Support Vector Machine model for hERG inhibitory activities based on the integrated hERG database using descriptor selection by NSGA-II
- PMID: 31434908
- PMCID: PMC6704061
- DOI: 10.1038/s41598-019-47536-3
Support Vector Machine model for hERG inhibitory activities based on the integrated hERG database using descriptor selection by NSGA-II
Abstract
Assessing the hERG liability in the early stages of drug discovery programs is important. The recent increase of hERG-related information in public databases enabled various successful applications of machine learning techniques to predict hERG inhibition. However, most of these researches constructed the datasets from only one database, limiting the predictability and scope of the models. In this study, a hERG classification model was constructed using the largest dataset for hERG inhibition built by integrating multiple databases. The integrated dataset consisted of more than 291,000 structurally diverse compounds derived from ChEMBL, GOSTAR, PubChem, and hERGCentral. The prediction model was built by support vector machine (SVM) with descriptor selection based on Non-dominated Sorting Genetic Algorithm-II (NSGA-II) to optimize the descriptor set for maximum prediction performance with the minimal number of descriptors. The SVM classification model using 72 selected descriptors and ECFP_4 structural fingerprints recorded kappa statistics of 0.733 and accuracy of 0.984 for the test set, substantially outperforming the prediction performance of the current commercial applications for hERG prediction. Finally, the applicability domain of the prediction model was assessed based on the molecular similarity between the training set and test set compounds.
Conflict of interest statement
The authors declare no competing interests.
Figures
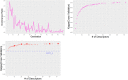
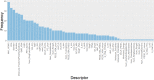
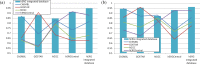
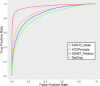
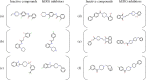
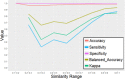
Similar articles
-
A comprehensive support vector machine binary hERG classification model based on extensive but biased end point hERG data sets.Chem Res Toxicol. 2011 Jun 20;24(6):934-49. doi: 10.1021/tx200099j. Epub 2011 May 6. Chem Res Toxicol. 2011. PMID: 21504223
-
Construction of an integrated database for hERG blocking small molecules.PLoS One. 2018 Jul 6;13(7):e0199348. doi: 10.1371/journal.pone.0199348. eCollection 2018. PLoS One. 2018. PMID: 29979714 Free PMC article.
-
ADMET Evaluation in Drug Discovery. 16. Predicting hERG Blockers by Combining Multiple Pharmacophores and Machine Learning Approaches.Mol Pharm. 2016 Aug 1;13(8):2855-66. doi: 10.1021/acs.molpharmaceut.6b00471. Epub 2016 Jul 18. Mol Pharm. 2016. PMID: 27379394
-
In silico prediction of hERG potassium channel blockage by chemical category approaches.Toxicol Res (Camb). 2016 Jan 14;5(2):570-582. doi: 10.1039/c5tx00294j. eCollection 2016 Mar 1. Toxicol Res (Camb). 2016. PMID: 30090371 Free PMC article.
-
Computer-Aided Synthesis Planning (CASP) and Machine Learning: Optimizing Chemical Reaction Conditions.Chemistry. 2024 Oct 1;30(55):e202401626. doi: 10.1002/chem.202401626. Epub 2024 Sep 17. Chemistry. 2024. PMID: 39083362 Review.
Cited by
-
The Trends and Future Prospective of In Silico Models from the Viewpoint of ADME Evaluation in Drug Discovery.Pharmaceutics. 2023 Nov 12;15(11):2619. doi: 10.3390/pharmaceutics15112619. Pharmaceutics. 2023. PMID: 38004597 Free PMC article. Review.
-
Prediction of arrhythmia susceptibility through mathematical modeling and machine learning.Proc Natl Acad Sci U S A. 2021 Sep 14;118(37):e2104019118. doi: 10.1073/pnas.2104019118. Proc Natl Acad Sci U S A. 2021. PMID: 34493665 Free PMC article.
-
PubChem 2023 update.Nucleic Acids Res. 2023 Jan 6;51(D1):D1373-D1380. doi: 10.1093/nar/gkac956. Nucleic Acids Res. 2023. PMID: 36305812 Free PMC article.
-
Toward Quantitative Models in Safety Assessment: A Case Study to Show Impact of Dose-Response Inference on hERG Inhibition Models.Int J Mol Sci. 2022 Dec 30;24(1):635. doi: 10.3390/ijms24010635. Int J Mol Sci. 2022. PMID: 36614078 Free PMC article.
-
Virtual screening of DrugBank database for hERG blockers using topological Laplacian-assisted AI models.Comput Biol Med. 2023 Feb;153:106491. doi: 10.1016/j.compbiomed.2022.106491. Epub 2022 Dec 28. Comput Biol Med. 2023. PMID: 36599209 Free PMC article.
References
-
- Kennedy T. Managing the drug discovery/development interface. Drug Discov. Today. 1997;2:436–444. doi: 10.1016/S1359-6446(97)01099-4. - DOI
-
- Redfern WS, et al. Relationships between Preclinical Cardiac Electrophysiology, Clinical QT Interval Prolongation and Torsade de Pointes for a Broad Range of Drugs: Evidence for a Provisional Safety Margin in Drug Development. Cardiovasc. Res. 2003;58:32–45. doi: 10.1016/S0008-6363(02)00846-5. - DOI - PubMed
Publication types
LinkOut - more resources
Full Text Sources