Computational Characterization of Antibody-Excipient Interactions for Rational Excipient Selection Using the Site Identification by Ligand Competitive Saturation-Biologics Approach
- PMID: 32965126
- PMCID: PMC7606568
- DOI: 10.1021/acs.molpharmaceut.0c00775
Computational Characterization of Antibody-Excipient Interactions for Rational Excipient Selection Using the Site Identification by Ligand Competitive Saturation-Biologics Approach
Abstract
Protein therapeutics typically require a concentrated protein formulation, which can lead to self-association and/or high viscosity due to protein-protein interaction (PPI). Excipients are often added to improve stability, bioavailability, and manufacturability of the protein therapeutics, but the selection of excipients often relies on trial and error. Therefore, understanding the excipient-protein interaction and its effect on non-specific PPI is important for rational selection of formulation development. In this study, we validate a general workflow based on the site identification by ligand competitive saturation (SILCS) technology, termed SILCS-Biologics, that can be applied to protein therapeutics for rational excipient selection. The National Institute of Standards and Technology monoclonal antibody (NISTmAb) reference along with the CNTO607 mAb is used as model antibody proteins to examine PPIs, and NISTmAb was used to further examine excipient-protein interactions, in silico. Metrics from SILCS include the distribution and predicted affinity of excipients, buffer interactions with the NISTmAb Fab, and the relation of the interactions to predicted PPI. Comparison with a range of experimental data showed multiple SILCS metrics to be predictive. Specifically, the number of favorable sites to which an excipient binds and the number of sites to which an excipient binds that are involved in predicted PPIs correlate with the experimentally determined viscosity. In addition, a combination of the number of binding sites and the predicted binding affinity is indicated to be predictive of relative protein stability. Comparison of arginine, trehalose, and sucrose, all of which give the highest viscosity in combination with analysis of B22 and kD and the SILCS metrics, indicates that higher viscosities are associated with a low number of predicted binding sites, with lower binding affinity of arginine leading to its anomalously high impact on viscosity. The present study indicates the potential for the SILCS-Biologics approach to be of utility in the rational design of excipients during biologics formulation.
Keywords: biologics; formulation; molecular dynamics; monoclonal antibody; protein-based drugs; protein−protein interactions.
Conflict of interest statement
Conflict of Interest
ADM Jr is co-founder and Chief Scientific Officer of SilcsBio, LLC. SJ is an employee of SilcsBio LLC when the studies were performed.
Figures
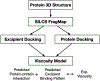
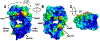
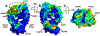
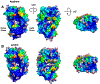
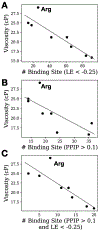
Similar articles
-
Studying Excipient Modulated Physical Stability and Viscosity of Monoclonal Antibody Formulations Using Small-Angle Scattering.Mol Pharm. 2019 Oct 7;16(10):4319-4338. doi: 10.1021/acs.molpharmaceut.9b00687. Epub 2019 Sep 24. Mol Pharm. 2019. PMID: 31487466
-
Toward Biotherapeutics Formulation Composition Engineering using Site-Identification by Ligand Competitive Saturation (SILCS).J Pharm Sci. 2021 Mar;110(3):1103-1110. doi: 10.1016/j.xphs.2020.10.051. Epub 2020 Nov 1. J Pharm Sci. 2021. PMID: 33137372 Free PMC article.
-
Site Identification by Ligand Competitive Saturation-Biologics Approach for Structure-Based Protein Charge Prediction.Mol Pharm. 2023 May 1;20(5):2600-2611. doi: 10.1021/acs.molpharmaceut.3c00064. Epub 2023 Apr 5. Mol Pharm. 2023. PMID: 37017675 Free PMC article.
-
NMR spectroscopy as a characterization tool enabling biologics formulation development.J Pharm Biomed Anal. 2023 Jan 20;223:115110. doi: 10.1016/j.jpba.2022.115110. Epub 2022 Oct 14. J Pharm Biomed Anal. 2023. PMID: 36308923 Review.
-
Histidine as a versatile excipient in the protein-based biopharmaceutical formulations.Int J Pharm. 2024 Sep 5;662:124472. doi: 10.1016/j.ijpharm.2024.124472. Epub 2024 Jul 14. Int J Pharm. 2024. PMID: 39013532 Review.
Cited by
-
Computer-Aided Drug Design: An Update.Methods Mol Biol. 2023;2601:123-152. doi: 10.1007/978-1-0716-2855-3_7. Methods Mol Biol. 2023. PMID: 36445582 Free PMC article.
-
Accelerating therapeutic protein design with computational approaches toward the clinical stage.Comput Struct Biotechnol J. 2023 Apr 29;21:2909-2926. doi: 10.1016/j.csbj.2023.04.027. eCollection 2023. Comput Struct Biotechnol J. 2023. PMID: 38213894 Free PMC article. Review.
-
Effects of Monovalent Salt on Protein-Protein Interactions of Dilute and Concentrated Monoclonal Antibody Formulations.Antibodies (Basel). 2022 Mar 31;11(2):24. doi: 10.3390/antib11020024. Antibodies (Basel). 2022. PMID: 35466277 Free PMC article.
-
Application of Site-Identification by Ligand Competitive Saturation in Computer-Aided Drug Design.New J Chem. 2022 Jan 21;46(3):919-932. doi: 10.1039/d1nj04028f. Epub 2021 Nov 29. New J Chem. 2022. PMID: 35210743 Free PMC article.
-
Mechanism of activation and the rewired network: New drug design concepts.Med Res Rev. 2022 Mar;42(2):770-799. doi: 10.1002/med.21863. Epub 2021 Oct 25. Med Res Rev. 2022. PMID: 34693559 Free PMC article. Review.
References
-
- Walsh G, Biopharmaceutical benchmarks 2010. Nat. Biotechnol 2010, 28 (9), 917–924. - PubMed
-
- Mullard A, 2016 FDA drug approvals. Nat. Rev. Drug Discov 2017, 16 (2), 73–76. - PubMed
-
- Mueller C; Altenburger U; Mohl S, Challenges for the pharmaceutical technical development of protein coformulations. J. Pharm. Pharmacol 2017, 70 (5), 666–674. - PubMed
Publication types
MeSH terms
Substances
Grants and funding
LinkOut - more resources
Full Text Sources