Predicting binding sites from unbound versus bound protein structures
- PMID: 32985584
- PMCID: PMC7522209
- DOI: 10.1038/s41598-020-72906-7
Predicting binding sites from unbound versus bound protein structures
Abstract
We present the application of seven binding-site prediction algorithms to a meticulously curated dataset of ligand-bound and ligand-free crystal structures for 304 unique protein sequences (2528 crystal structures). We probe the influence of starting protein structures on the results of binding-site prediction, so the dataset contains a minimum of two ligand-bound and two ligand-free structures for each protein. We use this dataset in a brief survey of five geometry-based, one energy-based, and one machine-learning-based methods: Surfnet, Ghecom, LIGSITEcsc, Fpocket, Depth, AutoSite, and Kalasanty. Distributions of the F scores and Matthew's correlation coefficients for ligand-bound versus ligand-free structure performance show no statistically significant difference in structure type versus performance for most methods. Only Fpocket showed a statistically significant but low magnitude enhancement in performance for holo structures. Lastly, we found that most methods will succeed on some crystal structures and fail on others within the same protein family, despite all structures being relatively high-quality structures with low structural variation. We expected better consistency across varying protein conformations of the same sequence. Interestingly, the success or failure of a given structure cannot be predicted by quality metrics such as resolution, Cruickshank Diffraction Precision index, or unresolved residues. Cryptic sites were also examined.
Conflict of interest statement
The authors declare no competing interests.
Figures
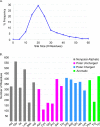
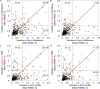
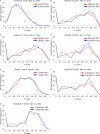
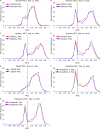
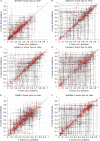
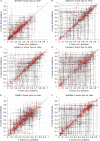
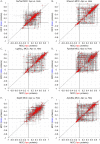
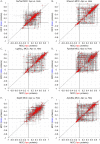
Similar articles
-
LIGSITEcsc: predicting ligand binding sites using the Connolly surface and degree of conservation.BMC Struct Biol. 2006 Sep 24;6:19. doi: 10.1186/1472-6807-6-19. BMC Struct Biol. 2006. PMID: 16995956 Free PMC article.
-
AHoJ-DB: A PDB-wide Assignment of apo & holo Relationships Based on Individual Protein-Ligand Interactions.J Mol Biol. 2024 Sep 1;436(17):168545. doi: 10.1016/j.jmb.2024.168545. Epub 2024 Mar 18. J Mol Biol. 2024. PMID: 38508305
-
Identification of cavities on protein surface using multiple computational approaches for drug binding site prediction.Bioinformatics. 2011 Aug 1;27(15):2083-8. doi: 10.1093/bioinformatics/btr331. Epub 2011 Jun 2. Bioinformatics. 2011. PMID: 21636590
-
In silico prediction of binding sites on proteins.Curr Med Chem. 2010;17(15):1550-62. doi: 10.2174/092986710790979944. Curr Med Chem. 2010. PMID: 20166931 Review.
-
DeepPocket: Ligand Binding Site Detection and Segmentation using 3D Convolutional Neural Networks.J Chem Inf Model. 2022 Nov 14;62(21):5069-5079. doi: 10.1021/acs.jcim.1c00799. Epub 2021 Aug 10. J Chem Inf Model. 2022. PMID: 34374539 Review.
Cited by
-
DeepBSRPred: deep learning-based binding site residue prediction for proteins.Amino Acids. 2023 Oct;55(10):1305-1316. doi: 10.1007/s00726-022-03228-3. Epub 2022 Dec 27. Amino Acids. 2023. PMID: 36574037
-
Scoring Functions for Protein-Ligand Binding Affinity Prediction using Structure-Based Deep Learning: A Review.Front Bioinform. 2022 Jun 17;2:885983. doi: 10.3389/fbinf.2022.885983. Front Bioinform. 2022. PMID: 36187180 Free PMC article.
-
Membrane Lipids Are an Integral Part of Transmembrane Allosteric Sites in GPCRs: A Case Study of Cannabinoid CB1 Receptor Bound to a Negative Allosteric Modulator, ORG27569, and Analogs.J Med Chem. 2022 Sep 22;65(18):12240-12255. doi: 10.1021/acs.jmedchem.2c00946. Epub 2022 Sep 6. J Med Chem. 2022. PMID: 36066412 Free PMC article.
-
AlphaFold2 protein structure prediction: Implications for drug discovery.Curr Opin Struct Biol. 2023 Feb;78:102526. doi: 10.1016/j.sbi.2022.102526. Epub 2023 Jan 6. Curr Opin Struct Biol. 2023. PMID: 36621153 Free PMC article. Review.
-
PDBspheres: a method for finding 3D similarities in local regions in proteins.NAR Genom Bioinform. 2022 Oct 10;4(4):lqac078. doi: 10.1093/nargab/lqac078. eCollection 2022 Dec. NAR Genom Bioinform. 2022. PMID: 36225529 Free PMC article.
References
-
- Xie Z-R, Hwang M-J. Molecular Modeling of Proteins Methods in Molecular Biology. New York: Humana Press; 2015. pp. 383–398.
Publication types
MeSH terms
Substances
Grants and funding
LinkOut - more resources
Full Text Sources