Statistical Approach for Biologically Relevant Gene Selection from High-Throughput Gene Expression Data
- PMID: 33286973
- PMCID: PMC7712650
- DOI: 10.3390/e22111205
Statistical Approach for Biologically Relevant Gene Selection from High-Throughput Gene Expression Data
Abstract
Selection of biologically relevant genes from high-dimensional expression data is a key research problem in gene expression genomics. Most of the available gene selection methods are either based on relevancy or redundancy measure, which are usually adjudged through post selection classification accuracy. Through these methods the ranking of genes was conducted on a single high-dimensional expression data, which led to the selection of spuriously associated and redundant genes. Hence, we developed a statistical approach through combining a support vector machine with Maximum Relevance and Minimum Redundancy under a sound statistical setup for the selection of biologically relevant genes. Here, the genes were selected through statistical significance values and computed using a nonparametric test statistic under a bootstrap-based subject sampling model. Further, a systematic and rigorous evaluation of the proposed approach with nine existing competitive methods was carried on six different real crop gene expression datasets. This performance analysis was carried out under three comparison settings, i.e., subject classification, biological relevant criteria based on quantitative trait loci and gene ontology. Our analytical results showed that the proposed approach selects genes which are more biologically relevant as compared to the existing methods. Moreover, the proposed approach was also found to be better with respect to the competitive existing methods. The proposed statistical approach provides a framework for combining filter and wrapper methods of gene selection.
Keywords: MRMR; SVM; biological relevance; bootstrap; gene expression; subject classification.
Conflict of interest statement
The authors declare no conflict of interest.
Figures
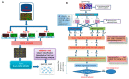
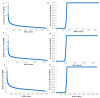
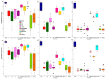
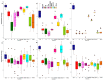
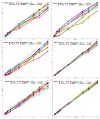
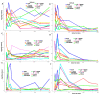
Similar articles
-
Statistical approach for selection of biologically informative genes.Gene. 2018 May 20;655:71-83. doi: 10.1016/j.gene.2018.02.044. Epub 2018 Feb 16. Gene. 2018. PMID: 29458166
-
SVM-RFE with MRMR filter for gene selection.IEEE Trans Nanobioscience. 2010 Mar;9(1):31-7. doi: 10.1109/TNB.2009.2035284. Epub 2009 Oct 30. IEEE Trans Nanobioscience. 2010. PMID: 19884101
-
A TRIZ-inspired bat algorithm for gene selection in cancer classification.Genomics. 2020 Jan;112(1):114-126. doi: 10.1016/j.ygeno.2019.09.015. Epub 2019 Oct 30. Genomics. 2020. PMID: 31676302
-
Hybrid Feature Selection Algorithm mRMR-ICA for Cancer Classification from Microarray Gene Expression Data.Comb Chem High Throughput Screen. 2018;21(6):420-430. doi: 10.2174/1386207321666180601074349. Comb Chem High Throughput Screen. 2018. PMID: 29852866
-
Filter versus wrapper gene selection approaches in DNA microarray domains.Artif Intell Med. 2004 Jun;31(2):91-103. doi: 10.1016/j.artmed.2004.01.007. Artif Intell Med. 2004. PMID: 15219288 Review.
Cited by
-
Single-cell transcriptomics: background, technologies, applications, and challenges.Mol Biol Rep. 2024 Apr 30;51(1):600. doi: 10.1007/s11033-024-09553-y. Mol Biol Rep. 2024. PMID: 38689046 Review.
-
Differential Expression Analysis of Single-Cell RNA-Seq Data: Current Statistical Approaches and Outstanding Challenges.Entropy (Basel). 2022 Jul 18;24(7):995. doi: 10.3390/e24070995. Entropy (Basel). 2022. PMID: 35885218 Free PMC article. Review.
-
A Framework for Comparison and Assessment of Synthetic RNA-Seq Data.Genes (Basel). 2022 Dec 14;13(12):2362. doi: 10.3390/genes13122362. Genes (Basel). 2022. PMID: 36553629 Free PMC article.
-
Multigroup prediction in lung cancer patients and comparative controls using signature of volatile organic compounds in breath samples.PLoS One. 2022 Nov 30;17(11):e0277431. doi: 10.1371/journal.pone.0277431. eCollection 2022. PLoS One. 2022. PMID: 36449484 Free PMC article.
-
Statistical Approach of Gene Set Analysis with Quantitative Trait Loci for Crop Gene Expression Studies.Entropy (Basel). 2021 Jul 23;23(8):945. doi: 10.3390/e23080945. Entropy (Basel). 2021. PMID: 34441085 Free PMC article.
References
-
- Charpe A.M. Advances in Biotechnology. Springer; New Delhi, India: 2014. DNA Microarray; pp. 71–104. - DOI
-
- Das S., Meher P.K., Rai A., Bhar L.M., Mandal B.N. Statistical approaches for gene selection, hub gene identification and module interaction in gene co-expression network analysis: An application to aluminum stress in soybean (Glycine max L.) PLoS ONE. 2017;12:e0169605. doi: 10.1371/journal.pone.0169605. - DOI - PMC - PubMed
Grants and funding
LinkOut - more resources
Full Text Sources