Genomic and drug target evaluation of 90 cardiovascular proteins in 30,931 individuals
- PMID: 33067605
- PMCID: PMC7611474
- DOI: 10.1038/s42255-020-00287-2
Genomic and drug target evaluation of 90 cardiovascular proteins in 30,931 individuals
Abstract
Circulating proteins are vital in human health and disease and are frequently used as biomarkers for clinical decision-making or as targets for pharmacological intervention. Here, we map and replicate protein quantitative trait loci (pQTL) for 90 cardiovascular proteins in over 30,000 individuals, resulting in 451 pQTLs for 85 proteins. For each protein, we further perform pathway mapping to obtain trans-pQTL gene and regulatory designations. We substantiate these regulatory findings with orthogonal evidence for trans-pQTLs using mouse knockdown experiments (ABCA1 and TRIB1) and clinical trial results (chemokine receptors CCR2 and CCR5), with consistent regulation. Finally, we evaluate known drug targets, and suggest new target candidates or repositioning opportunities using Mendelian randomization. This identifies 11 proteins with causal evidence of involvement in human disease that have not previously been targeted, including EGF, IL-16, PAPPA, SPON1, F3, ADM, CASP-8, CHI3L1, CXCL16, GDF15 and MMP-12. Taken together, these findings demonstrate the utility of large-scale mapping of the genetics of the proteome and provide a resource for future precision studies of circulating proteins in human health.
Conflict of interest statement
The other authors declare no competing interests
Figures
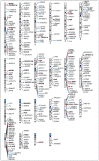
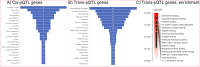
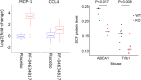
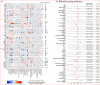
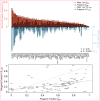
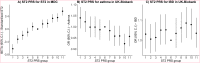
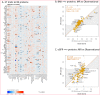
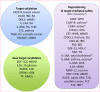
Similar articles
-
Causal roles and clinical utility of cardiovascular proteins in colorectal cancer risk: a multi-modal study integrating mendelian randomization, expression profiling, and survival analysis.BMC Med Genomics. 2024 May 22;17(1):138. doi: 10.1186/s12920-024-01909-4. BMC Med Genomics. 2024. PMID: 38778378 Free PMC article.
-
Genetics of circulating inflammatory proteins identifies drivers of immune-mediated disease risk and therapeutic targets.Nat Immunol. 2023 Sep;24(9):1540-1551. doi: 10.1038/s41590-023-01588-w. Epub 2023 Aug 10. Nat Immunol. 2023. PMID: 37563310 Free PMC article.
-
An Integrative Genomic Strategy Identifies sRAGE as a Causal and Protective Biomarker of Lung Function.Chest. 2022 Jan;161(1):76-84. doi: 10.1016/j.chest.2021.06.053. Epub 2021 Jul 6. Chest. 2022. PMID: 34237330 Free PMC article.
-
Immunochip Meta-Analysis of Inflammatory Bowel Disease Identifies Three Novel Loci and Four Novel Associations in Previously Reported Loci.J Crohns Colitis. 2018 May 25;12(6):730-741. doi: 10.1093/ecco-jcc/jjy002. J Crohns Colitis. 2018. PMID: 29584801 Free PMC article. Review.
-
Trouble With Tribbles-1.Arterioscler Thromb Vasc Biol. 2019 Jun;39(6):998-1005. doi: 10.1161/ATVBAHA.118.311573. Arterioscler Thromb Vasc Biol. 2019. PMID: 31018662 Free PMC article. Review.
Cited by
-
Role of interleukin-18 in mediating the impacts of celiac disease on osteoporosis: a Mendelian randomization study.Front Immunol. 2024 Oct 9;15:1453657. doi: 10.3389/fimmu.2024.1453657. eCollection 2024. Front Immunol. 2024. PMID: 39445015 Free PMC article.
-
Association Between Serum Galectin-3 and Parkinson's Disease: A Two-Sample Mendelian Randomization Study.Brain Behav. 2024 Oct;14(10):e70103. doi: 10.1002/brb3.70103. Brain Behav. 2024. PMID: 39444071 Free PMC article.
-
A Mendelian randomization study on causal relationship between metabolic factors and abnormal spermatozoa.Transl Androl Urol. 2024 Sep 30;13(9):2005-2015. doi: 10.21037/tau-24-187. Epub 2024 Sep 13. Transl Androl Urol. 2024. PMID: 39434741 Free PMC article.
-
Integrating metabolomics and proteomics to identify novel drug targets for heart failure and atrial fibrillation.Genome Med. 2024 Oct 21;16(1):120. doi: 10.1186/s13073-024-01395-4. Genome Med. 2024. PMID: 39434187 Free PMC article.
-
A comprehensive study of genetic regulation and disease associations of plasma circulatory microRNAs using population-level data.Genome Biol. 2024 Oct 21;25(1):276. doi: 10.1186/s13059-024-03420-6. Genome Biol. 2024. PMID: 39434104 Free PMC article.
References
Publication types
MeSH terms
Substances
Grants and funding
- MR/L003120/1/MRC_/Medical Research Council/United Kingdom
- CZB/4/710/CSO_/Chief Scientist Office/United Kingdom
- MR/S004068/1/MRC_/Medical Research Council/United Kingdom
- MC_UU_00011/1/MRC_/Medical Research Council/United Kingdom
- MR/S004068/2/MRC_/Medical Research Council/United Kingdom
- 637640/ERC_/European Research Council/International
- 335395/ERC_/European Research Council/International
- ARC_/Arthritis Research UK/United Kingdom
- MC_PC_U127592696/MRC_/Medical Research Council/United Kingdom
- CZB/4/276/CSO_/Chief Scientist Office/United Kingdom
- WT_/Wellcome Trust/United Kingdom
- 204979/Z/16/Z/WT_/Wellcome Trust/United Kingdom
- RG/13/13/30194/BHF_/British Heart Foundation/United Kingdom
- SP/09/002/BHF_/British Heart Foundation/United Kingdom
- MR/S003746/1/MRC_/Medical Research Council/United Kingdom
- R01 HL139731/HL/NHLBI NIH HHS/United States
- BTRU-2014-10024/DH_/Department of Health/United Kingdom
- FS/18/23/33512/BHF_/British Heart Foundation/United Kingdom
- RG/18/13/33946/BHF_/British Heart Foundation/United Kingdom
- MC_UU_00007/10/MRC_/Medical Research Council/United Kingdom
LinkOut - more resources
Full Text Sources
Other Literature Sources
Research Materials
Miscellaneous