Constructing and deconstructing GATA2-regulated cell fate programs to establish developmental trajectories
- PMID: 32736380
- PMCID: PMC7596813
- DOI: 10.1084/jem.20191526
Constructing and deconstructing GATA2-regulated cell fate programs to establish developmental trajectories
Abstract
Stem and progenitor cell fate transitions constitute key decision points in organismal development that enable access to a developmental path or actively preclude others. Using the hematopoietic system, we analyzed the relative importance of cell fate-promoting mechanisms versus negating fate-suppressing mechanisms to engineer progenitor cells with multilineage differentiation potential. Deletion of the murine Gata2-77 enhancer, with a human equivalent that causes leukemia, downregulates the transcription factor GATA2 and blocks progenitor differentiation into erythrocytes, megakaryocytes, basophils, and granulocytes, but not macrophages. Using multiomics and single-cell analyses, we demonstrated that the enhancer orchestrates a balance between pro- and anti-fate circuitry in single cells. By increasing GATA2 expression, the enhancer instigates a fate-promoting mechanism while abrogating an innate immunity-linked, fate-suppressing mechanism. During embryogenesis, the suppressing mechanism dominated in enhancer mutant progenitors, thus yielding progenitors with a predominant monocytic differentiation potential. Coordinating fate-promoting and -suppressing circuits therefore averts deconstruction of a multifate system into a monopotent system and maintains critical progenitor heterogeneity and functionality.
© 2020 Johnson et al.
Conflict of interest statement
Disclosures: The authors declare no competing interests exist.
Figures
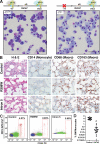
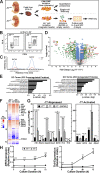
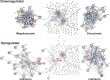
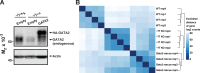
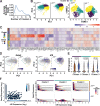
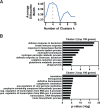
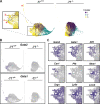
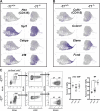
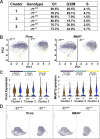
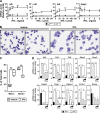
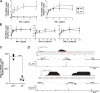
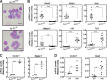
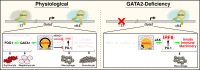
Similar articles
-
Pathogenic GATA2 genetic variants utilize an obligate enhancer mechanism to distort a multilineage differentiation program.Proc Natl Acad Sci U S A. 2024 Mar 5;121(10):e2317147121. doi: 10.1073/pnas.2317147121. Epub 2024 Feb 29. Proc Natl Acad Sci U S A. 2024. PMID: 38422019 Free PMC article.
-
Single-nucleotide human disease mutation inactivates a blood-regenerative GATA2 enhancer.J Clin Invest. 2019 Mar 1;129(3):1180-1192. doi: 10.1172/JCI122694. Epub 2019 Feb 11. J Clin Invest. 2019. PMID: 30620726 Free PMC article.
-
Integrating Enhancer Mechanisms to Establish a Hierarchical Blood Development Program.Cell Rep. 2017 Sep 19;20(12):2966-2979. doi: 10.1016/j.celrep.2017.08.090. Cell Rep. 2017. PMID: 28930689 Free PMC article.
-
Interferon regulatory factor-8-dependent innate immune alarm senses GATA2 deficiency to alter hematopoietic differentiation and function.Curr Opin Hematol. 2023 Jul 1;30(4):117-123. doi: 10.1097/MOH.0000000000000763. Epub 2023 Apr 27. Curr Opin Hematol. 2023. PMID: 37254854 Free PMC article. Review.
-
The role of the GATA2 transcription factor in normal and malignant hematopoiesis.Crit Rev Oncol Hematol. 2012 Apr;82(1):1-17. doi: 10.1016/j.critrevonc.2011.04.007. Epub 2011 May 24. Crit Rev Oncol Hematol. 2012. PMID: 21605981 Review.
Cited by
-
Linking GATA2 to myeloid dysplasia and complex cytogenetics in adult myelodysplastic neoplasm and acute myeloid leukemia.Blood Adv. 2024 Jan 9;8(1):80-92. doi: 10.1182/bloodadvances.2023011554. Blood Adv. 2024. PMID: 38029365 Free PMC article.
-
PRAM: a novel pooling approach for discovering intergenic transcripts from large-scale RNA sequencing experiments.Genome Res. 2020 Nov;30(11):1655-1666. doi: 10.1101/gr.252445.119. Epub 2020 Sep 21. Genome Res. 2020. PMID: 32958497 Free PMC article.
-
Pathogenic GATA2 genetic variants utilize an obligate enhancer mechanism to distort a multilineage differentiation program.Proc Natl Acad Sci U S A. 2024 Mar 5;121(10):e2317147121. doi: 10.1073/pnas.2317147121. Epub 2024 Feb 29. Proc Natl Acad Sci U S A. 2024. PMID: 38422019 Free PMC article.
-
GATA2 deficiency and MDS/AML: Experimental strategies for disease modelling and future therapeutic prospects.Br J Haematol. 2022 Nov;199(4):482-495. doi: 10.1111/bjh.18330. Epub 2022 Jun 26. Br J Haematol. 2022. PMID: 35753998 Free PMC article. Review.
-
Multidimensional profiling reveals GATA1-modulated stage-specific chromatin states and functional associations during human erythropoiesis.Nucleic Acids Res. 2023 Jul 21;51(13):6634-6653. doi: 10.1093/nar/gkad468. Nucleic Acids Res. 2023. PMID: 37254808 Free PMC article.
References
-
- Bigley V., Maisuria S., Cytlak U., Jardine L., Care M.A., Green K., Gunawan M., Milne P., Dickinson R., Wiscombe S., et al. . 2018. Biallelic interferon regulatory factor 8 mutation: A complex immunodeficiency syndrome with dendritic cell deficiency, monocytopenia, and immune dysregulation. J. Allergy Clin. Immunol. 141:2234–2248. 10.1016/j.jaci.2017.08.044 - DOI - PMC - PubMed
Publication types
MeSH terms
Substances
Grants and funding
LinkOut - more resources
Full Text Sources
Medical
Molecular Biology Databases