Epidemics with mutating infectivity on small-world networks
- PMID: 32246023
- PMCID: PMC7125191
- DOI: 10.1038/s41598-020-62597-5
Epidemics with mutating infectivity on small-world networks
Abstract
Epidemics and evolution of many pathogens occur on similar timescales so that their dynamics are often entangled. Here, in a first step to study this problem theoretically, we analyze mutating pathogens spreading on simple SIR networks with grid-like connectivity. We have in mind the spatial aspect of epidemics, which often advance on transport links between hosts or groups of hosts such as cities or countries. We focus on the case of mutations that enhance an agent's infection rate. We uncover that the small-world property, i.e., the presence of long-range connections, makes the network very vulnerable, supporting frequent supercritical mutations and bringing the network from disease extinction to full blown epidemic. For very large numbers of long-range links, however, the effect reverses and we find a reduced chance for large outbreaks. We study two cases, one with discrete number of mutational steps and one with a continuous genetic variable, and we analyze various scaling regimes. For the continuous case we derive a Fokker-Planck-like equation for the probability density and solve it for small numbers of shortcuts using the WKB approximation. Our analysis supports the claims that a potentiating mutation in the transmissibility might occur during an epidemic wave and not necessarily before its initiation.
Conflict of interest statement
The authors declare no competing interests.
Figures
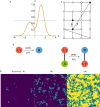
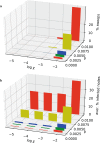
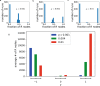
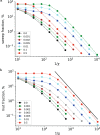
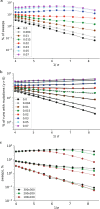
Similar articles
-
Epidemic spreading on interconnected networks.Phys Rev E Stat Nonlin Soft Matter Phys. 2012 Aug;86(2 Pt 2):026106. doi: 10.1103/PhysRevE.86.026106. Epub 2012 Aug 10. Phys Rev E Stat Nonlin Soft Matter Phys. 2012. PMID: 23005824
-
Epidemic outbreaks in two-scale community networks.Phys Rev E Stat Nonlin Soft Matter Phys. 2014 Jul;90(1):012810. doi: 10.1103/PhysRevE.90.012810. Epub 2014 Jul 21. Phys Rev E Stat Nonlin Soft Matter Phys. 2014. PMID: 25122345
-
Modelling development of epidemics with dynamic small-world networks.J Theor Biol. 2005 Jun 7;234(3):413-21. doi: 10.1016/j.jtbi.2004.12.003. Epub 2005 Jan 20. J Theor Biol. 2005. PMID: 15784275
-
Epidemic fronts in complex networks with metapopulation structure.Phys Rev E Stat Nonlin Soft Matter Phys. 2013 Jul;88(1):012809. doi: 10.1103/PhysRevE.88.012809. Epub 2013 Jul 15. Phys Rev E Stat Nonlin Soft Matter Phys. 2013. PMID: 23944520
-
Modeling epidemics: A primer and Numerus Model Builder implementation.Epidemics. 2018 Dec;25:9-19. doi: 10.1016/j.epidem.2018.06.001. Epub 2018 Jul 13. Epidemics. 2018. PMID: 30017895 Review.
Cited by
-
Small-world effects in a modified epidemiological model with mutation and permanent immune mechanism.Nonlinear Dyn. 2021;106(2):1557-1572. doi: 10.1007/s11071-021-06519-8. Epub 2021 May 11. Nonlinear Dyn. 2021. PMID: 33994664 Free PMC article.
-
COVID-19 related knowledge sharing practice and associated factors among healthcare providers worked in COVID-19 treatment centers at teaching hospitals in Northwest Ethiopia: A cross-sectional study.Inform Med Unlocked. 2022;28:100856. doi: 10.1016/j.imu.2022.100856. Epub 2022 Jan 15. Inform Med Unlocked. 2022. PMID: 35071731 Free PMC article.
-
Epidemic spreading under mutually independent intra- and inter-host pathogen evolution.Nat Commun. 2022 Oct 20;13(1):6218. doi: 10.1038/s41467-022-34027-9. Nat Commun. 2022. PMID: 36266285 Free PMC article.
-
Dynamic tracking with model-based forecasting for the spread of the COVID-19 pandemic.Chaos Solitons Fractals. 2020 Oct;139:110298. doi: 10.1016/j.chaos.2020.110298. Epub 2020 Sep 18. Chaos Solitons Fractals. 2020. PMID: 32982084 Free PMC article.
-
Assessment of the Burden of SARS-CoV-2 Variants of Concern Among Essential Workers in the Greater Toronto Area, Canada.JAMA Netw Open. 2021 Oct 1;4(10):e2130284. doi: 10.1001/jamanetworkopen.2021.30284. JAMA Netw Open. 2021. PMID: 34665241 Free PMC article.
References
-
- WHO: Ebola situation report 30 March 2016 (2016).