HAPPENN is a novel tool for hemolytic activity prediction for therapeutic peptides which employs neural networks
- PMID: 32616760
- PMCID: PMC7331684
- DOI: 10.1038/s41598-020-67701-3
HAPPENN is a novel tool for hemolytic activity prediction for therapeutic peptides which employs neural networks
Abstract
The growing prevalence of resistance to antibiotics motivates the search for new antibacterial agents. Antimicrobial peptides are a diverse class of well-studied membrane-active peptides which function as part of the innate host defence system, and form a promising avenue in antibiotic drug research. Some antimicrobial peptides exhibit toxicity against eukaryotic membranes, typically characterised by hemolytic activity assays, but currently, the understanding of what differentiates hemolytic and non-hemolytic peptides is limited. This study leverages advances in machine learning research to produce a novel artificial neural network classifier for the prediction of hemolytic activity from a peptide's primary sequence. The classifier achieves best-in-class performance, with cross-validated accuracy of [Formula: see text] and Matthews correlation coefficient of 0.71. This innovative classifier is available as a web server at https://research.timmons.eu/happenn , allowing the research community to utilise it for in silico screening of peptide drug candidates for high therapeutic efficacies.
Conflict of interest statement
The authors declare no competing interests.
Figures
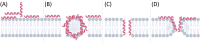
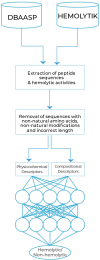
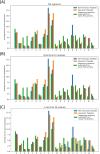
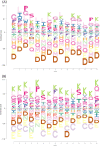
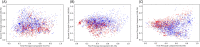
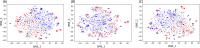
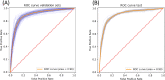
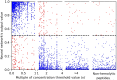
Similar articles
-
ENNAVIA is a novel method which employs neural networks for antiviral and anti-coronavirus activity prediction for therapeutic peptides.Brief Bioinform. 2021 Nov 5;22(6):bbab258. doi: 10.1093/bib/bbab258. Brief Bioinform. 2021. PMID: 34297817 Free PMC article.
-
HemoPred: a web server for predicting the hemolytic activity of peptides.Future Med Chem. 2017 Mar;9(3):275-291. doi: 10.4155/fmc-2016-0188. Epub 2017 Feb 17. Future Med Chem. 2017. PMID: 28211294
-
ENNAACT is a novel tool which employs neural networks for anticancer activity classification for therapeutic peptides.Biomed Pharmacother. 2021 Jan;133:111051. doi: 10.1016/j.biopha.2020.111051. Epub 2020 Nov 27. Biomed Pharmacother. 2021. PMID: 33254015
-
Machine learning-enabled discovery and design of membrane-active peptides.Bioorg Med Chem. 2018 Jun 1;26(10):2708-2718. doi: 10.1016/j.bmc.2017.07.012. Epub 2017 Jul 8. Bioorg Med Chem. 2018. PMID: 28728899 Free PMC article. Review.
-
The role of hydrophobic /hydrophilic balance in the activity of structurally flexible vs. rigid cytolytic polypeptides and analogs developed on their basis.Expert Rev Proteomics. 2018 Nov;15(11):873-886. doi: 10.1080/14789450.2018.1537786. Epub 2018 Oct 26. Expert Rev Proteomics. 2018. PMID: 30328726 Review.
Cited by
-
ENNAVIA is a novel method which employs neural networks for antiviral and anti-coronavirus activity prediction for therapeutic peptides.Brief Bioinform. 2021 Nov 5;22(6):bbab258. doi: 10.1093/bib/bbab258. Brief Bioinform. 2021. PMID: 34297817 Free PMC article.
-
Can large language models predict antimicrobial peptide activity and toxicity?RSC Med Chem. 2024 Apr 23;15(6):2030-2036. doi: 10.1039/d4md00159a. eCollection 2024 Jun 19. RSC Med Chem. 2024. PMID: 38911166 Free PMC article.
-
De Novo Design of AC-P19M, a Novel Anticancer Peptide with Apoptotic Effects on Lung Cancer Cells and Anti-Angiogenic Activity.Int J Mol Sci. 2022 Dec 9;23(24):15594. doi: 10.3390/ijms232415594. Int J Mol Sci. 2022. PMID: 36555235 Free PMC article.
-
Traditional and Computational Screening of Non-Toxic Peptides and Approaches to Improving Selectivity.Pharmaceuticals (Basel). 2022 Mar 8;15(3):323. doi: 10.3390/ph15030323. Pharmaceuticals (Basel). 2022. PMID: 35337121 Free PMC article. Review.
-
Improved Database Filtering Technology Enables More Efficient Ab Initio Design of Potent Peptides against Ebola Viruses.Pharmaceuticals (Basel). 2022 Apr 24;15(5):521. doi: 10.3390/ph15050521. Pharmaceuticals (Basel). 2022. PMID: 35631348 Free PMC article.
References
-
- Hultmark D. Drosophila immunity: paths and patterns. Curr. Opin. Immunol. 2003;15:12–19. - PubMed
-
- Yeaman MR, Yount NY. Mechanisms of antimicrobial peptide action and resistance. Pharmacol. Rev. 2003;55:27–55. - PubMed
-
- Vlieghe P, Lisowski V, Martinez J, Khrestchatisky M. Synthetic therapeutic peptides: science and market. Drug Discov. Today. 2010;15:40–56. - PubMed
Publication types
MeSH terms
Substances
LinkOut - more resources
Full Text Sources