Classification and Graphical Analysis of Alzheimer's Disease and Its Prodromal Stage Using Multimodal Features From Structural, Diffusion, and Functional Neuroimaging Data and the APOE Genotype
- PMID: 32848713
- PMCID: PMC7406801
- DOI: 10.3389/fnagi.2020.00238
Classification and Graphical Analysis of Alzheimer's Disease and Its Prodromal Stage Using Multimodal Features From Structural, Diffusion, and Functional Neuroimaging Data and the APOE Genotype
Abstract
Graphical, voxel, and region-based analysis has become a popular approach to studying neurodegenerative disorders such as Alzheimer's disease (AD) and its prodromal stage [mild cognitive impairment (MCI)]. These methods have been used previously for classification or discrimination of AD in subjects in a prodromal stage called stable MCI (MCIs), which does not convert to AD but remains stable over a period of time, and converting MCI (MCIc), which converts to AD, but the results reported across similar studies are often inconsistent. Furthermore, the classification accuracy for MCIs vs. MCIc is limited. In this study, we propose combining different neuroimaging modalities (sMRI, FDG-PET, AV45-PET, DTI, and rs-fMRI) with the apolipoprotein-E genotype to form a multimodal system for the discrimination of AD, and to increase the classification accuracy. Initially, we used two well-known analyses to extract features from each neuroimage for the discrimination of AD: whole-brain parcelation analysis (or region-based analysis), and voxel-wise analysis (or voxel-based morphometry). We also investigated graphical analysis (nodal and group) for all six binary classification groups (AD vs. HC, MCIs vs. MCIc, AD vs. MCIc, AD vs. MCIs, HC vs. MCIc, and HC vs. MCIs). Data for a total of 129 subjects (33 AD, 30 MCIs, 31 MCIc, and 35 HCs) for each imaging modality were obtained from the Alzheimer's Disease Neuroimaging Initiative (ADNI) homepage. These data also include two APOE genotype data points for the subjects. Moreover, we used the 2-mm AICHA atlas with the NiftyReg registration toolbox to extract 384 brain regions from each PET (FDG and AV45) and sMRI image. For the rs-fMRI images, we used the DPARSF toolbox in MATLAB for the automatic extraction of data and the results for REHO, ALFF, and fALFF. We also used the pyClusterROI script for the automatic parcelation of each rs-fMRI image into 200 brain regions. For the DTI images, we used the FSL (Version 6.0) toolbox for the extraction of fractional anisotropy (FA) images to calculate a tract-based spatial statistic. Moreover, we used the PANDA toolbox to obtain 50 white-matter-region-parcellated FA images on the basis of the 2-mm JHU-ICBM-labeled template atlas. To integrate the different modalities and different complementary information into one form, and to optimize the classifier, we used the multiple kernel learning (MKL) framework. The obtained results indicated that our multimodal approach yields a significant improvement in accuracy over any single modality alone. The areas under the curve obtained by the proposed method were 97.78, 96.94, 95.56, 96.25, 96.67, and 96.59% for AD vs. HC, MCIs vs. MCIc, AD vs. MCIc, AD vs. MCIs, HC vs. MCIc, and HC vs. MCIs binary classification, respectively. Our proposed multimodal method improved the classification result for MCIs vs. MCIc groups compared with the unimodal classification results. Our study found that the (left/right) precentral region was present in all six binary classification groups (this region can be considered the most significant region). Furthermore, using nodal network topology, we found that FDG, AV45-PET, and rs-fMRI were the most important neuroimages, and showed many affected regions relative to other modalities. We also compared our results with recently published results.
Keywords: APOE genotype; AV45-PET; Alzheimer’s disease; DTI; FDG-PET; multimodal fusion; rs-fMRI; sMRI.
Copyright © 2020 Gupta, Kim, Kim and Kwon.
