Deep learning modeling using normal mammograms for predicting breast cancer risk
- PMID: 31667873
- PMCID: PMC6980268
- DOI: 10.1002/mp.13886
Deep learning modeling using normal mammograms for predicting breast cancer risk
Abstract
Purpose: To investigate two deep learning-based modeling schemes for predicting short-term risk of developing breast cancer using prior normal screening digital mammograms in a case-control setting.
Methods: We conducted a retrospective Institutional Review Board-approved study on a case-control cohort of 226 patients (including 113 women diagnosed with breast cancer and 113 controls) who underwent general population breast cancer screening. For each patient, a prior normal (i.e., with negative or benign findings) digital mammogram examination [including mediolateral oblique (MLO) view and craniocaudal (CC) view two images] was collected. Thus, a total of 452 normal images (226 MLO view images and 226 CC view images) of this case-control cohort were analyzed to predict the outcome, i.e., developing breast cancer (cancer cases) or remaining breast cancer-free (controls) within the follow-up period. We implemented an end-to-end deep learning model and a GoogLeNet-LDA model and compared their effects in several experimental settings using two mammographic view images and inputting two different subregions of the images to the models. The proposed models were also compared to logistic regression modeling of mammographic breast density. Area under the receiver operating characteristic curve (AUC) was used as the model performance metric.
Results: The highest AUC was 0.73 [95% Confidence Interval (CI): 0.68-0.78; GoogLeNet-LDA model on CC view] when using the whole-breast and was 0.72 (95% CI: 0.67-0.76; GoogLeNet-LDA model on MLO + CC view) when using the dense tissue, respectively, as the model input. The GoogleNet-LDA model significantly (all P < 0.05) outperformed the end-to-end GoogLeNet model in all experiments. CC view was consistently more predictive than MLO view in both deep learning models, regardless of the input subregions. Both models exhibited superior performance than the percent breast density (AUC = 0.54; 95% CI: 0.49-0.59).
Conclusions: The proposed deep learning modeling approach can predict short-term breast cancer risk using normal screening mammogram images. Larger studies are needed to further reveal the promise of deep learning in enhancing imaging-based breast cancer risk assessment.
Keywords: breast cancer; breast density; deep learning; digital mammography; risk biomarkers.
© 2019 American Association of Physicists in Medicine.
Figures
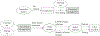
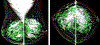
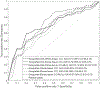
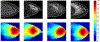
Similar articles
-
Understanding Clinical Mammographic Breast Density Assessment: a Deep Learning Perspective.J Digit Imaging. 2018 Aug;31(4):387-392. doi: 10.1007/s10278-017-0022-2. J Digit Imaging. 2018. PMID: 28932980 Free PMC article.
-
Assessment of a Four-View Mammographic Image Feature Based Fusion Model to Predict Near-Term Breast Cancer Risk.Ann Biomed Eng. 2015 Oct;43(10):2416-28. doi: 10.1007/s10439-015-1316-5. Epub 2015 Apr 8. Ann Biomed Eng. 2015. PMID: 25851469 Free PMC article.
-
Comparison of two-dimensional synthesized mammograms versus original digital mammograms: a quantitative assessment.Med Biol Eng Comput. 2021 Feb;59(2):355-367. doi: 10.1007/s11517-021-02313-1. Epub 2021 Jan 14. Med Biol Eng Comput. 2021. PMID: 33447988
-
Strategies to Increase Cancer Detection: Review of True-Positive and False-Negative Results at Digital Breast Tomosynthesis Screening.Radiographics. 2016 Nov-Dec;36(7):1954-1965. doi: 10.1148/rg.2016160049. Epub 2016 Oct 7. Radiographics. 2016. PMID: 27715711 Free PMC article. Review.
-
Deep Learning Approaches with Digital Mammography for Evaluating Breast Cancer Risk, a Narrative Review.Tomography. 2023 Jun 6;9(3):1110-1119. doi: 10.3390/tomography9030091. Tomography. 2023. PMID: 37368543 Free PMC article. Review.
Cited by
-
Artificial intelligence in mammographic phenotyping of breast cancer risk: a narrative review.Breast Cancer Res. 2022 Feb 20;24(1):14. doi: 10.1186/s13058-022-01509-z. Breast Cancer Res. 2022. PMID: 35184757 Free PMC article. Review.
-
Prediction of Breast Cancer using Machine Learning Approaches.J Biomed Phys Eng. 2022 Jun 1;12(3):297-308. doi: 10.31661/jbpe.v0i0.2109-1403. eCollection 2022 Jun. J Biomed Phys Eng. 2022. PMID: 35698545 Free PMC article.
-
Biosignal Compression Toolbox for Digital Biomarker Discovery.Sensors (Basel). 2021 Jan 13;21(2):516. doi: 10.3390/s21020516. Sensors (Basel). 2021. PMID: 33450898 Free PMC article.
-
An Assessment of the Predictive Performance of Current Machine Learning-Based Breast Cancer Risk Prediction Models: Systematic Review.JMIR Public Health Surveill. 2022 Dec 29;8(12):e35750. doi: 10.2196/35750. JMIR Public Health Surveill. 2022. PMID: 36426919 Free PMC article. Review.
-
CSA-Net: Channel and Spatial Attention-Based Network for Mammogram and Ultrasound Image Classification.J Imaging. 2024 Oct 16;10(10):256. doi: 10.3390/jimaging10100256. J Imaging. 2024. PMID: 39452419 Free PMC article.
References
-
- D’orsi C, Bassett L, Feig S, Breast imaging reporting and data system (BI-RADS), Breast imaging atlas, 4th edn. American College of Radiology, Reston: (1998).
-
- Ciatto S, Bernardi D, Calabrese M, Durando M, Gentilini MA, Mariscotti G, Monetti F, Moriconi E, Pesce B, Roselli A, A first evaluation of breast radiological density assessment by QUANTRA software as compared to visual classification, The Breast 21(4) (2012) 503–506. - PubMed
-
- Alain G, Bengio Y, Understanding intermediate layers using linear classifier probes, arXiv preprint arXiv:1610.01644 (2016).
-
- Boyd NF, Guo H, Martin LJ, Sun L, Stone J, Fishell E, Jong RA, Hislop G, Chiarelli A, Minkin S, Mammographic density and the risk and detection of breast cancer, New England Journal of Medicine 356(3) (2007) 227–236. - PubMed
MeSH terms
Grants and funding
LinkOut - more resources
Full Text Sources
Other Literature Sources
Medical