The mutational constraint spectrum quantified from variation in 141,456 humans
- PMID: 32461654
- PMCID: PMC7334197
- DOI: 10.1038/s41586-020-2308-7
The mutational constraint spectrum quantified from variation in 141,456 humans
Erratum in
-
Author Correction: The mutational constraint spectrum quantified from variation in 141,456 humans.Nature. 2021 Feb;590(7846):E53. doi: 10.1038/s41586-020-03174-8. Nature. 2021. PMID: 33536625 Free PMC article. No abstract available.
-
Addendum: The mutational constraint spectrum quantified from variation in 141,456 humans.Nature. 2021 Sep;597(7874):E3-E4. doi: 10.1038/s41586-021-03758-y. Nature. 2021. PMID: 34373650 Free PMC article. No abstract available.
Abstract
Genetic variants that inactivate protein-coding genes are a powerful source of information about the phenotypic consequences of gene disruption: genes that are crucial for the function of an organism will be depleted of such variants in natural populations, whereas non-essential genes will tolerate their accumulation. However, predicted loss-of-function variants are enriched for annotation errors, and tend to be found at extremely low frequencies, so their analysis requires careful variant annotation and very large sample sizes1. Here we describe the aggregation of 125,748 exomes and 15,708 genomes from human sequencing studies into the Genome Aggregation Database (gnomAD). We identify 443,769 high-confidence predicted loss-of-function variants in this cohort after filtering for artefacts caused by sequencing and annotation errors. Using an improved model of human mutation rates, we classify human protein-coding genes along a spectrum that represents tolerance to inactivation, validate this classification using data from model organisms and engineered human cells, and show that it can be used to improve the power of gene discovery for both common and rare diseases.
Conflict of interest statement
K.J.K. owns stock in Personalis. R.K.W. has received unrestricted research grants from Takeda Pharmaceutical Company. A.H.O’D.-L. has received honoraria from ARUP and Chan Zuckerberg Initiative. E.V.M. has received research support in the form of charitable contributions from Charles River Laboratories and Ionis Pharmaceuticals, and has consulted for Deerfield Management. J.S.W. is a consultant for MyoKardia. B.M.N. is a member of the scientific advisory board at Deep Genomics and consultant for Camp4 Therapeutics, Takeda Pharmaceutical, and Biogen. M.J.D. is a founder of Maze Therapeutics. D.G.M. is a founder with equity in Goldfinch Bio, and has received research support from AbbVie, Astellas, Biogen, BioMarin, Eisai, Merck, Pfizer, and Sanofi-Genzyme. The views expressed in this article are those of the author(s) and not necessarily those of the NHS, the NIHR, or the Department of Health. M.I.M. has served on advisory panels for Pfizer, NovoNordisk, Zoe Global; has received honoraria from Merck, Pfizer, NovoNordisk and Eli Lilly; has stock options in Zoe Global and has received research funding from Abbvie, Astra Zeneca, Boehringer Ingelheim, Eli Lilly, Janssen, Merck, NovoNordisk, Pfizer, Roche, Sanofi Aventis, Servier & Takeda. As of June 2019, M.I.M. is an employee of Genentech, and holds stock in Roche. N.R. is a non-executive director of AstraZeneca.
Figures
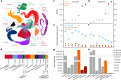
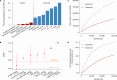
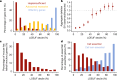
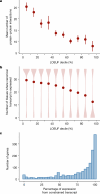
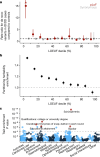
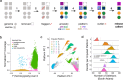
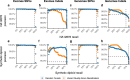
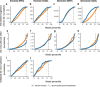
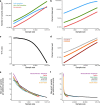
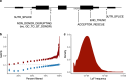
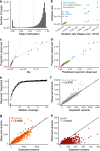
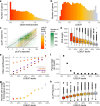
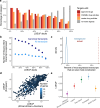
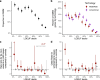
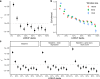
Comment in
-
Thousands of human sequences provide deep insight into single genomes.Nature. 2020 May;581(7809):385-386. doi: 10.1038/d41586-020-01485-4. Nature. 2020. PMID: 32461645 No abstract available.
-
Exploring human genomic diversity with gnomAD.Nat Rev Genet. 2020 Aug;21(8):448. doi: 10.1038/s41576-020-0255-7. Nat Rev Genet. 2020. PMID: 32488197 No abstract available.
