Locally optimized correlation-guided Bayesian adaptive regularization for ultrasound strain imaging
- PMID: 32028272
- PMCID: PMC7682728
- DOI: 10.1088/1361-6560/ab735f
Locally optimized correlation-guided Bayesian adaptive regularization for ultrasound strain imaging
Abstract
Ultrasound strain imaging utilizes radio-frequency (RF) ultrasound echo signals to estimate the relative elasticity of tissue under deformation. Due to the diagnostic value inherent in tissue elasticity, ultrasound strain imaging has found widespread clinical and preclinical applications. Accurate displacement estimation using pre and post-deformation RF signals is a crucial first step to derive high quality strain tensor images. Incorporating regularization into the displacement estimation framework is a commonly employed strategy to improve estimation accuracy and precision. In this work, we propose an adaptive variation of the iterative Bayesian regularization scheme utilizing RF similarity metric signal-to-noise ratio previously proposed by our group. The regularization scheme is incorporated into a 2D multi-level block matching (BM) algorithm for motion estimation. Adaptive nature of our algorithm is attributed to the dynamic variation of iteration number based on the normalized cross-correlation (NCC) function quality and a similarity measure between pre-deformation and motion compensated post-deformation RF signals. The proposed method is validated for either quasi-static and cardiac elastography or strain imaging applications using uniform and inclusion phantoms and canine cardiac deformation simulation models. Performance of adaptive Bayesian regularization was compared to conventional NCC and Bayesian regularization with fixed number of iterations. Results from uniform phantom simulation study show significant improvement in lateral displacement and strain estimation accuracy. For instance, at 1.5% lateral strain in a uniform phantom, Bayesian regularization with five iterations incurred a lateral strain error of 104.49%, which was significantly reduced using our adaptive approach to 27.51% (p < 0.001). Contrast-to-noise (CNR e ) ratios obtained from inclusion phantom indicate improved lesion detectability for both axial and lateral strain images. For instance, at 1.5% lateral strain, Bayesian regularization with five iterations had lateral CNR e of -0.31 dB which was significantly increased using the adaptive approach to 7.42 dB (p < 0.001). Similar results are seen with cardiac deformation modelling with improvement in myocardial strain images. In vivo feasibility was also demonstrated using data from a healthy murine heart. Overall, the proposed method makes Bayesian regularization robust for clinical and preclinical applications.
Figures
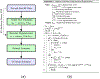
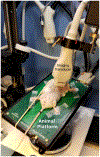
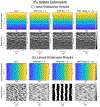
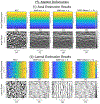
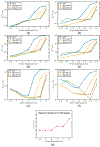
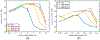
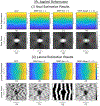
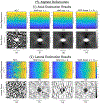
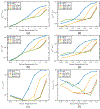
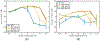
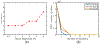
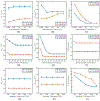
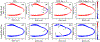
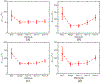
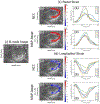
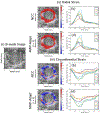
Similar articles
-
Hierarchical Motion Estimation With Bayesian Regularization in Cardiac Elastography: Simulation and In Vivo Validation.IEEE Trans Ultrason Ferroelectr Freq Control. 2019 Nov;66(11):1708-1722. doi: 10.1109/TUFFC.2019.2928546. Epub 2019 Jul 12. IEEE Trans Ultrason Ferroelectr Freq Control. 2019. PMID: 31329553 Free PMC article.
-
Improving Ultrasound Lateral Strain Estimation Accuracy using Log Compression of Regularized Correlation Function.Annu Int Conf IEEE Eng Med Biol Soc. 2020 Jul;2020:2031-2034. doi: 10.1109/EMBC44109.2020.9176531. Annu Int Conf IEEE Eng Med Biol Soc. 2020. PMID: 33018403 Free PMC article.
-
A coupled subsample displacement estimation method for ultrasound-based strain elastography.Phys Med Biol. 2015 Nov 7;60(21):8347-64. doi: 10.1088/0031-9155/60/21/8347. Epub 2015 Oct 12. Phys Med Biol. 2015. PMID: 26458219 Free PMC article.
-
Nonlinear regularization operators as derived from the micromorphic approach to gradient elasticity, viscoplasticity and damage.Proc Math Phys Eng Sci. 2016 Apr;472(2188):20150755. doi: 10.1098/rspa.2015.0755. Proc Math Phys Eng Sci. 2016. PMID: 27274684 Free PMC article. Review.
-
Regularization approaches in clinical biostatistics: A review of methods and their applications.Stat Methods Med Res. 2023 Feb;32(2):425-440. doi: 10.1177/09622802221133557. Epub 2022 Nov 16. Stat Methods Med Res. 2023. PMID: 36384320 Free PMC article. Review.
Cited by
-
Murine cardiac fibrosis localization using adaptive Bayesian cardiac strain imaging in vivo.Sci Rep. 2022 May 20;12(1):8522. doi: 10.1038/s41598-022-12579-6. Sci Rep. 2022. PMID: 35595876 Free PMC article.
-
Bayesian Regularized Strain Imaging for Assessment of Murine Cardiac Function In vivo.Annu Int Conf IEEE Eng Med Biol Soc. 2021 Nov;2021:2883-2886. doi: 10.1109/EMBC46164.2021.9630712. Annu Int Conf IEEE Eng Med Biol Soc. 2021. PMID: 34891849 Free PMC article.
-
Evaluation of illness severity of neonate infectious pneumonia and neurobehavioral development through ultrasonography under adaption algorithm.Pak J Med Sci. 2021;37(6):1682-1686. doi: 10.12669/pjms.37.6-WIT.4883. Pak J Med Sci. 2021. PMID: 34712306 Free PMC article.
-
In Vivo Adaptive Bayesian Regularized Lagrangian Carotid Strain Imaging for Murine Carotid Arteries and Its Associations With Histological Findings.Ultrasound Med Biol. 2023 Sep;49(9):2103-2112. doi: 10.1016/j.ultrasmedbio.2023.05.017. Epub 2023 Jul 1. Ultrasound Med Biol. 2023. PMID: 37400303 Free PMC article.
-
Spatiotemporal Bayesian Regularization for Cardiac Strain Imaging: Simulation and In Vivo Results.IEEE Open J Ultrason Ferroelectr Freq Control. 2021;1:21-36. doi: 10.1109/OJUFFC.2021.3130021. Epub 2021 Nov 22. IEEE Open J Ultrason Ferroelectr Freq Control. 2021. PMID: 35174360 Free PMC article.
References
-
- Alam SK and Ophir J 1997. Reduction of signal decorrelation from mechanical compression of tissues by temporal stretching: Applications to elastography Ultrasound in Medicine and Biology 23 95–105 - PubMed
-
- Ashikuzzaman M, Gauthier CJ and Rivaz H 2019. Global Ultrasound Elastography in Spatial and Temporal Domains IEEE transactions on ultrasonics, ferroelectrics, and frequency control 66 876–87 - PubMed
-
- Bilgen M and Insana M 1997 IEEE Ultrasonics Symposium Proceedings. An International Symposium (Cat. No. 97CH36118), 1997), vol. Series 2): IEEE; ) pp 1427–30
-
- Bohs LN, Friemel BH, McDermott BA and Trahey GE 1993. A real time system for quantifying and displaying two-dimensional velocities using ultrasound Ultrasound in medicine & biology 19 751–61 - PubMed
Publication types
MeSH terms
Grants and funding
LinkOut - more resources
Full Text Sources
Miscellaneous