Identification of a Glucose Metabolism-related Signature for prediction of Clinical Prognosis in Clear Cell Renal Cell Carcinoma
- PMID: 32742447
- PMCID: PMC7378912
- DOI: 10.7150/jca.45296
Identification of a Glucose Metabolism-related Signature for prediction of Clinical Prognosis in Clear Cell Renal Cell Carcinoma
Abstract
Background: Clear cell renal cell carcinoma (ccRCC) is one of the most prevalent and invasive histological subtypes among all renal cell carcinomas (RCC). Cancer cell metabolism, particularly glucose metabolism, has been reported as a hallmark of cancer. However, the characteristics of glucose metabolism-related gene sets in ccRCC have not been systematically profiled. Methods: In this study, we downloaded a gene expression profile and glucose metabolism-related gene set from TCGA (The Cancer Genome Altas) and MSigDB, respectively, to analyze the characteristics of glucose metabolism-related gene sets in ccRCC. We used a multivariable Cox regression analysis to develop a risk signature, which divided patients into low- and high- risk groups. In addition, a nomogram that combined the risk signature and clinical characteristics was created for predicting the 3- and 5-year overall survival (OS) of ccRCC. The accuracy of the nomogram prediction was evaluated using the area under the receiver operating characteristic curve (AUC) and a calibration plot. Results: A total of 231 glucose metabolism-related genes were found, and 68 differentially expressed genes (DEGs) were identified. After screening by univariate regression analysis, LASSO regression analysis and multivariable Cox regression analysis, six glucose metabolism-related DEGs (FBP1, GYG2, KAT2A, LGALS1, PFKP, and RGN) were selected to develop a risk signature. There were significant differences in the clinical features (Fuhrman nuclear grade and TNM stage) between the high- and low-risk groups. The multivariable Cox regression indicated that the risk score was independent of the prognostic factors (training set: HR=3.393, 95% CI [2.025, 5.685], p<0.001; validation set: HR=1.933, 95% CI [1.130, 3.308], p=0.016). The AUCs of the nomograms for the 3-year OS in the training and validation sets were 0.808 and 0.819, respectively, and 0.777 and 0.796, respectively, for the 5- year OS. Conclusion: We demonstrated a novel glucose metabolism-related risk signature for predicting the prognosis of ccRCC. However, additional in vitro and in vivo research is required to validate our findings.
Keywords: clear cell renal cell carcinoma; glucose metabolism; signature.
© The author(s).
Conflict of interest statement
Competing Interests: The authors have declared that no competing interest exists.
Figures
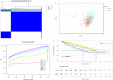
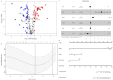
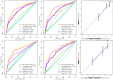
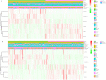
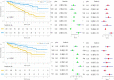
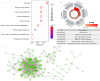
Similar articles
-
Profiles of overall survival-related gene expression-based risk signature and their prognostic implications in clear cell renal cell carcinoma.Biosci Rep. 2020 Sep 30;40(9):BSR20200492. doi: 10.1042/BSR20200492. Biosci Rep. 2020. PMID: 32789468 Free PMC article.
-
Construction of the metabolic reprogramming-associated gene signature for clear cell renal cell carcinoma prognosis prediction.BMC Urol. 2023 Sep 15;23(1):147. doi: 10.1186/s12894-023-01317-3. BMC Urol. 2023. PMID: 37715154 Free PMC article.
-
Construction of a novel gene-based model for prognosis prediction of clear cell renal cell carcinoma.Cancer Cell Int. 2020 Jan 28;20:27. doi: 10.1186/s12935-020-1113-6. eCollection 2020. Cancer Cell Int. 2020. PMID: 32002016 Free PMC article.
-
A DNA Damage Repair Gene Signature Associated With Immunotherapy Response and Clinical Prognosis in Clear Cell Renal Cell Carcinoma.Front Genet. 2022 May 17;13:798846. doi: 10.3389/fgene.2022.798846. eCollection 2022. Front Genet. 2022. PMID: 35656315 Free PMC article.
-
The Uniqueness of Clear Cell Renal Cell Carcinoma: Summary of the Process and Abnormality of Glucose Metabolism and Lipid Metabolism in ccRCC.Front Oncol. 2021 Sep 15;11:727778. doi: 10.3389/fonc.2021.727778. eCollection 2021. Front Oncol. 2021. PMID: 34604067 Free PMC article. Review.
Cited by
-
Identification of Metabolism-Related Gene-Based Subgroup in Prostate Cancer.Front Oncol. 2022 Jun 16;12:909066. doi: 10.3389/fonc.2022.909066. eCollection 2022. Front Oncol. 2022. PMID: 35785167 Free PMC article.
-
Development and external validation of a novel nomogram for predicting cancer-specific survival in patients with ascending colon adenocarcinoma after surgery: a population-based study.World J Surg Oncol. 2022 Apr 19;20(1):126. doi: 10.1186/s12957-022-02576-4. World J Surg Oncol. 2022. PMID: 35439983 Free PMC article.
-
Role of glycosphingolipid biosynthesis coregulators in malignant progression of thymoma.Int J Biol Sci. 2023 Aug 21;19(14):4442-4456. doi: 10.7150/ijbs.83468. eCollection 2023. Int J Biol Sci. 2023. PMID: 37781041 Free PMC article.
-
Optimized cell type signatures revealed from single-cell data by combining principal feature analysis, mutual information, and machine learning.Comput Struct Biotechnol J. 2023 Jun 5;21:3293-3314. doi: 10.1016/j.csbj.2023.06.002. eCollection 2023. Comput Struct Biotechnol J. 2023. PMID: 37333862 Free PMC article.
-
Prognostic Role of the Ubiquitin Proteasome System in Clear Cell Renal Cell Carcinoma: A Bioinformatic Perspective.J Cancer. 2021 May 13;12(14):4134-4147. doi: 10.7150/jca.53760. eCollection 2021. J Cancer. 2021. PMID: 34093816 Free PMC article.
References
-
- Bray F, Ferlay J, Soerjomataram I, Siegel RL, Torre LA, Jemal A. Global cancer statistics 2018: GLOBOCAN estimates of incidence and mortality worldwide for 36 cancers in 185 countries. CA Cancer J Clin. 2018;68(6):394–424. - PubMed
-
- Siegel RL, Miller KD, Jemal A. Cancer statistics, 2019. CA. CA Cancer J Clin. 2019;69(1):7–34. - PubMed
LinkOut - more resources
Full Text Sources
Research Materials
Miscellaneous