Heterogeneous Multi-Layered Network Model for Omics Data Integration and Analysis
- PMID: 32063919
- PMCID: PMC6997577
- DOI: 10.3389/fgene.2019.01381
Heterogeneous Multi-Layered Network Model for Omics Data Integration and Analysis
Abstract
Advances in next-generation sequencing and high-throughput techniques have enabled the generation of vast amounts of diverse omics data. These big data provide an unprecedented opportunity in biology, but impose great challenges in data integration, data mining, and knowledge discovery due to the complexity, heterogeneity, dynamics, uncertainty, and high-dimensionality inherited in the omics data. Network has been widely used to represent relations between entities in biological system, such as protein-protein interaction, gene regulation, and brain connectivity (i.e. network construction) as well as to infer novel relations given a reconstructed network (aka link prediction). Particularly, heterogeneous multi-layered network (HMLN) has proven successful in integrating diverse biological data for the representation of the hierarchy of biological system. The HMLN provides unparalleled opportunities but imposes new computational challenges on establishing causal genotype-phenotype associations and understanding environmental impact on organisms. In this review, we focus on the recent advances in developing novel computational methods for the inference of novel biological relations from the HMLN. We first discuss the properties of biological HMLN. Then we survey four categories of state-of-the-art methods (matrix factorization, random walk, knowledge graph, and deep learning). Thirdly, we demonstrate their applications to omics data integration and analysis. Finally, we outline strategies for future directions in the development of new HMLN models.
Keywords: biological data analysis; biological network; data mining and knowledge discovery; deep learning; link prediction; machine learning; relation inference.
Copyright © 2020 Lee, Zhang, Poleksic and Xie.
Figures
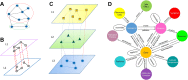
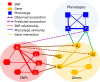
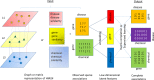
Similar articles
-
Integrate multi-omics data with biological interaction networks using Multi-view Factorization AutoEncoder (MAE).BMC Genomics. 2019 Dec 20;20(Suppl 11):944. doi: 10.1186/s12864-019-6285-x. BMC Genomics. 2019. PMID: 31856727 Free PMC article.
-
Multi-omics integration in biomedical research - A metabolomics-centric review.Anal Chim Acta. 2021 Jan 2;1141:144-162. doi: 10.1016/j.aca.2020.10.038. Epub 2020 Oct 22. Anal Chim Acta. 2021. PMID: 33248648 Free PMC article. Review.
-
A cross-level information transmission network for hierarchical omics data integration and phenotype prediction from a new genotype.Bioinformatics. 2021 Dec 22;38(1):204-210. doi: 10.1093/bioinformatics/btab580. Bioinformatics. 2021. PMID: 34390577 Free PMC article.
-
Machine learning: its challenges and opportunities in plant system biology.Appl Microbiol Biotechnol. 2022 May;106(9-10):3507-3530. doi: 10.1007/s00253-022-11963-6. Epub 2022 May 16. Appl Microbiol Biotechnol. 2022. PMID: 35575915 Review.
-
Transforming Clinical Research: The Power of High-Throughput Omics Integration.Proteomes. 2024 Sep 6;12(3):25. doi: 10.3390/proteomes12030025. Proteomes. 2024. PMID: 39311198 Free PMC article. Review.
Cited by
-
A multimodal graph neural network framework for cancer molecular subtype classification.BMC Bioinformatics. 2024 Jan 15;25(1):27. doi: 10.1186/s12859-023-05622-4. BMC Bioinformatics. 2024. PMID: 38225583 Free PMC article.
-
Omics in a Digital World: The Role of Bioinformatics in Providing New Insights Into Human Aging.Front Genet. 2021 Jun 10;12:689824. doi: 10.3389/fgene.2021.689824. eCollection 2021. Front Genet. 2021. PMID: 34178042 Free PMC article. Review.
-
Data-Driven Modeling of Pregnancy-Related Complications.Trends Mol Med. 2021 Aug;27(8):762-776. doi: 10.1016/j.molmed.2021.01.007. Epub 2021 Feb 8. Trends Mol Med. 2021. PMID: 33573911 Free PMC article. Review.
-
MARML: Motif-Aware Deep Representation Learning in Multilayer Networks.IEEE Trans Neural Netw Learn Syst. 2024 Sep;35(9):11649-11660. doi: 10.1109/TNNLS.2023.3341347. Epub 2024 Sep 3. IEEE Trans Neural Netw Learn Syst. 2024. PMID: 38113155
-
Identifying ceRNA Networks Associated With the Susceptibility and Persistence of Atrial Fibrillation Through Weighted Gene Co-Expression Network Analysis.Front Genet. 2021 Jun 23;12:653474. doi: 10.3389/fgene.2021.653474. eCollection 2021. Front Genet. 2021. PMID: 34249084 Free PMC article.
References
-
- Batmaz Z., Yurekli A., Bilge A., Kaleli C. (2019). A review on deep learning for recommender systems: challenges and remedies. Artif. Intell. Rev. 52, 1–37. 10.1007/s10462-018-9654-y - DOI
-
- Battaglia P. W., Hamrick J. B., Bapst V., Sanchez-Gonzalez A., Zambaldi V., Malinowski M., et al. (2018). Relational inductive biases, deep learning, and graph networks. arXiv preprint arXiv:1806.01261.
-
- Breese J. S., Heckerman D., Kadie C. (1998). “Empirical analysis of predictive algorithms for collaborative filtering” in Proceedings of the Fourteenth conference on Uncertainty in artificial intelligence (Morgan Kaufmann Publishers Inc.: Burlington, MA: ), 43–52.
-
- Cai L., Wang W. Y. (2017). Kbgan: Adversarial learning for knowledge graph embeddings. arXiv preprint arXiv:1711.04071.
Publication types
Grants and funding
LinkOut - more resources
Full Text Sources