Systematic determination of the mitochondrial proportion in human and mice tissues for single-cell RNA-sequencing data quality control
- PMID: 32840568
- PMCID: PMC8599307
- DOI: 10.1093/bioinformatics/btaa751
Systematic determination of the mitochondrial proportion in human and mice tissues for single-cell RNA-sequencing data quality control
Abstract
Motivation: Quality control (QC) is a critical step in single-cell RNA-seq (scRNA-seq) data analysis. Low-quality cells are removed from the analysis during the QC process to avoid misinterpretation of the data. An important QC metric is the mitochondrial proportion (mtDNA%), which is used as a threshold to filter out low-quality cells. Early publications in the field established a threshold of 5% and since then, it has been used as a default in several software packages for scRNA-seq data analysis, and adopted as a standard in many scRNA-seq studies. However, the validity of using a uniform threshold across different species, single-cell technologies, tissues and cell types has not been adequately assessed.
Results: We systematically analyzed 5 530 106 cells reported in 1349 annotated datasets available in the PanglaoDB database and found that the average mtDNA% in scRNA-seq data across human tissues is significantly higher than in mouse tissues. This difference is not confounded by the platform used to generate the data. Based on this finding, we propose new reference values of the mtDNA% for 121 tissues of mouse and 44 tissues of humans. In general, for mouse tissues, the 5% threshold performs well to distinguish between healthy and low-quality cells. However, for human tissues, the 5% threshold should be reconsidered as it fails to accurately discriminate between healthy and low-quality cells in 29.5% (13 of 44) tissues analyzed. We conclude that omitting the mtDNA% QC filter or adopting a suboptimal mtDNA% threshold may lead to erroneous biological interpretations of scRNA-seq data.
Availabilityand implementation: The code used to download datasets, perform the analyzes and produce the figures is available at https://github.com/dosorio/mtProportion.
Supplementary information: Supplementary data are available at Bioinformatics online.
© The Author(s) 2020. Published by Oxford University Press. All rights reserved. For permissions, please e-mail: journals.permissions@oup.com.
Figures
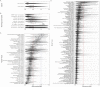
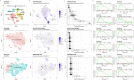
Similar articles
-
scQCEA: a framework for annotation and quality control report of single-cell RNA-sequencing data.BMC Genomics. 2023 Jul 6;24(1):381. doi: 10.1186/s12864-023-09447-6. BMC Genomics. 2023. PMID: 37415108 Free PMC article.
-
miQC: An adaptive probabilistic framework for quality control of single-cell RNA-sequencing data.PLoS Comput Biol. 2021 Aug 24;17(8):e1009290. doi: 10.1371/journal.pcbi.1009290. eCollection 2021 Aug. PLoS Comput Biol. 2021. PMID: 34428202 Free PMC article.
-
Cell-level somatic mutation detection from single-cell RNA sequencing.Bioinformatics. 2019 Nov 1;35(22):4679-4687. doi: 10.1093/bioinformatics/btz288. Bioinformatics. 2019. PMID: 31028395 Free PMC article.
-
Machine learning and statistical methods for clustering single-cell RNA-sequencing data.Brief Bioinform. 2020 Jul 15;21(4):1209-1223. doi: 10.1093/bib/bbz063. Brief Bioinform. 2020. PMID: 31243426 Review.
-
A Single-Cell Sequencing Guide for Immunologists.Front Immunol. 2018 Oct 23;9:2425. doi: 10.3389/fimmu.2018.02425. eCollection 2018. Front Immunol. 2018. PMID: 30405621 Free PMC article. Review.
Cited by
-
Single-cell RNA Sequencing Reveals How the Aryl Hydrocarbon Receptor Shapes Cellular Differentiation Potency in the Mouse Colon.Cancer Prev Res (Phila). 2022 Jan;15(1):17-28. doi: 10.1158/1940-6207.CAPR-21-0378. Epub 2021 Nov 22. Cancer Prev Res (Phila). 2022. PMID: 34815312 Free PMC article.
-
CHIT1 at diagnosis predicts faster disability progression and reflects early microglial activation in multiple sclerosis.Nat Commun. 2024 Jun 12;15(1):5013. doi: 10.1038/s41467-024-49312-y. Nat Commun. 2024. PMID: 38866782 Free PMC article.
-
Single-cell transcriptomics reveals lasting changes in the lung cellular landscape into adulthood after neonatal hyperoxic exposure.Redox Biol. 2021 Dec;48:102091. doi: 10.1016/j.redox.2021.102091. Epub 2021 Aug 16. Redox Biol. 2021. PMID: 34417156 Free PMC article.
-
Single-cell transcriptomics for the assessment of cardiac disease.Nat Rev Cardiol. 2023 May;20(5):289-308. doi: 10.1038/s41569-022-00805-7. Epub 2022 Dec 20. Nat Rev Cardiol. 2023. PMID: 36539452 Review.
-
Time-resolved single-cell RNAseq profiling identifies a novel Fabp5+ subpopulation of inflammatory myeloid cells with delayed cytotoxic profile in chronic spinal cord injury.Heliyon. 2023 Aug 6;9(8):e18339. doi: 10.1016/j.heliyon.2023.e18339. eCollection 2023 Aug. Heliyon. 2023. PMID: 37636454 Free PMC article.