language model-driven selection of glutathione peroxidase 4 as a candidate blood transcriptional biomarker for circulating erythroid cells.Sci Rep. 2024 Oct 5;14(1):23225. doi: 10.1038/s41598-024-73916-5. Sci Rep. 2024. PMID: 39369090 Free PMC article.
-
Clonal landscape of autoantibody-secreting plasmablasts in COVID-19 patients.Life Sci Alliance. 2024 Sep 17;7(12):e202402774. doi: 10.26508/lsa.202402774. Print 2024 Dec. Life Sci Alliance. 2024. PMID: 39288992 Free PMC article.
-
Gut microbiota dysbiosis is associated with altered tryptophan metabolism and dysregulated inflammatory response in COVID-19.NPJ Biofilms Microbiomes. 2024 Aug 1;10(1):66. doi: 10.1038/s41522-024-00538-0. NPJ Biofilms Microbiomes. 2024. PMID: 39085233 Free PMC article.
References
-
- Alexa A., Rahnenführer J., Lengauer T. Improved scoring of functional groups from gene expression data by decorrelating GO graph structure. Bioinformatics. 2006;22:1600–1607. - PubMed
Publication types
MeSH terms
Substances
Grants and funding
LinkOut - more resources
Full Text Sources
Other Literature Sources
Medical
Miscellaneous