Deep Learning Implicitly Handles Tissue Specific Phenomena to Predict Tumor DNA Accessibility and Immune Activity
- PMID: 31563852
- PMCID: PMC6823659
- DOI: 10.1016/j.isci.2019.09.018
Deep Learning Implicitly Handles Tissue Specific Phenomena to Predict Tumor DNA Accessibility and Immune Activity
Abstract
DNA accessibility is a key dynamic feature of chromatin regulation that can potentiate transcriptional events and tumor progression. To gain insight into chromatin state across existing tumor data, we improved neural network models for predicting accessibility from DNA sequence and extended them to incorporate a global set of RNA sequencing gene expression inputs. Our expression-informed model expanded the application domain beyond specific tissue types to tissues not present in training and achieved consistently high accuracy in predicting DNA accessibility at promoter and promoter flank regions. We then leveraged our new tool by analyzing the DNA accessibility landscape of promoters across The Cancer Genome Atlas. We show that in lung adenocarcinoma the accessibility perspective uniquely highlights immune pathways inversely correlated with a more open chromatin state and that accessibility patterns learned from even a single tumor type can discriminate immune inflammation across many cancers, often with direct relation to patient prognosis.
Keywords: Bioinformatics; Cancer; Neural Networks.
Copyright © 2019 The Author(s). Published by Elsevier Inc. All rights reserved.
Conflict of interest statement
This work was funded by NantWorks affiliates (ImmunityBio, NantOmics, NantHealth) and performed by its employees; there are no other conflicts of interest.
Figures
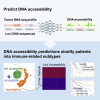
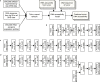
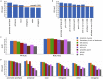
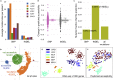
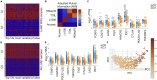
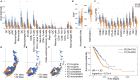
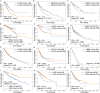
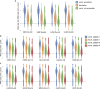
Similar articles
-
Modeling chromatin state from sequence across angiosperms using recurrent convolutional neural networks.Plant Genome. 2022 Sep;15(3):e20249. doi: 10.1002/tpg2.20249. Epub 2022 Aug 3. Plant Genome. 2022. PMID: 35924336
-
DeepPHiC: predicting promoter-centered chromatin interactions using a novel deep learning approach.Bioinformatics. 2023 Jan 1;39(1):btac801. doi: 10.1093/bioinformatics/btac801. Bioinformatics. 2023. PMID: 36495179 Free PMC article.
-
Differential chromatin accessibility landscape of gain-of-function mutant p53 tumours.BMC Cancer. 2021 Jun 5;21(1):669. doi: 10.1186/s12885-021-08362-x. BMC Cancer. 2021. PMID: 34090364 Free PMC article.
-
Distributed probing of chromatin structure in vivo reveals pervasive chromatin accessibility for expressed and non-expressed genes during tissue differentiation in C. elegans.BMC Genomics. 2010 Aug 6;11:465. doi: 10.1186/1471-2164-11-465. BMC Genomics. 2010. PMID: 20691096 Free PMC article.
-
A survey on protein-DNA-binding sites in computational biology.Brief Funct Genomics. 2022 Sep 16;21(5):357-375. doi: 10.1093/bfgp/elac009. Brief Funct Genomics. 2022. PMID: 35652477 Review.
Cited by
-
A Study of the Recent Trends of Immunology: Key Challenges, Domains, Applications, Datasets, and Future Directions.Sensors (Basel). 2021 Nov 23;21(23):7786. doi: 10.3390/s21237786. Sensors (Basel). 2021. PMID: 34883787 Free PMC article. Review.
-
Genome-wide Prediction of Chromatin Accessibility Based on Gene Expression.Wiley Interdiscip Rev Comput Stat. 2021 Sep-Oct;13(5):e1544. doi: 10.1002/wics.1544. Epub 2020 Dec 17. Wiley Interdiscip Rev Comput Stat. 2021. PMID: 39391743 Free PMC article.
-
Accelerating in silico saturation mutagenesis using compressed sensing.Bioinformatics. 2022 Jul 11;38(14):3557-3564. doi: 10.1093/bioinformatics/btac385. Bioinformatics. 2022. PMID: 35678521 Free PMC article.
References
-
- Alipanahi B., Delong A., Weirauch M.T., Frey B.J. Predicting the sequence specificities of DNA- and RNA-binding proteins by deep learning. Nat. Biotechnol. 2015;33:831–838. - PubMed
-
- Bahdanau D., Cho K., Bengio Y. Neural machine translation by jointly learning to align and translate. arxiv.org. 2014 arXiv:1409.0473.
LinkOut - more resources
Full Text Sources