Predicting Antibody Developability Profiles Through Early Stage Discovery Screening
- PMID: 32249670
- PMCID: PMC7153844
- DOI: 10.1080/19420862.2020.1743053
Predicting Antibody Developability Profiles Through Early Stage Discovery Screening
Abstract
Monoclonal antibodies play an increasingly important role for the development of new drugs across multiple therapy areas. The term 'developability' encompasses the feasibility of molecules to successfully progress from discovery to development via evaluation of their physicochemical properties. These properties include the tendency for self-interaction and aggregation, thermal stability, colloidal stability, and optimization of their properties through sequence engineering. Selection of the best antibody molecule based on biological function, efficacy, safety, and developability allows for a streamlined and successful CMC phase. An efficient and practical high-throughput developability workflow (100 s-1,000 s of molecules) implemented during early antibody generation and screening is crucial to select the best lead candidates. This involves careful assessment of critical developability parameters, combined with binding affinity and biological properties evaluation using small amounts of purified material (<1 mg), as well as an efficient data management and database system. Herein, a panel of 152 various human or humanized monoclonal antibodies was analyzed in biophysical property assays. Correlations between assays for different sets of properties were established. We demonstrated in two case studies that physicochemical properties and key assay endpoints correlate with key downstream process parameters. The workflow allows the elimination of antibodies with suboptimal properties and a rank ordering of molecules for further evaluation early in the candidate selection process. This enables any further engineering for problematic sequence attributes without affecting program timelines.
Keywords: CMC; Monoclonal antibodies; antibody discovery; antibody screening; biophysical properties; developability; manufacturability; protein analytics; protein engineering.
Figures
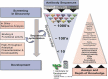
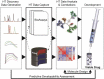
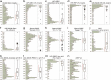
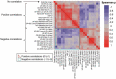
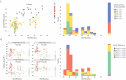
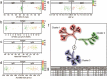
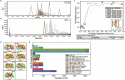
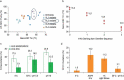
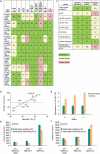
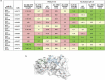
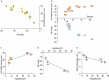
Similar articles
-
Current advances in biopharmaceutical informatics: guidelines, impact and challenges in the computational developability assessment of antibody therapeutics.MAbs. 2022 Jan-Dec;14(1):2020082. doi: 10.1080/19420862.2021.2020082. MAbs. 2022. PMID: 35104168 Free PMC article. Review.
-
Structure, heterogeneity and developability assessment of therapeutic antibodies.MAbs. 2019 Feb/Mar;11(2):239-264. doi: 10.1080/19420862.2018.1553476. Epub 2018 Dec 17. MAbs. 2019. PMID: 30543482 Free PMC article. Review.
-
In vitro and in silico assessment of the developability of a designed monoclonal antibody library.MAbs. 2019 Feb/Mar;11(2):388-400. doi: 10.1080/19420862.2018.1556082. Epub 2019 Jan 18. MAbs. 2019. PMID: 30523762 Free PMC article.
-
Approaches to expand the conventional toolbox for discovery and selection of antibodies with drug-like physicochemical properties.MAbs. 2023 Jan-Dec;15(1):2164459. doi: 10.1080/19420862.2022.2164459. MAbs. 2023. PMID: 36629855 Free PMC article.
-
Blueprint for antibody biologics developability.MAbs. 2023 Jan-Dec;15(1):2185924. doi: 10.1080/19420862.2023.2185924. MAbs. 2023. PMID: 36880643 Free PMC article. Review.
Cited by
-
Accelerating therapeutic protein design with computational approaches toward the clinical stage.Comput Struct Biotechnol J. 2023 Apr 29;21:2909-2926. doi: 10.1016/j.csbj.2023.04.027. eCollection 2023. Comput Struct Biotechnol J. 2023. PMID: 38213894 Free PMC article. Review.
-
Analytical Workflows to Unlock Predictive Power in Biotherapeutic Developability.Pharm Res. 2023 Feb;40(2):487-500. doi: 10.1007/s11095-022-03448-y. Epub 2022 Dec 5. Pharm Res. 2023. PMID: 36471025 Free PMC article.
-
Crystal Structure and Characterization of Human Heavy-Chain Only Antibodies Reveals a Novel, Stable Dimeric Structure Similar to Monoclonal Antibodies.Antibodies (Basel). 2020 Nov 22;9(4):66. doi: 10.3390/antib9040066. Antibodies (Basel). 2020. PMID: 33266498 Free PMC article.
-
Fast-tracking antibody maturation using a B cell-based display system.MAbs. 2022 Jan-Dec;14(1):2122275. doi: 10.1080/19420862.2022.2122275. MAbs. 2022. PMID: 36202784 Free PMC article.
-
Current advances in biopharmaceutical informatics: guidelines, impact and challenges in the computational developability assessment of antibody therapeutics.MAbs. 2022 Jan-Dec;14(1):2020082. doi: 10.1080/19420862.2021.2020082. MAbs. 2022. PMID: 35104168 Free PMC article. Review.
References
MeSH terms
Substances
LinkOut - more resources
Full Text Sources
Other Literature Sources