Figures
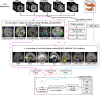
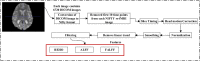
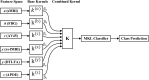
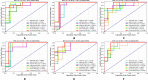
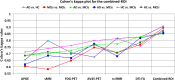
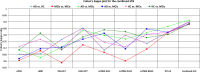
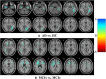
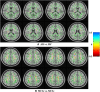
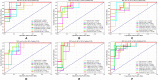
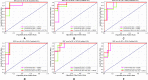
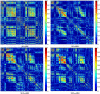
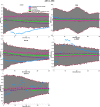
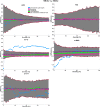
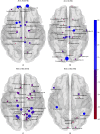
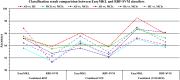
Similar articles
-
Prediction and Classification of Alzheimer's Disease Based on Combined Features From Apolipoprotein-E Genotype, Cerebrospinal Fluid, MR, and FDG-PET Imaging Biomarkers.Front Comput Neurosci. 2019 Oct 16;13:72. doi: 10.3389/fncom.2019.00072. eCollection 2019. Front Comput Neurosci. 2019. PMID: 31680923 Free PMC article.
-
Alzheimer's Disease Diagnosis and Biomarker Analysis Using Resting-State Functional MRI Functional Brain Network With Multi-Measures Features and Hippocampal Subfield and Amygdala Volume of Structural MRI.Front Aging Neurosci. 2022 May 30;14:818871. doi: 10.3389/fnagi.2022.818871. eCollection 2022. Front Aging Neurosci. 2022. PMID: 35707703 Free PMC article.
-
An Efficient Combination among sMRI, CSF, Cognitive Score, and APOE ε4 Biomarkers for Classification of AD and MCI Using Extreme Learning Machine.Comput Intell Neurosci. 2020 Jun 4;2020:8015156. doi: 10.1155/2020/8015156. eCollection 2020. Comput Intell Neurosci. 2020. PMID: 32565773 Free PMC article.
-
APOE-related biomarker profiles in non-pathological aging and early phases of Alzheimer's disease.Neurosci Biobehav Rev. 2013 Sep;37(8):1322-35. doi: 10.1016/j.neubiorev.2013.05.006. Epub 2013 May 20. Neurosci Biobehav Rev. 2013. PMID: 23701948 Review.
-
Function-structure associations of the brain: evidence from multimodal connectivity and covariance studies.Neuroimage. 2014 Nov 15;102 Pt 1:11-23. doi: 10.1016/j.neuroimage.2013.09.044. Epub 2013 Sep 29. Neuroimage. 2014. PMID: 24084066 Free PMC article. Review.
Cited by
-
18F-FDG PET can effectively rule out conversion to dementia and the presence of CSF biomarker of neurodegeneration: a real-world data analysis.Alzheimers Res Ther. 2024 Aug 13;16(1):182. doi: 10.1186/s13195-024-01535-3. Alzheimers Res Ther. 2024. PMID: 39135067 Free PMC article.
-
OViTAD: Optimized Vision Transformer to Predict Various Stages of Alzheimer's Disease Using Resting-State fMRI and Structural MRI Data.Brain Sci. 2023 Feb 3;13(2):260. doi: 10.3390/brainsci13020260. Brain Sci. 2023. PMID: 36831803 Free PMC article.
-
Feature Importance Analysis and Machine Learning for Alzheimer's Disease Early Detection: Feature Fusion of the Hippocampus, Entorhinal Cortex, and Standardized Uptake Value Ratio.Digit Biomark. 2024 Apr 22;8(1):59-74. doi: 10.1159/000538486. eCollection 2024 Jan-Dec. Digit Biomark. 2024. PMID: 38650695 Free PMC article.
-
Whole-brain structure-function coupling abnormalities in mild cognitive impairment: a study combining amplitude of low-frequency fluctuations and voxel-based morphometry.Front Neurosci. 2023 Jul 31;17:1236221. doi: 10.3389/fnins.2023.1236221. eCollection 2023. Front Neurosci. 2023. PMID: 37583417 Free PMC article.
-
White matter alterations in mild cognitive impairment revealed by meta-analysis of diffusion tensor imaging using tract-based spatial statistics.Brain Imaging Behav. 2023 Dec;17(6):639-651. doi: 10.1007/s11682-023-00791-5. Epub 2023 Sep 1. Brain Imaging Behav. 2023. PMID: 37656372
References
-
- Aiolli F., Donini M. (2015). EasyMKL: a scalable multiple kernel learning algorithm. Neurocomputing 169 215–224. 10.1016/j.neucom.2014.11.078 - DOI
LinkOut - more resources
Full Text Sources
Miscellaneous