Effective Diagnosis of Alzheimer's Disease via Multimodal Fusion Analysis Framework
- PMID: 31649738
- PMCID: PMC6795747
- DOI: 10.3389/fgene.2019.00976
Effective Diagnosis of Alzheimer's Disease via Multimodal Fusion Analysis Framework
Abstract
Alzheimer's disease (AD) is a complex neurodegenerative disease involving a variety of pathogenic factors, and the etiology detection of this disease has been a major concern of researchers. Neuroimaging is a basic and important means to explore the problem. It is the main current scientific research direction for combining neuroimaging with other modal data to dig deep into the potential information of AD through the complementarities among multiple data points. Machine learning methods possess great potentiality and have reached some achievements in this research area. A few studies have proposed some solutions to the effects of multimodal data fusion, however, the overall analytical framework for data fusion and fusion result analysis has thus far been ignored. In this paper, we first put forward a novel multimodal data fusion method, and further present a new machine learning framework of data fusion, classification, feature selection, and disease-causing factor extraction. The real dataset of 37 AD patients and 35 normal controls (NC) with functional magnetic resonance imaging (fMRI) and genetic data was used to verify the effectiveness of the framework, which was more accurate in classification and optimal feature extraction than other methods. Furthermore, we revealed disease-causing brain regions and genes, such as the olfactory cortex, insula, posterior cingulate gyrus, lingual gyrus, CNTNAP2, LRP1B, FRMD4A, and DAB1. The results show that the machine learning framework could effectively perform multimodal data fusion analysis, providing new insights and perspectives for the diagnosis of Alzheimer's disease.
Keywords: Alzheimer’s disease; disease diagnosis; functional magnetic resonance imaging; gene; multimodal fusion analysis framework.
Copyright © 2019 Bi, Cai, Wang and Liu.
Figures
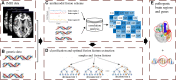
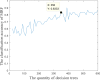
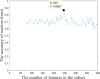
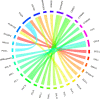
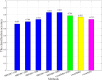
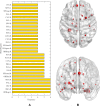
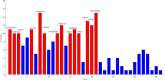
Similar articles
-
Voxel-wise Fusion of Resting fMRI Networks and Gray Matter Volume for Alzheimer's Disease Classification using Deep Multimodal Learning.Res Sq [Preprint]. 2023 Dec 13:rs.3.rs-3740218. doi: 10.21203/rs.3.rs-3740218/v1. Res Sq. 2023. PMID: 38168287 Free PMC article. Preprint.
-
Classification and Graphical Analysis of Alzheimer's Disease and Its Prodromal Stage Using Multimodal Features From Structural, Diffusion, and Functional Neuroimaging Data and the APOE Genotype.Front Aging Neurosci. 2020 Jul 30;12:238. doi: 10.3389/fnagi.2020.00238. eCollection 2020. Front Aging Neurosci. 2020. PMID: 32848713 Free PMC article.
-
Multimodal Data Fusion of Deep Learning and Dynamic Functional Connectivity Features to Predict Alzheimer's Disease Progression.Annu Int Conf IEEE Eng Med Biol Soc. 2019 Jul;2019:4409-4413. doi: 10.1109/EMBC.2019.8856500. Annu Int Conf IEEE Eng Med Biol Soc. 2019. PMID: 31946844
-
A survey on applications and analysis methods of functional magnetic resonance imaging for Alzheimer's disease.J Neurosci Methods. 2019 Apr 1;317:121-140. doi: 10.1016/j.jneumeth.2018.12.012. Epub 2018 Dec 26. J Neurosci Methods. 2019. PMID: 30593787 Review.
-
Multimodal Neuroimaging: Basic Concepts and Classification of Neuropsychiatric Diseases.Clin EEG Neurosci. 2019 Jan;50(1):20-33. doi: 10.1177/1550059418782093. Epub 2018 Jun 20. Clin EEG Neurosci. 2019. PMID: 29925268 Review.
Cited by
-
A review of brain imaging biomarker genomics in Alzheimer's disease: implementation and perspectives.Transl Neurodegener. 2022 Sep 15;11(1):42. doi: 10.1186/s40035-022-00315-z. Transl Neurodegener. 2022. PMID: 36109823 Free PMC article. Review.
-
Deep multiview learning to identify imaging-driven subtypes in mild cognitive impairment.BMC Bioinformatics. 2022 Sep 29;23(Suppl 3):402. doi: 10.1186/s12859-022-04946-x. BMC Bioinformatics. 2022. PMID: 36175853 Free PMC article.
-
CNTNAP2 Protein Is Degraded by the Ubiquitin-Proteasome System and the Macroautophagy-Lysosome Pathway.Mol Neurobiol. 2023 May;60(5):2455-2469. doi: 10.1007/s12035-023-03227-9. Epub 2023 Jan 20. Mol Neurobiol. 2023. PMID: 36658382
-
Machine learning for the life-time risk prediction of Alzheimer's disease: a systematic review.Brain Commun. 2021 Oct 21;3(4):fcab246. doi: 10.1093/braincomms/fcab246. eCollection 2021. Brain Commun. 2021. PMID: 34805994 Free PMC article. Review.
-
AI-Aided Disease Prediction in Visualized Medicine.Adv Exp Med Biol. 2023;1199:107-126. doi: 10.1007/978-981-32-9902-3_6. Adv Exp Med Biol. 2023. PMID: 37460729
References
-
- Association A. s. (2017). 2017 Alzheimer’s disease facts and figures. Alzheimer’s Dement. 13 (4), 325–373. 10.1016/j.jalz.2017.02.001 - DOI
-
- Association A. s. (2018). 2018 Alzheimer’s disease facts and figures. Alzheimer’s Dement. 14 (3), 367–429. 10.1016/j.jalz.2018.02.001 - DOI
-
- Benoit M. E., Hernandez M. X., Dinh M. L., Benavente F., Vasquez O., Tenner A. J. (2013). C1q-induced LRP1B and GPR6 proteins expressed early in Alzheimer disease mouse models, are essential for the C1q-mediated protection against amyloid-β neurotoxicity. J. Biol. Chem. 288 (1), 654–665. 10.1074/jbc.M112.400168 - DOI - PMC - PubMed
LinkOut - more resources
Full Text Sources