Global reference mapping of human transcription factor footprints
- PMID: 32728250
- PMCID: PMC7410829
- DOI: 10.1038/s41586-020-2528-x
Global reference mapping of human transcription factor footprints
Abstract
Combinatorial binding of transcription factors to regulatory DNA underpins gene regulation in all organisms. Genetic variation in regulatory regions has been connected with diseases and diverse phenotypic traits1, but it remains challenging to distinguish variants that affect regulatory function2. Genomic DNase I footprinting enables the quantitative, nucleotide-resolution delineation of sites of transcription factor occupancy within native chromatin3-6. However, only a small fraction of such sites have been precisely resolved on the human genome sequence6. Here, to enable comprehensive mapping of transcription factor footprints, we produced high-density DNase I cleavage maps from 243 human cell and tissue types and states and integrated these data to delineate about 4.5 million compact genomic elements that encode transcription factor occupancy at nucleotide resolution. We map the fine-scale structure within about 1.6 million DNase I-hypersensitive sites and show that the overwhelming majority are populated by well-spaced sites of single transcription factor-DNA interaction. Cell-context-dependent cis-regulation is chiefly executed by wholesale modulation of accessibility at regulatory DNA rather than by differential transcription factor occupancy within accessible elements. We also show that the enrichment of genetic variants associated with diseases or phenotypic traits in regulatory regions1,7 is almost entirely attributable to variants within footprints, and that functional variants that affect transcription factor occupancy are nearly evenly partitioned between loss- and gain-of-function alleles. Unexpectedly, we find increased density of human genetic variation within transcription factor footprints, revealing an unappreciated driver of cis-regulatory evolution. Our results provide a framework for both global and nucleotide-precision analyses of gene regulatory mechanisms and functional genetic variation.
Conflict of interest statement
The authors declare no competing interests.
Figures
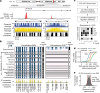
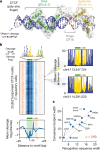
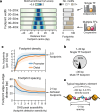
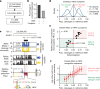
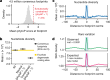
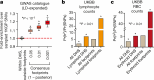
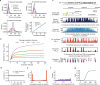
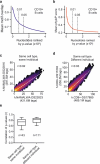
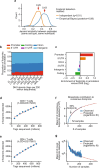
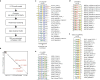
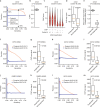
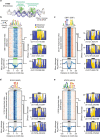
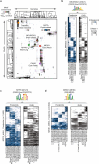
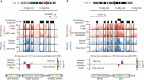
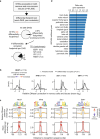
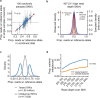
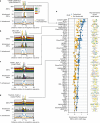
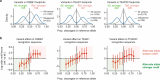
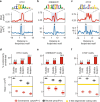
Similar articles
-
An expansive human regulatory lexicon encoded in transcription factor footprints.Nature. 2012 Sep 6;489(7414):83-90. doi: 10.1038/nature11212. Nature. 2012. PMID: 22955618 Free PMC article.
-
The accessible chromatin landscape of the human genome.Nature. 2012 Sep 6;489(7414):75-82. doi: 10.1038/nature11232. Nature. 2012. PMID: 22955617 Free PMC article.
-
Most brain disease-associated and eQTL haplotypes are not located within transcription factor DNase-seq footprints in brain.Hum Mol Genet. 2017 Jan 1;26(1):79-89. doi: 10.1093/hmg/ddw369. Hum Mol Genet. 2017. PMID: 27798116 Free PMC article.
-
Genomic footprinting.Nat Methods. 2016 Mar;13(3):213-21. doi: 10.1038/nmeth.3768. Nat Methods. 2016. PMID: 26914205 Review.
-
Exploring the function of genetic variants in the non-coding genomic regions: approaches for identifying human regulatory variants affecting gene expression.Brief Bioinform. 2015 May;16(3):393-412. doi: 10.1093/bib/bbu018. Epub 2014 Jun 10. Brief Bioinform. 2015. PMID: 24916300 Review.
Cited by
-
Denisovan introgression has shaped the immune system of present-day Papuans.PLoS Genet. 2022 Dec 8;18(12):e1010470. doi: 10.1371/journal.pgen.1010470. eCollection 2022 Dec. PLoS Genet. 2022. PMID: 36480515 Free PMC article.
-
Building integrative functional maps of gene regulation.Hum Mol Genet. 2022 Oct 20;31(R1):R114-R122. doi: 10.1093/hmg/ddac195. Hum Mol Genet. 2022. PMID: 36083269 Free PMC article.
-
Multiomic profiling of transcription factor binding and function in human brain.Nat Neurosci. 2024 Jul;27(7):1387-1399. doi: 10.1038/s41593-024-01658-8. Epub 2024 Jun 3. Nat Neurosci. 2024. PMID: 38831039
-
Predicting the impact of sequence motifs on gene regulation using single-cell data.Genome Biol. 2023 Aug 15;24(1):189. doi: 10.1186/s13059-023-03021-9. Genome Biol. 2023. PMID: 37582793 Free PMC article.
-
The ENCODE Uniform Analysis Pipelines.Res Sq [Preprint]. 2023 Jul 19:rs.3.rs-3111932. doi: 10.21203/rs.3.rs-3111932/v1. Res Sq. 2023. PMID: 37503119 Free PMC article. Preprint.
References
Publication types
MeSH terms
Substances
Grants and funding
LinkOut - more resources
Full Text Sources
Other Literature Sources