The Evolution of Variance Control
- PMID: 31519463
- PMCID: PMC7482585
- DOI: 10.1016/j.tree.2019.08.005
The Evolution of Variance Control
Abstract
Genetically identical individuals can be phenotypically variable, even in constant environmental conditions. The ubiquity of this phenomenon, known as 'intra-genotypic variability', is increasingly evident and the relevant mechanistic underpinnings are beginning to be understood. In parallel, theory has delineated a number of formal expectations for contexts in which such a feature would be adaptive. Here, we review empirical evidence across biological systems and theoretical expectations, including nonlinear averaging and bet hedging. We synthesize existing results to illustrate the dependence of selection outcomes both on trait characteristics, features of environmental variability, and species' demographic context. We conclude by discussing ways to bridge the gap between empirical evidence of intra-genotypic variability, studies demonstrating its genetic component, and evidence that it is adaptive.
Keywords: bet hedging; intra-genotypic variability; micro-environmental plasticity; nonlinear averaging; phenotypic variance; vQTL.
Copyright © 2019. Published by Elsevier Ltd.
Figures
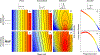
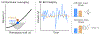
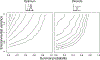
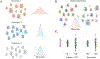
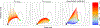
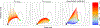
Similar articles
-
When sensing is gambling: An experimental system reveals how plasticity can generate tunable bet-hedging strategies.Evolution. 2017 Apr;71(4):859-871. doi: 10.1111/evo.13199. Epub 2017 Mar 7. Evolution. 2017. PMID: 28213964 Free PMC article.
-
Short-term insurance versus long-term bet-hedging strategies as adaptations to variable environments.Evolution. 2019 Feb;73(2):145-157. doi: 10.1111/evo.13659. Epub 2018 Dec 26. Evolution. 2019. PMID: 30549260 Free PMC article.
-
Fluctuating natural selection accounts for the evolution of diversification bet hedging.Proc Biol Sci. 2009 Jun 7;276(1664):1987-92. doi: 10.1098/rspb.2008.1920. Epub 2009 Mar 4. Proc Biol Sci. 2009. PMID: 19324774 Free PMC article.
-
Modes of response to environmental change and the elusive empirical evidence for bet hedging.Proc Biol Sci. 2011 Jun 7;278(1712):1601-9. doi: 10.1098/rspb.2011.0176. Epub 2011 Mar 16. Proc Biol Sci. 2011. PMID: 21411456 Free PMC article. Review.
-
Evolutionary bet-hedging in the real world: empirical evidence and challenges revealed by plants.Proc Biol Sci. 2010 Oct 22;277(1697):3055-64. doi: 10.1098/rspb.2010.0707. Epub 2010 Jun 23. Proc Biol Sci. 2010. PMID: 20573624 Free PMC article. Review.
Cited by
-
Species interactions drive continuous assembly of freshwater communities in stochastic environments.Sci Rep. 2024 Sep 18;14(1):21747. doi: 10.1038/s41598-024-72405-z. Sci Rep. 2024. PMID: 39294211 Free PMC article.
-
Optimal phenology of life history events in Calanus finmarchicus: exit from diapause in relation to interannual variation in spring bloom timing and predation.J Plankton Res. 2024 Jun 7;46(4):439-451. doi: 10.1093/plankt/fbae028. eCollection 2024 Jul-Aug. J Plankton Res. 2024. PMID: 39091692 Free PMC article.
-
Wolbachia impacts microbiome diversity and fitness-associated traits for Drosophila melanogaster in a seasonally fluctuating environment.Ecol Evol. 2024 Jul 22;14(7):e70004. doi: 10.1002/ece3.70004. eCollection 2024 Jul. Ecol Evol. 2024. PMID: 39041013 Free PMC article.
-
Environmental memory alters the fitness effects of adaptive mutations in fluctuating environments.Nat Ecol Evol. 2024 Sep;8(9):1760-1775. doi: 10.1038/s41559-024-02475-9. Epub 2024 Jul 17. Nat Ecol Evol. 2024. PMID: 39020024
-
Genetic architecture of trait variance in craniofacial morphology.Genetics. 2024 Apr 3;226(4):iyae028. doi: 10.1093/genetics/iyae028. Genetics. 2024. PMID: 38386896
References
-
- Lande R (1976) Natural selection and random genetic drift in phenotype evolution. Evolution 30, 314–334 - PubMed
-
- Piersma T and Drent J (2003) Phenotypic flexibility and the evolution of organismal design. Trends Ecol. Evol 18, 228–233
-
- Bradshaw AD (1965) Evolutionary significance of phenotypic plasticity in plants. Adv. Genet 13, 116–155
-
- Philippi T and Seger J (1989) Hedging one’s evolutionary bets, revisited. Trends Ecol. Evol 4, 41–44 - PubMed
-
- Garreau H et al. (2008) Results of four generations of a canalising selection for rabbit birth weight. Livest. Sci 119, 55–62
Publication types
MeSH terms
Grants and funding
LinkOut - more resources
Full Text Sources
Miscellaneous