Investigation of gene-gene interactions in cardiac traits and serum fatty acid levels in the LURIC Health Study
- PMID: 32915819
- PMCID: PMC7485803
- DOI: 10.1371/journal.pone.0238304
Investigation of gene-gene interactions in cardiac traits and serum fatty acid levels in the LURIC Health Study
Abstract
Epistasis analysis elucidates the effects of gene-gene interactions (G×G) between multiple loci for complex traits. However, the large computational demands and the high multiple testing burden impede their discoveries. Here, we illustrate the utilization of two methods, main effect filtering based on individual GWAS results and biological knowledge-based modeling through Biofilter software, to reduce the number of interactions tested among single nucleotide polymorphisms (SNPs) for 15 cardiac-related traits and 14 fatty acids. We performed interaction analyses using the two filtering methods, adjusting for age, sex, body mass index (BMI), waist-hip ratio, and the first three principal components from genetic data, among 2,824 samples from the Ludwigshafen Risk and Cardiovascular (LURIC) Health Study. Using Biofilter, one interaction nearly met Bonferroni significance: an interaction between rs7735781 in XRCC4 and rs10804247 in XRCC5 was identified for venous thrombosis with a Bonferroni-adjusted likelihood ratio test (LRT) p: 0.0627. A total of 57 interactions were identified from main effect filtering for the cardiac traits G×G (10) and fatty acids G×G (47) at Bonferroni-adjusted LRT p < 0.05. For cardiac traits, the top interaction involved SNPs rs1383819 in SNTG1 and rs1493939 (138kb from 5' of SAMD12) with Bonferroni-adjusted LRT p: 0.0228 which was significantly associated with history of arterial hypertension. For fatty acids, the top interaction between rs4839193 in KCND3 and rs10829717 in LOC107984002 with Bonferroni-adjusted LRT p: 2.28×10-5 was associated with 9-trans 12-trans octadecanoic acid, an omega-6 trans fatty acid. The model inflation factor for the interactions under different filtering methods was evaluated from the standard median and the linear regression approach. Here, we applied filtering approaches to identify numerous genetic interactions related to cardiac-related outcomes as potential targets for therapy. The approaches described offer ways to detect epistasis in the complex traits and to improve precision medicine capability.
Conflict of interest statement
The authors have read the journal's policy and have the following competing interests: WZ and MEK are paid employees of The Synlab Holding Deutschland GmbH. There are no patents, products in development or marketed products associated with this research to declare. This does not alter our adherence to PLOS ONE policies on sharing data and materials.
Figures
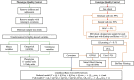
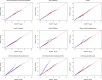
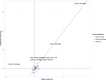
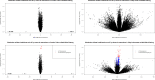
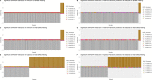
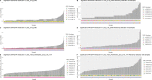
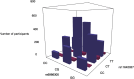
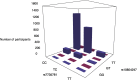
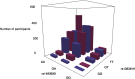
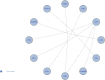
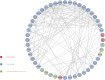
Similar articles
-
Next-generation analysis of cataracts: determining knowledge driven gene-gene interactions using biofilter, and gene-environment interactions using the Phenx Toolkit*.Pac Symp Biocomput. 2015:495-505. Pac Symp Biocomput. 2015. PMID: 25741542
-
Phenome-wide association studies on cardiovascular health and fatty acids considering phenotype quality control practices for epidemiological data.Pac Symp Biocomput. 2020;25:659-670. Pac Symp Biocomput. 2020. PMID: 31797636
-
Next-generation analysis of cataracts: determining knowledge driven gene-gene interactions using Biofilter, and gene-environment interactions using the PhenX Toolkit.Pac Symp Biocomput. 2013:147-58. Pac Symp Biocomput. 2013. Corrected and republished in: Pac Symp Biocomput. 2015:495-505. PMID: 23424120 Free PMC article. Corrected and republished.
-
Rationale and design of the LURIC study--a resource for functional genomics, pharmacogenomics and long-term prognosis of cardiovascular disease.Pharmacogenomics. 2001 Feb;2(1 Suppl 1):S1-73. doi: 10.1517/14622416.2.1.S1. Pharmacogenomics. 2001. PMID: 11258203 Review.
-
Brief Survey on Machine Learning in Epistasis.Methods Mol Biol. 2021;2212:169-179. doi: 10.1007/978-1-0716-0947-7_11. Methods Mol Biol. 2021. PMID: 33733356 Review.
Cited by
-
Genetic Contributors of Incident Stroke in 10,700 African Americans With Hypertension: A Meta-Analysis From the Genetics of Hypertension Associated Treatments and Reasons for Geographic and Racial Differences in Stroke Studies.Front Genet. 2021 Dec 21;12:781451. doi: 10.3389/fgene.2021.781451. eCollection 2021. Front Genet. 2021. PMID: 34992631 Free PMC article.
-
Statistical inference with large-scale trait imputation.Stat Med. 2024 Feb 20;43(4):625-641. doi: 10.1002/sim.9975. Epub 2023 Dec 1. Stat Med. 2024. PMID: 38038193
-
Fatty acids and risk of dilated cardiomyopathy: A two-sample Mendelian randomization study.Front Nutr. 2023 Feb 16;10:1068050. doi: 10.3389/fnut.2023.1068050. eCollection 2023. Front Nutr. 2023. PMID: 36875854 Free PMC article.
-
Using GWAS summary data to impute traits for genotyped individuals.HGG Adv. 2023 Apr 12;4(3):100197. doi: 10.1016/j.xhgg.2023.100197. eCollection 2023 Jul 13. HGG Adv. 2023. PMID: 37181332 Free PMC article.
References
Publication types
MeSH terms
Substances
Grants and funding
LinkOut - more resources
Full Text Sources
Research Materials
Miscellaneous