Similar articles
-
Exome sequencing and analysis of 454,787 UK Biobank participants.Nature. 2021 Nov;599(7886):628-634. doi: 10.1038/s41586-021-04103-z. Epub 2021 Oct 18. Nature. 2021. PMID: 34662886 Free PMC article.
-
The UK10K project identifies rare variants in health and disease.Nature. 2015 Oct 1;526(7571):82-90. doi: 10.1038/nature14962. Epub 2015 Sep 14. Nature. 2015. PMID: 26367797 Free PMC article.
-
Molecular genetic studies of complex phenotypes.Transl Res. 2012 Feb;159(2):64-79. doi: 10.1016/j.trsl.2011.08.001. Epub 2011 Aug 31. Transl Res. 2012. PMID: 22243791 Free PMC article. Review.
-
Efficient and flexible Integration of variant characteristics in rare variant association studies using integrated nested Laplace approximation.PLoS Comput Biol. 2021 Feb 19;17(2):e1007784. doi: 10.1371/journal.pcbi.1007784. eCollection 2021 Feb. PLoS Comput Biol. 2021. PMID: 33606672 Free PMC article.
-
Diagnosing rare diseases after the exome.Cold Spring Harb Mol Case Stud. 2018 Dec 17;4(6):a003392. doi: 10.1101/mcs.a003392. Print 2018 Dec. Cold Spring Harb Mol Case Stud. 2018. PMID: 30559314 Free PMC article. Review.
Cited by
-
Cardiomyopathies in 100,000 genomes project: interval evaluation improves diagnostic yield and informs strategies for ongoing gene discovery.Genome Med. 2024 Oct 29;16(1):125. doi: 10.1186/s13073-024-01390-9. Genome Med. 2024. PMID: 39472908 Free PMC article.
-
Understanding the heterogeneous performance of variant effect predictors across human protein-coding genes.Sci Rep. 2024 Oct 30;14(1):26114. doi: 10.1038/s41598-024-76202-6. Sci Rep. 2024. PMID: 39478110 Free PMC article.
-
BCL11A intellectual developmental disorder: defining the clinical spectrum and genotype-phenotype correlations.Eur J Hum Genet. 2024 Oct 24. doi: 10.1038/s41431-024-01701-z. Online ahead of print. Eur J Hum Genet. 2024. PMID: 39448799
-
Whole genome variation in 27 Mexican indigenous populations, demographic and biomedical insights.PLoS One. 2021 Apr 8;16(4):e0249773. doi: 10.1371/journal.pone.0249773. eCollection 2021. PLoS One. 2021. PMID: 33831079 Free PMC article. Clinical Trial.
-
Whole-genome sequencing identifies new candidate genes for nonobstructive azoospermia.Andrology. 2022 Nov;10(8):1605-1624. doi: 10.1111/andr.13269. Epub 2022 Sep 7. Andrology. 2022. PMID: 36017582 Free PMC article.
References
-
- Schneeberger, K. Using next-generation sequencing to isolate mutant genes from forward genetic screens. Nat. Rev. Genet. 15, 662–676 (2014). - PubMed
-
- Zambrowicz, B. P. & Sands, A. T. Knockouts model the 100 best-selling drugs—will they model the next 100? Nat. Rev. Drug Discov. 2, 38–51 (2003). - PubMed
Publication types
MeSH terms
Substances
Grants and funding
- R01 MH115957/MH/NIMH NIH HHS/United States
- CS/14/2/30841/BHF_/British Heart Foundation/United Kingdom
- UL1 TR001863/TR/NCATS NIH HHS/United States
- MC_UP_1102/20/MRC_/Medical Research Council/United Kingdom
- R01 HD081256/HD/NICHD NIH HHS/United States
- R01 MH085548/MH/NIMH NIH HHS/United States
- R56 MH115957/MH/NIMH NIH HHS/United States
- R01 GM104371/GM/NIGMS NIH HHS/United States
- HD081256/HD/NICHD NIH HHS/United States
- UM1 HG008900/HG/NHGRI NIH HHS/United States
- RG/18/10/33842/BHF_/British Heart Foundation/United Kingdom
- T32 HG002295/HG/NHGRI NIH HHS/United States
- MH115957/MH/NIMH NIH HHS/United States
- U54 DK105566/DK/NIDDK NIH HHS/United States
- UM1 HG006493/HG/NHGRI NIH HHS/United States
- U24 HG008956/HG/NHGRI NIH HHS/United States
- F32 GM115208/GM/NIGMS NIH HHS/United States
LinkOut - more resources
Full Text Sources
Other Literature Sources
Miscellaneous