Machine and deep learning meet genome-scale metabolic modeling
- PMID: 31295267
- PMCID: PMC6622478
- DOI: 10.1371/journal.pcbi.1007084
Machine and deep learning meet genome-scale metabolic modeling
Abstract
Omic data analysis is steadily growing as a driver of basic and applied molecular biology research. Core to the interpretation of complex and heterogeneous biological phenotypes are computational approaches in the fields of statistics and machine learning. In parallel, constraint-based metabolic modeling has established itself as the main tool to investigate large-scale relationships between genotype, phenotype, and environment. The development and application of these methodological frameworks have occurred independently for the most part, whereas the potential of their integration for biological, biomedical, and biotechnological research is less known. Here, we describe how machine learning and constraint-based modeling can be combined, reviewing recent works at the intersection of both domains and discussing the mathematical and practical aspects involved. We overlap systematic classifications from both frameworks, making them accessible to nonexperts. Finally, we delineate potential future scenarios, propose new joint theoretical frameworks, and suggest concrete points of investigation for this joint subfield. A multiview approach merging experimental and knowledge-driven omic data through machine learning methods can incorporate key mechanistic information in an otherwise biologically-agnostic learning process.
Conflict of interest statement
The authors have declared that no competing interests exist.
Figures
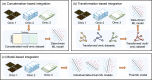
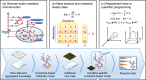
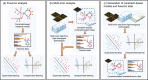
Similar articles
-
Machine learning for the advancement of genome-scale metabolic modeling.Biotechnol Adv. 2024 Sep;74:108400. doi: 10.1016/j.biotechadv.2024.108400. Epub 2024 Jun 27. Biotechnol Adv. 2024. PMID: 38944218 Review.
-
A Practical Guide to Integrating Multimodal Machine Learning and Metabolic Modeling.Methods Mol Biol. 2022;2399:87-122. doi: 10.1007/978-1-0716-1831-8_5. Methods Mol Biol. 2022. PMID: 35604554
-
A mechanism-aware and multiomic machine-learning pipeline characterizes yeast cell growth.Proc Natl Acad Sci U S A. 2020 Aug 4;117(31):18869-18879. doi: 10.1073/pnas.2002959117. Epub 2020 Jul 16. Proc Natl Acad Sci U S A. 2020. PMID: 32675233 Free PMC article.
-
Machine learning, the kidney, and genotype-phenotype analysis.Kidney Int. 2020 Jun;97(6):1141-1149. doi: 10.1016/j.kint.2020.02.028. Epub 2020 Apr 1. Kidney Int. 2020. PMID: 32359808 Free PMC article. Review.
-
Combining handcrafted features with latent variables in machine learning for prediction of radiation-induced lung damage.Med Phys. 2019 May;46(5):2497-2511. doi: 10.1002/mp.13497. Epub 2019 Apr 8. Med Phys. 2019. PMID: 30891794 Free PMC article.
Cited by
-
Computational Biology and Machine Learning Approaches to Understand Mechanistic Microbiome-Host Interactions.Front Microbiol. 2021 May 11;12:618856. doi: 10.3389/fmicb.2021.618856. eCollection 2021. Front Microbiol. 2021. PMID: 34046017 Free PMC article. Review.
-
Perspectives on computational modeling of biological systems and the significance of the SysMod community.Bioinform Adv. 2024 Jun 26;4(1):vbae090. doi: 10.1093/bioadv/vbae090. eCollection 2024. Bioinform Adv. 2024. PMID: 38948011 Free PMC article.
-
Flux sampling in genome-scale metabolic modeling of microbial communities.BMC Bioinformatics. 2024 Jan 29;25(1):45. doi: 10.1186/s12859-024-05655-3. BMC Bioinformatics. 2024. PMID: 38287239 Free PMC article.
-
Deep Learning for Smart Healthcare-A Survey on Brain Tumor Detection from Medical Imaging.Sensors (Basel). 2022 Mar 2;22(5):1960. doi: 10.3390/s22051960. Sensors (Basel). 2022. PMID: 35271115 Free PMC article. Review.
-
Exploring synergies between plant metabolic modelling and machine learning.Comput Struct Biotechnol J. 2022 Apr 16;20:1885-1900. doi: 10.1016/j.csbj.2022.04.016. eCollection 2022. Comput Struct Biotechnol J. 2022. PMID: 35521559 Free PMC article. Review.
References
Publication types
MeSH terms
Grants and funding
LinkOut - more resources
Full Text Sources
Other Literature Sources