Deep learning approach for quantification of organelles and misfolded polypeptide delivery within degradative compartments
- PMID: 32401604
- PMCID: PMC7359569
- DOI: 10.1091/mbc.E20-04-0269
Deep learning approach for quantification of organelles and misfolded polypeptide delivery within degradative compartments
Abstract
Endolysosomal compartments maintain cellular fitness by clearing dysfunctional organelles and proteins from cells. Modulation of their activity offers therapeutic opportunities. Quantification of cargo delivery to and/or accumulation within endolysosomes is instrumental for characterizing lysosome-driven pathways at the molecular level and monitoring consequences of genetic or environmental modifications. Here we introduce LysoQuant, a deep learning approach for segmentation and classification of fluorescence images capturing cargo delivery within endolysosomes for clearance. LysoQuant is trained for unbiased and rapid recognition with human-level accuracy, and the pipeline informs on a series of quantitative parameters such as endolysosome number, size, shape, position within cells, and occupancy, which report on activity of lysosome-driven pathways. In our selected examples, LysoQuant successfully determines the magnitude of mechanistically distinct catabolic pathways that ensure lysosomal clearance of a model organelle, the endoplasmic reticulum, and of a model protein, polymerogenic ATZ. It does so with accuracy and velocity compatible with those of high-throughput analyses.
Figures
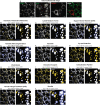
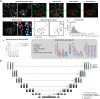
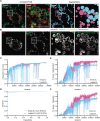
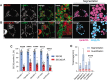
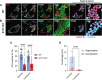
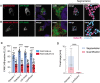
Similar articles
-
Quantitative and time-resolved monitoring of organelle and protein delivery to the lysosome with a tandem fluorescent Halo-GFP reporter.Mol Biol Cell. 2022 May 15;33(6):ar57. doi: 10.1091/mbc.E21-10-0526. Epub 2022 Feb 2. Mol Biol Cell. 2022. PMID: 35108065 Free PMC article.
-
Proteasomal and lysosomal clearance of faulty secretory proteins: ER-associated degradation (ERAD) and ER-to-lysosome-associated degradation (ERLAD) pathways.Crit Rev Biochem Mol Biol. 2019 Apr;54(2):153-163. doi: 10.1080/10409238.2019.1610351. Epub 2019 May 14. Crit Rev Biochem Mol Biol. 2019. PMID: 31084437 Review.
-
Role of endolysosomes and inter-organellar signaling in brain disease.Neurobiol Dis. 2020 Feb;134:104670. doi: 10.1016/j.nbd.2019.104670. Epub 2019 Nov 9. Neurobiol Dis. 2020. PMID: 31707116 Free PMC article. Review.
-
SNX19 restricts endolysosome motility through contacts with the endoplasmic reticulum.Nat Commun. 2021 Jul 27;12(1):4552. doi: 10.1038/s41467-021-24709-1. Nat Commun. 2021. PMID: 34315878 Free PMC article.
-
Transcriptional changes of mouse splenocyte organelle components following acute infection with Toxoplasma gondii.Exp Parasitol. 2016 Aug;167:7-16. doi: 10.1016/j.exppara.2016.04.019. Epub 2016 Apr 27. Exp Parasitol. 2016. PMID: 27132051
Cited by
-
Validation of a highly sensitive HaloTag-based assay to evaluate the potency of a novel class of allosteric β-Galactosidase correctors.PLoS One. 2023 Nov 29;18(11):e0294437. doi: 10.1371/journal.pone.0294437. eCollection 2023. PLoS One. 2023. PMID: 38019733 Free PMC article.
-
N-glycan processing selects ERAD-resistant misfolded proteins for ER-to-lysosome-associated degradation.EMBO J. 2021 Aug 2;40(15):e107240. doi: 10.15252/embj.2020107240. Epub 2021 Jun 21. EMBO J. 2021. PMID: 34152647 Free PMC article.
-
Guidelines for the use and interpretation of assays for monitoring autophagy (4th edition)1.Autophagy. 2021 Jan;17(1):1-382. doi: 10.1080/15548627.2020.1797280. Epub 2021 Feb 8. Autophagy. 2021. PMID: 33634751 Free PMC article.
-
Quantitative and time-resolved monitoring of organelle and protein delivery to the lysosome with a tandem fluorescent Halo-GFP reporter.Mol Biol Cell. 2022 May 15;33(6):ar57. doi: 10.1091/mbc.E21-10-0526. Epub 2022 Feb 2. Mol Biol Cell. 2022. PMID: 35108065 Free PMC article.
-
ER-to-lysosome-associated degradation acts as failsafe mechanism upon ERAD dysfunction.EMBO Rep. 2024 Jun;25(6):2773-2785. doi: 10.1038/s44319-024-00165-y. Epub 2024 May 21. EMBO Rep. 2024. PMID: 38773321 Free PMC article.
References
-
- Arganda-Carreras I, Kaynig V, Rueden C, Eliceiri KW, Schindelin J, Cardona A, Sebastian Seung H. (2017). Trainable Weka segmentation: a machine learning tool for microscopy pixel classification. Bioinformatics , 2424–2426. - PubMed
-
- Atherton TJ, Kerbyson DJ. (1999). Size invariant circle detection. Image Vision Comput , 795–803.
-
- Ballabio A, Bonifacino JS. (2019). Lysosomes as dynamic regulators of cell and organismal homeostasis. Nat Rev Mol Cell Biol. - PubMed
-
- Berg S, Kutra D, Kroeger T, Straehle CN, Kausler BX, Haubold C, Schiegg M, Ales J, Beier T, Rudy M, et al. (2019). iLastik: interactive machine learning for (bio)image analysis. Nat Methods , 1226–1232. - PubMed
Publication types
MeSH terms
Substances
LinkOut - more resources
Full Text Sources