A yeast phenomic model for the influence of Warburg metabolism on genetic buffering of doxorubicin
- PMID: 31660150
- PMCID: PMC6806529
- DOI: 10.1186/s40170-019-0201-3
A yeast phenomic model for the influence of Warburg metabolism on genetic buffering of doxorubicin
Abstract
Background: The influence of the Warburg phenomenon on chemotherapy response is unknown. Saccharomyces cerevisiae mimics the Warburg effect, repressing respiration in the presence of adequate glucose. Yeast phenomic experiments were conducted to assess potential influences of Warburg metabolism on gene-drug interaction underlying the cellular response to doxorubicin. Homologous genes from yeast phenomic and cancer pharmacogenomics data were analyzed to infer evolutionary conservation of gene-drug interaction and predict therapeutic relevance.
Methods: Cell proliferation phenotypes (CPPs) of the yeast gene knockout/knockdown library were measured by quantitative high-throughput cell array phenotyping (Q-HTCP), treating with escalating doxorubicin concentrations under conditions of respiratory or glycolytic metabolism. Doxorubicin-gene interaction was quantified by departure of CPPs observed for the doxorubicin-treated mutant strain from that expected based on an interaction model. Recursive expectation-maximization clustering (REMc) and Gene Ontology (GO)-based analyses of interactions identified functional biological modules that differentially buffer or promote doxorubicin cytotoxicity with respect to Warburg metabolism. Yeast phenomic and cancer pharmacogenomics data were integrated to predict differential gene expression causally influencing doxorubicin anti-tumor efficacy.
Results: Yeast compromised for genes functioning in chromatin organization, and several other cellular processes are more resistant to doxorubicin under glycolytic conditions. Thus, the Warburg transition appears to alleviate requirements for cellular functions that buffer doxorubicin cytotoxicity in a respiratory context. We analyzed human homologs of yeast genes exhibiting gene-doxorubicin interaction in cancer pharmacogenomics data to predict causality for differential gene expression associated with doxorubicin cytotoxicity in cancer cells. This analysis suggested conserved cellular responses to doxorubicin due to influences of homologous recombination, sphingolipid homeostasis, telomere tethering at nuclear periphery, actin cortical patch localization, and other gene functions.
Conclusions: Warburg status alters the genetic network required for yeast to buffer doxorubicin toxicity. Integration of yeast phenomic and cancer pharmacogenomics data suggests evolutionary conservation of gene-drug interaction networks and provides a new experimental approach to model their influence on chemotherapy response. Thus, yeast phenomic models could aid the development of precision oncology algorithms to predict efficacious cytotoxic drugs for cancer, based on genetic and metabolic profiles of individual tumors.
Keywords: Cell proliferation parameters (CPPs); Differential gene interaction networks; Doxorubicin; Genetic buffering; Human-like/HL yeast media; Pharmacogenomics; Quantitative high throughput cell array phenotyping (Q-HTCP); Recursive expectation-maximization clustering (REMc); Warburg metabolism; Yeast phenomics.
© The Author(s). 2019.
Conflict of interest statement
Competing interestsJLH has ownership in the Spectrum PhenomX, LLC, a shell company that was formed to commercialize Q-HTCP technology. The authors declare that they have no other competing interests.
Figures
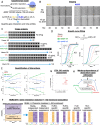
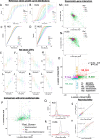
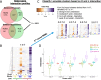
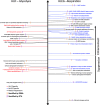
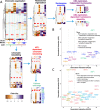
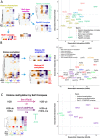
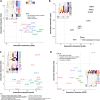
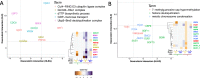
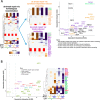
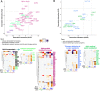
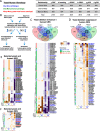
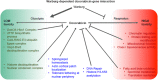
Similar articles
-
A Humanized Yeast Phenomic Model of Deoxycytidine Kinase to Predict Genetic Buffering of Nucleoside Analog Cytotoxicity.Genes (Basel). 2019 Sep 30;10(10):770. doi: 10.3390/genes10100770. Genes (Basel). 2019. PMID: 31575041 Free PMC article.
-
Recursive expectation-maximization clustering: a method for identifying buffering mechanisms composed of phenomic modules.Chaos. 2010 Jun;20(2):026103. doi: 10.1063/1.3455188. Chaos. 2010. PMID: 20590332 Free PMC article.
-
A yeast phenomic model for the gene interaction network modulating CFTR-ΔF508 protein biogenesis.Genome Med. 2012 Dec 27;4(12):103. doi: 10.1186/gm404. Genome Med. 2012. PMID: 23270647 Free PMC article.
-
Two transition states of the glycogen shunt and two steady states of gene expression support metabolic flexibility and the Warburg effect in cancer.Neoplasia. 2021 Sep;23(9):879-886. doi: 10.1016/j.neo.2021.06.004. Epub 2021 Jul 21. Neoplasia. 2021. PMID: 34303218 Free PMC article. Review.
-
Targeting Cancer Metabolism - Revisiting the Warburg Effects.Toxicol Res. 2016 Jul;32(3):177-93. doi: 10.5487/TR.2016.32.3.177. Epub 2016 Jul 30. Toxicol Res. 2016. PMID: 27437085 Free PMC article. Review.
Cited by
-
High-resolution yeast quiescence profiling in human-like media reveals complex influences of auxotrophy and nutrient availability.Geroscience. 2021 Apr;43(2):941-964. doi: 10.1007/s11357-020-00265-2. Epub 2020 Oct 5. Geroscience. 2021. PMID: 33015753 Free PMC article.
-
Effect of Collagen Matrix on Doxorubicin Distribution and Cancer Cells' Response to Treatment in 3D Tumor Model.Cancers (Basel). 2022 Nov 8;14(22):5487. doi: 10.3390/cancers14225487. Cancers (Basel). 2022. PMID: 36428580 Free PMC article.
-
Mitochondrial activities play a pivotal role in regulating cell cycle in response to doxorubicin.Cell Cycle. 2021 Jun;20(11):1067-1079. doi: 10.1080/15384101.2021.1919839. Epub 2021 May 12. Cell Cycle. 2021. PMID: 33978554 Free PMC article.
-
A Humanized Yeast Phenomic Model of Deoxycytidine Kinase to Predict Genetic Buffering of Nucleoside Analog Cytotoxicity.Genes (Basel). 2019 Sep 30;10(10):770. doi: 10.3390/genes10100770. Genes (Basel). 2019. PMID: 31575041 Free PMC article.
References
-
- Diaz-Ruiz R, Rigoulet M, Devin A. The Warburg and Crabtree effects: on the origin of cancer cell energy metabolism and of yeast glucose repression. Biochim Biophys Acta. 1807;2011:568–576. - PubMed
-
- Diaz-Ruiz R, Uribe-Carvajal S, Devin A, Rigoulet M. Tumor cell energy metabolism and its common features with yeast metabolism. Biochim Biophys Acta. 2009;1796:252–265. - PubMed
Grants and funding
LinkOut - more resources
Full Text Sources
Molecular Biology Databases