Automatic Reconstruction of Mitochondria and Endoplasmic Reticulum in Electron Microscopy Volumes by Deep Learning
- PMID: 32792893
- PMCID: PMC7394701
- DOI: 10.3389/fnins.2020.00599
Automatic Reconstruction of Mitochondria and Endoplasmic Reticulum in Electron Microscopy Volumes by Deep Learning
Abstract
Together, mitochondria and the endoplasmic reticulum (ER) occupy more than 20% of a cell's volume, and morphological abnormality may lead to cellular function disorders. With the rapid development of large-scale electron microscopy (EM), manual contouring and three-dimensional (3D) reconstruction of these organelles has previously been accomplished in biological studies. However, manual segmentation of mitochondria and ER from EM images is time consuming and thus unable to meet the demands of large data analysis. Here, we propose an automated pipeline for mitochondrial and ER reconstruction, including the mitochondrial and ER contact sites (MAMs). We propose a novel recurrent neural network to detect and segment mitochondria and a fully residual convolutional network to reconstruct the ER. Based on the sparse distribution of synapses, we use mitochondrial context information to rectify the local misleading results and obtain 3D mitochondrial reconstructions. The experimental results demonstrate that the proposed method achieves state-of-the-art performance.
Keywords: 3D reconstruction; electron microscopes; endoplasmic reticulum; mitochondria; segmentation.
Copyright © 2020 Liu, Li, Yang, Hong, Chen, Xie and Han.
Figures

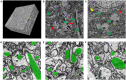
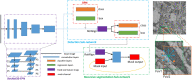
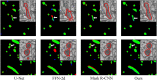
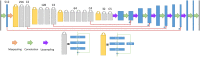
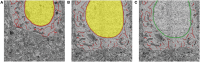
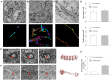
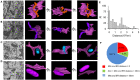
Similar articles
-
Automatic Mitochondria Segmentation for EM Data Using a 3D Supervised Convolutional Network.Front Neuroanat. 2018 Nov 2;12:92. doi: 10.3389/fnana.2018.00092. eCollection 2018. Front Neuroanat. 2018. PMID: 30450040 Free PMC article.
-
Effective automated pipeline for 3D reconstruction of synapses based on deep learning.BMC Bioinformatics. 2018 Jul 13;19(1):263. doi: 10.1186/s12859-018-2232-0. BMC Bioinformatics. 2018. PMID: 30005590 Free PMC article.
-
Three-dimensional organization of the endoplasmic reticulum membrane around the mitochondrial constriction site in mammalian cells revealed by using focused-ion beam tomography.Microscopy (Oxf). 2014 Nov;63 Suppl 1:i34. doi: 10.1093/jmicro/dfu076. Microscopy (Oxf). 2014. PMID: 25359839
-
The roles of mitochondria-associated membranes in mitochondrial quality control under endoplasmic reticulum stress.Life Sci. 2019 Aug 15;231:116587. doi: 10.1016/j.lfs.2019.116587. Epub 2019 Jun 18. Life Sci. 2019. PMID: 31220526 Review.
-
VoxResNet: Deep voxelwise residual networks for brain segmentation from 3D MR images.Neuroimage. 2018 Apr 15;170:446-455. doi: 10.1016/j.neuroimage.2017.04.041. Epub 2017 Apr 23. Neuroimage. 2018. PMID: 28445774 Review.
Cited by
-
A semi-automatic method for extracting mitochondrial cristae characteristics from 3D focused ion beam scanning electron microscopy data.Commun Biol. 2024 Mar 28;7(1):377. doi: 10.1038/s42003-024-06045-4. Commun Biol. 2024. PMID: 38548849 Free PMC article.
-
VGG-UNet/VGG-SegNet Supported Automatic Segmentation of Endoplasmic Reticulum Network in Fluorescence Microscopy Images.Scanning. 2022 Jun 8;2022:7733860. doi: 10.1155/2022/7733860. eCollection 2022. Scanning. 2022. PMID: 35800206 Free PMC article.
-
Quantifying Regulated Mitochondrial Fission in Macrophages.Methods Mol Biol. 2022;2523:281-301. doi: 10.1007/978-1-0716-2449-4_18. Methods Mol Biol. 2022. PMID: 35759204
-
Integrated Array Tomography for 3D Correlative Light and Electron Microscopy.Front Mol Biosci. 2022 Jan 19;8:822232. doi: 10.3389/fmolb.2021.822232. eCollection 2021. Front Mol Biosci. 2022. PMID: 35127826 Free PMC article.
-
Instance segmentation of mitochondria in electron microscopy images with a generalist deep learning model trained on a diverse dataset.Cell Syst. 2023 Jan 18;14(1):58-71.e5. doi: 10.1016/j.cels.2022.12.006. Cell Syst. 2023. PMID: 36657391 Free PMC article.
References
-
- Agus M., Calì C., Al-Awami A., Gobbetti E., Magistretti P., Hadwiger M. (2019). Interactive volumetric visual analysis of glycogen-derived energy absorption in nanometric brain structures. Comput. Graph. Forum 38, 427–439. 10.1111/cgf.13700 - DOI
-
- Calì C., Agus M., Kare K., Boges D. J., Lehväslaiho H., Hadwiger M., et al. . (2019). 3D cellular reconstruction of cortical glia and parenchymal morphometric analysis from serial block-face electron microscopy of juvenile rat. Prog. Neurobiol. 183:101696. 10.1016/j.pneurobio.2019.101696 - DOI - PubMed
LinkOut - more resources
Full Text Sources