Automated glioma grading on conventional MRI images using deep convolutional neural networks
- PMID: 32277478
- PMCID: PMC8494136
- DOI: 10.1002/mp.14168
Automated glioma grading on conventional MRI images using deep convolutional neural networks
Erratum in
-
Erratum: "Automated glioma grading on conventional MRI images using deep convolutional neural networks".Med Phys. 2023 Sep;50(9):5930-5931. doi: 10.1002/mp.16588. Med Phys. 2023. PMID: 37689089 No abstract available.
Abstract
Purpose: Gliomas are the most common primary tumor of the brain and are classified into grades I-IV of the World Health Organization (WHO), based on their invasively histological appearance. Gliomas grading plays an important role to determine the treatment plan and prognosis prediction. In this study we propose two novel methods for automatic, non-invasively distinguishing low-grade (Grades II and III) glioma (LGG) and high-grade (grade IV) glioma (HGG) on conventional MRI images by using deep convolutional neural networks (CNNs).
Methods: All MRI images have been preprocessed first by rigid image registration and intensity inhomogeneity correction. Both proposed methods consist of two steps: (a) three-dimensional (3D) brain tumor segmentation based on a modification of the popular U-Net model; (b) tumor classification on segmented brain tumor. In the first method, the slice with largest area of tumor is determined and the state-of-the-art mask R-CNN model is employed for tumor grading. To improve the performance of the grading model, a two-dimensional (2D) data augmentation has been implemented to increase both the amount and the diversity of the training images. In the second method, denoted as 3DConvNet, a 3D volumetric CNNs is applied directly on bounding image regions of segmented tumor for classification, which can fully leverage the 3D spatial contextual information of volumetric image data.
Results: The proposed schemes were evaluated on The Cancer Imaging Archive (TCIA) low grade glioma (LGG) data, and the Multimodal Brain Tumor Image Segmentation (BraTS) Benchmark 2018 training datasets with fivefold cross validation. All data are divided into training, validation, and test sets. Based on biopsy-proven ground truth, the performance metrics of sensitivity, specificity, and accuracy are measured on the test sets. The results are 0.935 (sensitivity), 0.972 (specificity), and 0.963 (accuracy) for the 2D Mask R-CNN based method, and 0.947 (sensitivity), 0.968 (specificity), and 0.971 (accuracy) for the 3DConvNet method, respectively. In regard to efficiency, for 3D brain tumor segmentation, the program takes around ten and a half hours for training with 300 epochs on BraTS 2018 dataset and takes only around 50 s for testing of a typical image with a size of 160 × 216 × 176. For 2D Mask R-CNN based tumor grading, the program takes around 4 h for training with around 60 000 iterations, and around 1 s for testing of a 2D slice image with size of 128 × 128. For 3DConvNet based tumor grading, the program takes around 2 h for training with 10 000 iterations, and 0.25 s for testing of a 3D cropped image with size of 64 × 64 × 64, using a DELL PRECISION Tower T7910, with two NVIDIA Titan Xp GPUs.
Conclusions: Two effective glioma grading methods on conventional MRI images using deep convolutional neural networks have been developed. Our methods are fully automated without manual specification of region-of-interests and selection of slices for model training, which are common in traditional machine learning based brain tumor grading methods. This methodology may play a crucial role in selecting effective treatment options and survival predictions without the need for surgical biopsy.
Keywords: Glioma grading; MRI image; brain tumor; convolutional neural networks; deep learning.
© 2020 American Association of Physicists in Medicine.
Figures
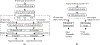
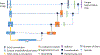
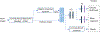
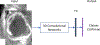
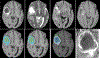
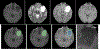
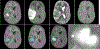
Similar articles
-
Brain tumor segmentation using holistically nested neural networks in MRI images.Med Phys. 2017 Oct;44(10):5234-5243. doi: 10.1002/mp.12481. Epub 2017 Aug 20. Med Phys. 2017. PMID: 28736864 Free PMC article.
-
Deep Multi-Scale 3D Convolutional Neural Network (CNN) for MRI Gliomas Brain Tumor Classification.J Digit Imaging. 2020 Aug;33(4):903-915. doi: 10.1007/s10278-020-00347-9. J Digit Imaging. 2020. PMID: 32440926 Free PMC article.
-
Brain tumor segmentation and grading of lower-grade glioma using deep learning in MRI images.Comput Biol Med. 2020 Jun;121:103758. doi: 10.1016/j.compbiomed.2020.103758. Epub 2020 Apr 22. Comput Biol Med. 2020. PMID: 32568668
-
Analyzing magnetic resonance imaging data from glioma patients using deep learning.Comput Med Imaging Graph. 2021 Mar;88:101828. doi: 10.1016/j.compmedimag.2020.101828. Epub 2020 Dec 2. Comput Med Imaging Graph. 2021. PMID: 33571780 Free PMC article. Review.
-
A state-of-the-art technique to perform cloud-based semantic segmentation using deep learning 3D U-Net architecture.BMC Bioinformatics. 2022 Jun 24;23(1):251. doi: 10.1186/s12859-022-04794-9. BMC Bioinformatics. 2022. PMID: 35751030 Free PMC article. Review.
Cited by
-
Brain tumor grade classification using the ConvNext architecture.Digit Health. 2024 Sep 28;10:20552076241284920. doi: 10.1177/20552076241284920. eCollection 2024 Jan-Dec. Digit Health. 2024. PMID: 39372816 Free PMC article.
-
A computer-aided diagnosis system for brain tumors based on artificial intelligence algorithms.Front Neurosci. 2023 Jul 7;17:1120781. doi: 10.3389/fnins.2023.1120781. eCollection 2023. Front Neurosci. 2023. PMID: 37483342 Free PMC article.
-
Detection and Grading of Gliomas Using a Novel Two-Phase Machine Learning Method Based on MRI Images.Front Neurosci. 2021 May 14;15:650629. doi: 10.3389/fnins.2021.650629. eCollection 2021. Front Neurosci. 2021. PMID: 34054411 Free PMC article.
-
Segmentation Algorithm of Magnetic Resonance Imaging Glioma under Fully Convolutional Densely Connected Convolutional Networks.Stem Cells Int. 2022 Oct 17;2022:8619690. doi: 10.1155/2022/8619690. eCollection 2022. Stem Cells Int. 2022. Retraction in: Stem Cells Int. 2023 Sep 14;2023:9842197. doi: 10.1155/2023/9842197. PMID: 36299467 Free PMC article. Retracted.
-
Magnetic Fields and Cancer: Epidemiology, Cellular Biology, and Theranostics.Int J Mol Sci. 2022 Jan 25;23(3):1339. doi: 10.3390/ijms23031339. Int J Mol Sci. 2022. PMID: 35163262 Free PMC article. Review.
References
-
- Louis DN, Perry A, Reifenberger G, et al. The 2016 world health organization classification of tumors of the central nervous system: a summary. Acta Neuropathol. 2016;131:803–820. - PubMed
-
- DeAngelis LM. Brain tumors. N Engl J Med. 2001;344:114–123. - PubMed
-
- Caulo M, Panara V, Tortora D, et al. Data-driven grading of brain gliomas: a multiparametric MR imaging study. Radiology. 2014;272:494–503. - PubMed
MeSH terms
Grants and funding
LinkOut - more resources
Full Text Sources
Other Literature Sources
Medical