Anticancer Drug Response Prediction in Cell Lines Using Weighted Graph Regularized Matrix Factorization
- PMID: 31265947
- PMCID: PMC6610642
- DOI: 10.1016/j.omtn.2019.05.017
Anticancer Drug Response Prediction in Cell Lines Using Weighted Graph Regularized Matrix Factorization
Abstract
Precision medicine has become a novel and rising concept, which depends much on the identification of individual genomic signatures for different patients. The cancer cell lines could reflect the "omic" diversity of primary tumors, based on which many works have been carried out to study the cancer biology and drug discovery both in experimental and computational aspects. In this work, we presented a novel method to utilize weighted graph regularized matrix factorization (WGRMF) for inferring anticancer drug response in cell lines. We constructed a p-nearest neighbor graph to sparsify drug similarity matrix and cell line similarity matrix, respectively. Using the sparsified matrices in the graph regularization terms, we performed matrix factorization to generate the latent matrices for drug and cell line. The graph regularization terms including neighbor information could help to exclude the noisy ingredient and improve the prediction accuracy. The 10-fold cross-validation was implemented, and the Pearson correlation coefficient (PCC), root-mean-square error (RMSE), PCCsr, and RMSEsr averaged over all drugs were calculated to evaluate the performance of WGRMF. The results on the Genomics of Drug Sensitivity in Cancer (GDSC) dataset are 0.64 ± 0.16, 1.37 ± 0.35, 0.73 ± 0.14, and 1.71 ± 0.44 for PCC, RMSE, PCCsr, and RMSEsr in turn. And for the Cancer Cell Line Encyclopedia (CCLE) dataset, WGRMF got results of 0.72 ± 0.09, 0.56 ± 0.19, 0.79 ± 0.07, and 0.69 ± 0.19, respectively. The results showed the superiority of WGRMF compared with previous methods. Besides, based on the prediction results using the GDSC dataset, three types of case studies were carried out. The results from both cross-validation and case studies have shown the effectiveness of WGRMF on the prediction of drug response in cell lines.
Keywords: cell line; drug response; graph regularization; matrix factorization; response prediction.
Copyright © 2019 The Author(s). Published by Elsevier Inc. All rights reserved.
Figures
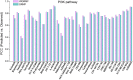
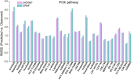
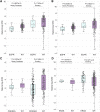
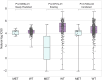
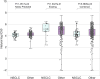
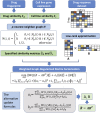
Similar articles
-
Improved anticancer drug response prediction in cell lines using matrix factorization with similarity regularization.BMC Cancer. 2017 Aug 2;17(1):513. doi: 10.1186/s12885-017-3500-5. BMC Cancer. 2017. PMID: 28768489 Free PMC article.
-
Predicting lncRNA-Protein Interaction With Weighted Graph-Regularized Matrix Factorization.Front Genet. 2021 Jul 16;12:690096. doi: 10.3389/fgene.2021.690096. eCollection 2021. Front Genet. 2021. PMID: 34335693 Free PMC article.
-
De novo Prediction of Cell-Drug Sensitivities Using Deep Learning-based Graph Regularized Matrix Factorization.Pac Symp Biocomput. 2022;27:278-289. Pac Symp Biocomput. 2022. PMID: 34890156 Free PMC article.
-
A weighted non-negative matrix factorization approach to predict potential associations between drug and disease.J Transl Med. 2022 Dec 3;20(1):552. doi: 10.1186/s12967-022-03757-1. J Transl Med. 2022. PMID: 36463215 Free PMC article.
-
Current Trends in Drug Sensitivity Prediction.Curr Pharm Des. 2016;22(46):6918-6927. doi: 10.2174/1381612822666161026154430. Curr Pharm Des. 2016. PMID: 27784247 Review.
Cited by
-
DRPreter: Interpretable Anticancer Drug Response Prediction Using Knowledge-Guided Graph Neural Networks and Transformer.Int J Mol Sci. 2022 Nov 11;23(22):13919. doi: 10.3390/ijms232213919. Int J Mol Sci. 2022. PMID: 36430395 Free PMC article.
-
An Innovative Multi-Omics Model Integrating Latent Alignment and Attention Mechanism for Drug Response Prediction.J Pers Med. 2024 Jun 27;14(7):694. doi: 10.3390/jpm14070694. J Pers Med. 2024. PMID: 39063948 Free PMC article.
-
Drug response prediction using graph representation learning and Laplacian feature selection.BMC Bioinformatics. 2022 Dec 9;23(Suppl 8):532. doi: 10.1186/s12859-022-05080-4. BMC Bioinformatics. 2022. PMID: 36494630 Free PMC article.
-
Computational drug repositioning using similarity constrained weight regularization matrix factorization: A case of COVID-19.J Cell Mol Med. 2022 Jul;26(13):3772-3782. doi: 10.1111/jcmm.17412. Epub 2022 May 29. J Cell Mol Med. 2022. PMID: 35644992 Free PMC article.
-
DBDNMF: A Dual Branch Deep Neural Matrix Factorization method for drug response prediction.PLoS Comput Biol. 2024 Apr 4;20(4):e1012012. doi: 10.1371/journal.pcbi.1012012. eCollection 2024 Apr. PLoS Comput Biol. 2024. PMID: 38574114 Free PMC article.
References
LinkOut - more resources
Full Text Sources