Portable Automated Surveillance of Surgical Site Infections Using Natural Language Processing: Development and Validation
- PMID: 32773639
- PMCID: PMC9040555
- DOI: 10.1097/SLA.0000000000004133
Portable Automated Surveillance of Surgical Site Infections Using Natural Language Processing: Development and Validation
Abstract
Objectives: We present the development and validation of a portable NLP approach for automated surveillance of SSIs.
Summary of background data: The surveillance of SSIs is labor-intensive limiting the generalizability and scalability of surgical quality surveillance programs.
Methods: We abstracted patient clinical text notes after surgical procedures from 2 independent healthcare systems using different electronic healthcare records. An SSI detected as part of the American College of Surgeons' National Surgical Quality Improvement Program was used as the reference standard. We developed a rules-based NLP system (Easy Clinical Information Extractor [CIE]-SSI) for operative event-level detection of SSIs using an training cohort (4574 operative events) from 1 healthcare system and then conducted internal validation on a blind cohort from the same healthcare system (1850 operative events) and external validation on a blind cohort from the second healthcare system (15,360 operative events). EasyCIE-SSI performance was measured using sensitivity, specificity, and area under the receiver-operating-curve (AUC).
Results: The prevalence of SSI was 4% and 5% in the internal and external validation corpora. In internal validation, EasyCIE-SSI had a sensitivity, specificity, AUC of 94%, 88%, 0.912 for the detection of SSI, respectively. In external validation, EasyCIE-SSI had sensitivity, specificity, AUC of 79%, 92%, 0.852 for the detection of SSI, respectively. The sensitivity of EasyCIE-SSI decreased in clean, skin/subcutaneous, and outpatient procedures in the external validation compared to internal validation.
Conclusion: Automated surveillance of SSIs can be achieved using NLP of clinical notes with high sensitivity and specificity.
Conflict of interest statement
CONFLICT OF INTEREST DISCLOSURE
Dr. Chapman reported consulting for IBM and serving on the Scientific Advisory Board of Health Fidelity. These companies had no role in the study. No other disclosures are reported.
Figures
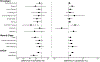
Comment in
-
"Say it Louder, Say it Clear" - Assessing the Utility of Natural Language Processing.Ann Surg. 2020 Oct;272(4):637-638. doi: 10.1097/SLA.0000000000004134. Ann Surg. 2020. PMID: 32932319 No abstract available.
Similar articles
-
Natural language processing for the surveillance of postoperative venous thromboembolism.Surgery. 2021 Oct;170(4):1175-1182. doi: 10.1016/j.surg.2021.04.027. Epub 2021 Jun 3. Surgery. 2021. PMID: 34090671 Free PMC article.
-
Detection of clinically important colorectal surgical site infection using Bayesian network.J Surg Res. 2017 Mar;209:168-173. doi: 10.1016/j.jss.2016.09.058. Epub 2016 Oct 5. J Surg Res. 2017. PMID: 28032554 Free PMC article.
-
Can We Geographically Validate a Natural Language Processing Algorithm for Automated Detection of Incidental Durotomy Across Three Independent Cohorts From Two Continents?Clin Orthop Relat Res. 2022 Sep 1;480(9):1766-1775. doi: 10.1097/CORR.0000000000002200. Epub 2022 Apr 12. Clin Orthop Relat Res. 2022. PMID: 35412473 Free PMC article.
-
Validation of an electronic tool for flagging surgical site infections based on clinical practice patterns for triaging surveillance: Operational successes and barriers.Am J Infect Control. 2018 Feb;46(2):186-190. doi: 10.1016/j.ajic.2017.08.026. Epub 2017 Oct 12. Am J Infect Control. 2018. PMID: 29031434
-
Applying Machine Learning Across Sites: External Validation of a Surgical Site Infection Detection Algorithm.J Am Coll Surg. 2021 Jun;232(6):963-971.e1. doi: 10.1016/j.jamcollsurg.2021.03.026. Epub 2021 Apr 5. J Am Coll Surg. 2021. PMID: 33831539 Free PMC article.
Cited by
-
Assessing the utility of deep neural networks in detecting superficial surgical site infections from free text electronic health record data.Front Digit Health. 2024 Jan 8;5:1249835. doi: 10.3389/fdgth.2023.1249835. eCollection 2023. Front Digit Health. 2024. PMID: 38259257 Free PMC article.
-
Recent technological advancements in Artificial Intelligence for orthopaedic wound management.J Clin Orthop Trauma. 2024 Oct 15;57:102561. doi: 10.1016/j.jcot.2024.102561. eCollection 2024 Oct. J Clin Orthop Trauma. 2024. PMID: 39502891
-
Development of machine learning models for the detection of surgical site infections following total hip and knee arthroplasty: a multicenter cohort study.Antimicrob Resist Infect Control. 2023 Sep 2;12(1):88. doi: 10.1186/s13756-023-01294-0. Antimicrob Resist Infect Control. 2023. PMID: 37658409 Free PMC article.
-
Development and evaluation of an interoperable natural language processing system for identifying pneumonia across clinical settings of care and institutions.JAMIA Open. 2022 Dec 30;5(4):ooac114. doi: 10.1093/jamiaopen/ooac114. eCollection 2022 Dec. JAMIA Open. 2022. PMID: 36601365 Free PMC article.
-
The augmented value of using clinical notes in semi-automated surveillance of deep surgical site infections after colorectal surgery.Antimicrob Resist Infect Control. 2023 Oct 26;12(1):117. doi: 10.1186/s13756-023-01316-x. Antimicrob Resist Infect Control. 2023. PMID: 37884948 Free PMC article.
References
-
- Ko CY, Hall BL, Hart AJ, et al. The American College of Surgeons National Surgical Quality Improvement Program: achieving better and safer surgery. Jt Comm J Qual Patient Saf 2015; 41(5):199–204. - PubMed
-
- Hu QL, Liu JY, Hobson DB, et al. Best Practices in Data Use for Achieving Successful Implementation of Enhanced Recovery Pathway. J Am Coll Surg 2019; 229(6):626–632.e1. - PubMed
-
- Classen DC, Griffin FA, Berwick DM. Measuring Patient Safety in Real Time: An Essential Method for Effectively Improving the Safety of Care. Ann Intern Med 2017; 167(12):882–883. - PubMed
-
- Ju MH, Ko CY, Hall BL, et al. A comparison of 2 surgical site infection monitoring systems. JAMA Surg 2015; 150(1):51–7. - PubMed
Publication types
MeSH terms
Grants and funding
LinkOut - more resources
Full Text Sources
Research Materials