An empirical Bayes approach to normalization and differential abundance testing for microbiome data
- PMID: 32493208
- PMCID: PMC7268703
- DOI: 10.1186/s12859-020-03552-z
An empirical Bayes approach to normalization and differential abundance testing for microbiome data
Abstract
Background: Advances in DNA sequencing have offered researchers an unprecedented opportunity to better study the variety of species living in and on the human body. However, the analysis of microbiome data is complicated by several challenges. First, the sequencing depth may vary by orders of magnitude across samples. Second, species are rare and the data often contain many zeros. Third, the specimen is a fraction of the microbial ecosystem, and so the data are compositional carrying only relative information. Other characteristics of microbiome data include pronounced over-dispersion in taxon abundances, and the existence of a phylogenetic tree that relates all bacterial species. To address some of these challenges, microbiome analysis workflows often normalize the read counts prior to downstream analysis. However, there are limitations in the current literature on the normalization of microbiome data.
Results: Under the multinomial distribution for the read counts and a prior for the unknown proportions, we propose an empirical Bayes approach to microbiome data normalization. Using a tree-based extension of the Dirichlet prior, we further extend our method by incorporating the phylogenetic tree into the normalization process. We study the impact of normalization on differential abundance analysis. In the presence of tree structure, we propose a phylogeny-aware detection procedure.
Conclusions: Extensive simulations and gut microbiome data applications are conducted to demonstrate the superior performance of our empirical Bayes method over other normalization methods, and over commonly-used methods for differential abundance testing. Original R scripts are available at GitHub (https://github.com/liudoubletian/eBay).
Keywords: Bayesian shrinkage; Differentially abundant OTUs; MetagenomeSeq; Phylogeny-aware analysis; Rarefying.
Conflict of interest statement
The authors declare that they have no competing interests.
Figures
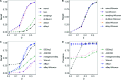
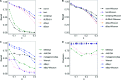
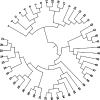
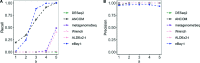
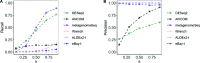
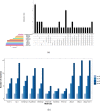
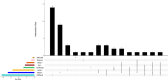
Similar articles
-
Transformation and differential abundance analysis of microbiome data incorporating phylogeny.Bioinformatics. 2021 Dec 11;37(24):4652-4660. doi: 10.1093/bioinformatics/btab543. Bioinformatics. 2021. PMID: 34302462
-
Normalization and microbial differential abundance strategies depend upon data characteristics.Microbiome. 2017 Mar 3;5(1):27. doi: 10.1186/s40168-017-0237-y. Microbiome. 2017. PMID: 28253908 Free PMC article.
-
phyloMDA: an R package for phylogeny-aware microbiome data analysis.BMC Bioinformatics. 2022 Jun 6;23(1):213. doi: 10.1186/s12859-022-04744-5. BMC Bioinformatics. 2022. PMID: 35668363 Free PMC article.
-
Analysis of microbial compositions: a review of normalization and differential abundance analysis.NPJ Biofilms Microbiomes. 2020 Dec 2;6(1):60. doi: 10.1038/s41522-020-00160-w. NPJ Biofilms Microbiomes. 2020. PMID: 33268781 Free PMC article. Review.
-
Correlation and association analyses in microbiome study integrating multiomics in health and disease.Prog Mol Biol Transl Sci. 2020;171:309-491. doi: 10.1016/bs.pmbts.2020.04.003. Epub 2020 May 23. Prog Mol Biol Transl Sci. 2020. PMID: 32475527 Review.
Cited by
-
Rusa deer microbiota: the importance of preliminary data analysis for meaningful diversity comparisons.Int Microbiol. 2024 Apr 8. doi: 10.1007/s10123-024-00521-x. Online ahead of print. Int Microbiol. 2024. PMID: 38589705
-
piCRISPR: Physically informed deep learning models for CRISPR/Cas9 off-target cleavage prediction.Artif Intell Life Sci. 2023 Dec;3:None. doi: 10.1016/j.ailsci.2023.100075. Artif Intell Life Sci. 2023. PMID: 38047242 Free PMC article.
-
Impact of Data and Study Characteristics on Microbiome Volatility Estimates.Genes (Basel). 2023 Jan 14;14(1):218. doi: 10.3390/genes14010218. Genes (Basel). 2023. PMID: 36672959 Free PMC article.
-
A maximum-type microbial differential abundance test with application to high-dimensional microbiome data analyses.Front Cell Infect Microbiol. 2022 Oct 28;12:988717. doi: 10.3389/fcimb.2022.988717. eCollection 2022. Front Cell Infect Microbiol. 2022. PMID: 36389165 Free PMC article.
-
Investigating differential abundance methods in microbiome data: A benchmark study.PLoS Comput Biol. 2022 Sep 8;18(9):e1010467. doi: 10.1371/journal.pcbi.1010467. eCollection 2022 Sep. PLoS Comput Biol. 2022. PMID: 36074761 Free PMC article. Review.
References
-
- Zhao L, Zhang F, Ding X, Wu G, Lam YY, Wang X, et al. Gut bacteria selectively promoted by dietary fibers alleviate type 2 diabetes. Science. 2018;359(6380):1151–6. - PubMed
-
- Spor A, Koren O, Ley R. Unravelling the effects of the environment and host genotype on the gut microbiome. Nat Rev Microbiol. 2011;9(4):279. - PubMed
-
- Rothschild D, Weissbrod O, Barkan E, Kurilshikov A, Korem T, Zeevi D, et al. Environment dominates over host genetics in shaping human gut microbiota. Nature. 2018;555(7695):210–15. - PubMed
MeSH terms
Grants and funding
LinkOut - more resources
Full Text Sources