Genetic cooperativity in multi-layer networks implicates cell survival and senescence in the striatum of Huntington's disease mice synchronous to symptoms
- PMID: 31228193
- PMCID: PMC6956776
- DOI: 10.1093/bioinformatics/btz514
Genetic cooperativity in multi-layer networks implicates cell survival and senescence in the striatum of Huntington's disease mice synchronous to symptoms
Abstract
Motivation: Huntington's disease (HD) may evolve through gene deregulation. However, the impact of gene deregulation on the dynamics of genetic cooperativity in HD remains poorly understood. Here, we built a multi-layer network model of temporal dynamics of genetic cooperativity in the brain of HD knock-in mice (allelic series of Hdh mice). To enhance biological precision and gene prioritization, we integrated three complementary families of source networks, all inferred from the same RNA-seq time series data in Hdh mice, into weighted-edge networks where an edge recapitulates path-length variation across source-networks and age-points.
Results: Weighted edge networks identify two consecutive waves of tight genetic cooperativity enriched in deregulated genes (critical phases), pre-symptomatically in the cortex, implicating neurotransmission, and symptomatically in the striatum, implicating cell survival (e.g. Hipk4) intertwined with cell proliferation (e.g. Scn4b) and cellular senescence (e.g. Cdkn2a products) responses. Top striatal weighted edges are enriched in modulators of defective behavior in invertebrate models of HD pathogenesis, validating their relevance to neuronal dysfunction in vivo. Collectively, these findings reveal highly dynamic temporal features of genetic cooperativity in the brain of Hdh mice where a 2-step logic highlights the importance of cellular maintenance and senescence in the striatum of symptomatic mice, providing highly prioritized targets.
Availability and implementation: Weighted edge network analysis (WENA) data and source codes for performing spectral decomposition of the signal (SDS) and WENA analysis, both written using Python, are available at http://www.broca.inserm.fr/HD-WENA/.
Supplementary information: Supplementary data are available at Bioinformatics online.
© The Author(s) 2019. Published by Oxford University Press.
Figures
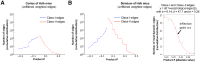
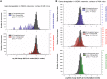
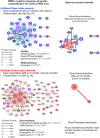
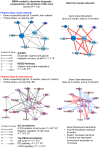
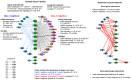
Similar articles
-
Deficits in coordinated neuronal activity and network topology are striatal hallmarks in Huntington's disease.BMC Biol. 2020 May 28;18(1):58. doi: 10.1186/s12915-020-00794-4. BMC Biol. 2020. PMID: 32466798 Free PMC article.
-
Systems Genetic Analyses Highlight a TGFβ-FOXO3 Dependent Striatal Astrocyte Network Conserved across Species and Associated with Stress, Sleep, and Huntington's Disease.PLoS Genet. 2016 Jul 8;12(7):e1006137. doi: 10.1371/journal.pgen.1006137. eCollection 2016 Jul. PLoS Genet. 2016. PMID: 27390852 Free PMC article.
-
The p75 neurotrophin receptor augments survival signaling in the striatum of pre-symptomatic Q175(WT/HD) mice.Neuroscience. 2016 Jun 2;324:297-306. doi: 10.1016/j.neuroscience.2016.02.069. Epub 2016 Mar 3. Neuroscience. 2016. PMID: 26947127 Free PMC article.
-
Ectopic expression of the striatal-enriched GTPase Rhes elicits cerebellar degeneration and an ataxia phenotype in Huntington's disease.Neurobiol Dis. 2015 Oct;82:66-77. doi: 10.1016/j.nbd.2015.05.011. Epub 2015 Jun 3. Neurobiol Dis. 2015. PMID: 26048156
-
Huntington's disease is a disorder of the corpus striatum: focus on Rhes (Ras homologue enriched in the striatum).Neuropharmacology. 2011 Jun;60(7-8):1187-92. doi: 10.1016/j.neuropharm.2010.10.025. Epub 2010 Oct 31. Neuropharmacology. 2011. PMID: 21044641 Review.
Cited by
-
DNA Damage Response-Associated Cell Cycle Re-Entry and Neuronal Senescence in Brain Aging and Alzheimer's Disease.J Alzheimers Dis. 2023;94(s1):S429-S451. doi: 10.3233/JAD-220203. J Alzheimers Dis. 2023. PMID: 35848025 Free PMC article. Review.
-
Biological Aging and the Cellular Pathogenesis of Huntington's Disease.J Huntingtons Dis. 2020;9(2):115-128. doi: 10.3233/JHD-200395. J Huntingtons Dis. 2020. PMID: 32417788 Free PMC article. Review.
-
How is Big Data reshaping preclinical aging research?Lab Anim (NY). 2023 Dec;52(12):289-314. doi: 10.1038/s41684-023-01286-y. Epub 2023 Nov 28. Lab Anim (NY). 2023. PMID: 38017182 Review.
-
Shape deformation analysis reveals the temporal dynamics of cell-type-specific homeostatic and pathogenic responses to mutant huntingtin.Elife. 2021 Feb 23;10:e64984. doi: 10.7554/eLife.64984. Elife. 2021. PMID: 33618800 Free PMC article.
-
Identification of key methylation differentially expressed genes in posterior fossa ependymoma based on epigenomic and transcriptome analysis.J Transl Med. 2021 Apr 26;19(1):174. doi: 10.1186/s12967-021-02834-1. J Transl Med. 2021. PMID: 33902636 Free PMC article.
References
-
- Achour M. et al. (2015) Neuronal identity genes regulated by super-enhancers are preferentially down-regulated in the striatum of Huntington’s disease mice. Hum. Mol. Genet., 24, 3481–3496. - PubMed
-
- Aguirre A. et al. (2007) A functional role for EGFR signaling in myelination and remyelination. Nat. Neurosci., 10, 990–1002. - PubMed
-
- Alexandrov V. et al. (2016) Large-scale phenome analysis defines a behavioral signature for Huntington’s disease genotype in mice. Nat. Biotechnol., 34, 838–844. - PubMed
Publication types
MeSH terms
LinkOut - more resources
Full Text Sources
Medical
Miscellaneous