Predicting short- and long-term glycated haemoglobin response after insulin initiation in patients with type 2 diabetes mellitus using machine-learning algorithms
- PMID: 31453664
- PMCID: PMC6899933
- DOI: 10.1111/dom.13860
Predicting short- and long-term glycated haemoglobin response after insulin initiation in patients with type 2 diabetes mellitus using machine-learning algorithms
Abstract
Aim: To assess the potential of supervised machine-learning techniques to identify clinical variables for predicting short-term and long-term glycated haemoglobin (HbA1c) response after insulin treatment initiation in patients with type 2 diabetes mellitus (T2DM).
Materials and methods: We included patients with T2DM from the Groningen Initiative to Analyse Type 2 diabetes Treatment (GIANTT) database who started insulin treatment between 2007 and 2013 and had a minimum follow-up of 2 years. Short- and long-term responses at 6 (±2) and 24 (±2) months after insulin initiation, respectively, were assessed. Patients were defined as good responders if they had a decrease in HbA1c ≥ 5 mmol/mol or reached the recommended level of HbA1c ≤ 53 mmol/mol. Twenty-four baseline clinical variables were used for the analysis and an elastic net regularization technique was used for variable selection. The performance of three traditional machine-learning algorithms was compared for the prediction of short- and long-term responses and the area under the receiver-operating characteristic curve (AUC) was used to assess the performance of the prediction models.
Results: The elastic net regularization-based generalized linear model, which included baseline HbA1c and estimated glomerular filtration rate, correctly classified short- and long-term HbA1c response after treatment initiation, with AUCs of 0.80 (95% CI 0.78-0.83) and 0.81 (95% CI 0.79-0.84), respectively, and outperformed the other machine-learning algorithms. Using baseline HbA1c alone, an AUC = 0.71 (95% CI 0.65-0.73) and 0.72 (95% CI 0.66-0.75) was obtained for predicting short-term and long-term response, respectively.
Conclusions: Machine-learning algorithm performed well in the prediction of an individual's short-term and long-term HbA1c response using baseline clinical variables.
Keywords: cohort study; database research; insulin therapy; observational study; primary care; type 2 diabetes.
© 2019 The Authors. Diabetes, Obesity and Metabolism published by John Wiley & Sons Ltd.
Conflict of interest statement
The authors have no conflicts of interest.
Figures
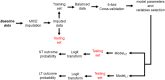
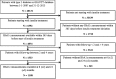
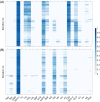
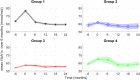
Similar articles
-
No significant association of type 2 diabetes-related genetic risk scores with glycated haemoglobin levels after initiating metformin or sulphonylurea derivatives.Diabetes Obes Metab. 2019 Oct;21(10):2267-2273. doi: 10.1111/dom.13803. Epub 2019 Jun 24. Diabetes Obes Metab. 2019. PMID: 31168905 Free PMC article.
-
HbA1c response after insulin initiation in patients with type 2 diabetes mellitus in real life practice: Identifying distinct subgroups.Diabetes Obes Metab. 2018 Aug;20(8):1957-1964. doi: 10.1111/dom.13332. Epub 2018 May 24. Diabetes Obes Metab. 2018. PMID: 29687577 Free PMC article.
-
Longitudinal changes in glycated haemoglobin following treatment intensification after inadequate response to two oral antidiabetic agents in patients with type 2 diabetes.Diabetes Obes Metab. 2019 Jul;21(7):1725-1733. doi: 10.1111/dom.13694. Epub 2019 Apr 5. Diabetes Obes Metab. 2019. PMID: 30848039 Free PMC article.
-
Short acting insulin analogues versus regular human insulin in patients with diabetes mellitus.Cochrane Database Syst Rev. 2004 Oct 18;(4):CD003287. doi: 10.1002/14651858.CD003287.pub3. Cochrane Database Syst Rev. 2004. Update in: Cochrane Database Syst Rev. 2006 Apr 19;(2):CD003287. doi: 10.1002/14651858.CD003287.pub4. PMID: 15495047 Updated. Review.
-
Short acting insulin analogues versus regular human insulin in patients with diabetes mellitus.Cochrane Database Syst Rev. 2004;(2):CD003287. doi: 10.1002/14651858.CD003287.pub2. Cochrane Database Syst Rev. 2004. Update in: Cochrane Database Syst Rev. 2004 Oct 18;(4):CD003287. doi: 10.1002/14651858.CD003287.pub3. PMID: 15106199 Updated. Review.
Cited by
-
Predicting Response to Bolus Insulin Therapy in Patients With Type 2 Diabetes.J Diabetes Sci Technol. 2023 Nov;17(6):1573-1579. doi: 10.1177/19322968221098057. Epub 2022 May 20. J Diabetes Sci Technol. 2023. PMID: 35596567 Free PMC article.
-
Implications of Artificial Intelligence Algorithms in the Diagnosis and Treatment of Motor Neuron Diseases-A Review.Life (Basel). 2023 Apr 17;13(4):1031. doi: 10.3390/life13041031. Life (Basel). 2023. PMID: 37109560 Free PMC article.
-
Machine Learning Approaches to Predict Risks of Diabetic Complications and Poor Glycemic Control in Nonadherent Type 2 Diabetes.Front Pharmacol. 2021 Jun 22;12:665951. doi: 10.3389/fphar.2021.665951. eCollection 2021. Front Pharmacol. 2021. PMID: 34239440 Free PMC article.
-
Estimating heritability of glycaemic response to metformin using nationwide electronic health records and population-sized pedigree.Commun Med (Lond). 2021 Dec 1;1:55. doi: 10.1038/s43856-021-00058-4. eCollection 2021. Commun Med (Lond). 2021. PMID: 35602224 Free PMC article.
-
Machine Learning Techniques to Predict Timeliness of Care among Lung Cancer Patients.Healthcare (Basel). 2023 Oct 18;11(20):2756. doi: 10.3390/healthcare11202756. Healthcare (Basel). 2023. PMID: 37893830 Free PMC article.
References
-
- Rutten G, Grauw WD, Nijpels G, et al. NHG‐Standaard Diabetes mellitus type 2 (derde herziening). Huisarts Wet. 2013;56:512‐525.
-
- Cantrell RA, Alatorre CI, Davis EJ, et al. A review of treatment response in type 2 diabetes: assessing the role of patient heterogeneity. Diabetes Obes Metab. 2010;12(10):845‐857. - PubMed
Publication types
MeSH terms
Substances
LinkOut - more resources
Full Text Sources
Medical