GBDTCDA: Predicting circRNA-disease Associations Based on Gradient Boosting Decision Tree with Multiple Biological Data Fusion
- PMID: 31853227
- PMCID: PMC6909967
- DOI: 10.7150/ijbs.33806
GBDTCDA: Predicting circRNA-disease Associations Based on Gradient Boosting Decision Tree with Multiple Biological Data Fusion
Abstract
Circular RNA (circRNA) is a closed-loop structural non-coding RNA molecule which plays a significant role during the gene regulation processes. There are many previous studies shown that circRNAs can be regarded as the sponges of miRNAs. Thus, circRNA is also a key point for disease diagnosing, treating and inferring. However, traditional experimental approaches to verify the associations between the circRNA and disease are time-consuming and money-consuming. There are few computational models to predict potential circRNA-disease associations, which become our motivation to propose a new computational model. In this study, we propose a machine learning based computational model named Gradient Boosting Decision Tree with multiple biological data to predict circRNA-disease associations (GBDTCDA). The known circRNA-disease associations' data are downloaded from cricR2Disease database (http://bioinfo.snnu.edu.cn/CircR2Disease/). The feature vector of each circRNA-disease association pair is composed of four parts, which are the statistics information of different biological networks, the graph theory information of different biological networks, circRNA-disease associations' network information and circRNA nucleotide sequence information, respectively. Therefore, we use those feature vectors to train the gradient boosting decision tree regression model. Then, the leave one out cross validation (LOOCV) is adopted to evaluate the performance of our computational model. As for predicting some common diseases related circRNAs, our method GBDTCDA also obtains the better results. The Area under the ROC Curve (AUC) values of Basal cell carcinoma, Non-small cell lung cancer and cervical cancer are 95.8%, 88.3% and 93.5%, respectively. For further illustrating the performance of GBDTCDA, a case study of breast cancer is also supplemented in this study. Thus, our proposed method GBDTCDA is a powerful tool to predict potential circRNA-disease associations based on experimental results and analyses.
Keywords: Gradient Boosting; circRNA-disease associations; machine learning; multiple biological data.
© The author(s).
Conflict of interest statement
Competing Interests: The authors have declared that no competing interest exists.
Figures
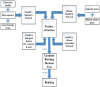
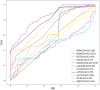
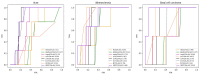
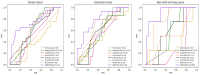
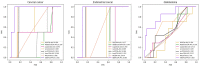
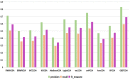
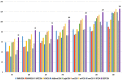
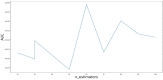
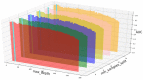
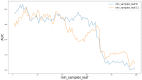
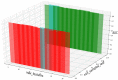
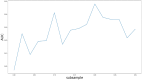
Similar articles
-
Circular RNAs and complex diseases: from experimental results to computational models.Brief Bioinform. 2021 Nov 5;22(6):bbab286. doi: 10.1093/bib/bbab286. Brief Bioinform. 2021. PMID: 34329377 Free PMC article. Review.
-
Predicting circRNA-Disease Associations Based on Improved Collaboration Filtering Recommendation System With Multiple Data.Front Genet. 2019 Sep 25;10:897. doi: 10.3389/fgene.2019.00897. eCollection 2019. Front Genet. 2019. PMID: 31608124 Free PMC article.
-
GCNCDA: A new method for predicting circRNA-disease associations based on Graph Convolutional Network Algorithm.PLoS Comput Biol. 2020 May 20;16(5):e1007568. doi: 10.1371/journal.pcbi.1007568. eCollection 2020 May. PLoS Comput Biol. 2020. PMID: 32433655 Free PMC article.
-
PWCDA: Path Weighted Method for Predicting circRNA-Disease Associations.Int J Mol Sci. 2018 Oct 31;19(11):3410. doi: 10.3390/ijms19113410. Int J Mol Sci. 2018. PMID: 30384427 Free PMC article.
-
Identification of circRNA-disease associations via multi-model fusion and ensemble learning.J Cell Mol Med. 2024 Apr;28(7):e18180. doi: 10.1111/jcmm.18180. J Cell Mol Med. 2024. PMID: 38506066 Free PMC article. Review.
Cited by
-
Circular RNAs: Their Role in the Pathogenesis and Orchestration of Breast Cancer.Front Cell Dev Biol. 2021 Mar 11;9:647736. doi: 10.3389/fcell.2021.647736. eCollection 2021. Front Cell Dev Biol. 2021. PMID: 33777954 Free PMC article. Review.
-
Circular RNAs and complex diseases: from experimental results to computational models.Brief Bioinform. 2021 Nov 5;22(6):bbab286. doi: 10.1093/bib/bbab286. Brief Bioinform. 2021. PMID: 34329377 Free PMC article. Review.
-
Double matrix completion for circRNA-disease association prediction.BMC Bioinformatics. 2021 Jun 8;22(1):307. doi: 10.1186/s12859-021-04231-3. BMC Bioinformatics. 2021. PMID: 34103016 Free PMC article.
-
Prioritizing CircRNA-Disease Associations With Convolutional Neural Network Based on Multiple Similarity Feature Fusion.Front Genet. 2020 Sep 16;11:540751. doi: 10.3389/fgene.2020.540751. eCollection 2020. Front Genet. 2020. PMID: 33193615 Free PMC article.
-
Hsa_circ_0000520 overexpression increases CDK2 expression via miR-1296 to facilitate cervical cancer cell proliferation.J Transl Med. 2021 Jul 20;19(1):314. doi: 10.1186/s12967-021-02953-9. J Transl Med. 2021. PMID: 34284793 Free PMC article.
References
-
- Memczak S, Jens M, Elefsinioti A, Torti F, Krueger J, Rybak A. et al. Circular RNAs are a large class of animal RNAs with regulatory potency. Nature. 2013;495:333. - PubMed
-
- Qu S, Yang X, Li X, Wang J, Gao Y, Shang R. et al. Circular RNA: A new star of noncoding RNAs. Cancer Lett. 2015;365:141–8. - PubMed
-
- Hsu MT, Coca-Prados M. Electron microscopic evidence for the circular form of RNA in the cytoplasm of eukaryotic cells. Nature. 1979;280:339–40. - PubMed
-
- Kos A, Dijkema R, Arnberg AC, van der Meide PH, Schellekens H. The hepatitis delta (delta) virus possesses a circular RNA. Nature. 1986;323:558–60. - PubMed
Publication types
MeSH terms
Substances
LinkOut - more resources
Full Text Sources
Research Materials