The ability of transcription factors to differentially regulate gene expression is a crucial component of the mechanism underlying inversion, a frequently observed genetic interaction pattern
- PMID: 31083661
- PMCID: PMC6532943
- DOI: 10.1371/journal.pcbi.1007061
The ability of transcription factors to differentially regulate gene expression is a crucial component of the mechanism underlying inversion, a frequently observed genetic interaction pattern
Abstract
Genetic interactions, a phenomenon whereby combinations of mutations lead to unexpected effects, reflect how cellular processes are wired and play an important role in complex genetic diseases. Understanding the molecular basis of genetic interactions is crucial for deciphering pathway organization as well as understanding the relationship between genetic variation and disease. Several hypothetical molecular mechanisms have been linked to different genetic interaction types. However, differences in genetic interaction patterns and their underlying mechanisms have not yet been compared systematically between different functional gene classes. Here, differences in the occurrence and types of genetic interactions are compared for two classes, gene-specific transcription factors (GSTFs) and signaling genes (kinases and phosphatases). Genome-wide gene expression data for 63 single and double deletion mutants in baker's yeast reveals that the two most common genetic interaction patterns are buffering and inversion. Buffering is typically associated with redundancy and is well understood. In inversion, genes show opposite behavior in the double mutant compared to the corresponding single mutants. The underlying mechanism is poorly understood. Although both classes show buffering and inversion patterns, the prevalence of inversion is much stronger in GSTFs. To decipher potential mechanisms, a Petri Net modeling approach was employed, where genes are represented as nodes and relationships between genes as edges. This allowed over 9 million possible three and four node models to be exhaustively enumerated. The models show that a quantitative difference in interaction strength is a strict requirement for obtaining inversion. In addition, this difference is frequently accompanied with a second gene that shows buffering. Taken together, these results provide a mechanistic explanation for inversion. Furthermore, the ability of transcription factors to differentially regulate expression of their targets provides a likely explanation why inversion is more prevalent for GSTFs compared to kinases and phosphatases.
Conflict of interest statement
The authors have declared that no competing interests exist.
Figures
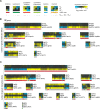
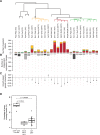
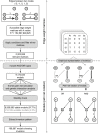
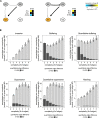
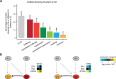
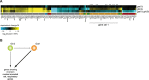
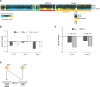
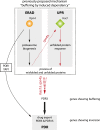
Similar articles
-
A high-resolution gene expression atlas of epistasis between gene-specific transcription factors exposes potential mechanisms for genetic interactions.BMC Biol. 2015 Dec 23;13:112. doi: 10.1186/s12915-015-0222-5. BMC Biol. 2015. PMID: 26700642 Free PMC article.
-
Recursive expectation-maximization clustering: a method for identifying buffering mechanisms composed of phenomic modules.Chaos. 2010 Jun;20(2):026103. doi: 10.1063/1.3455188. Chaos. 2010. PMID: 20590332 Free PMC article.
-
Prediction of phenotype and gene expression for combinations of mutations.Mol Syst Biol. 2007;3:96. doi: 10.1038/msb4100137. Epub 2007 Mar 27. Mol Syst Biol. 2007. PMID: 17389876 Free PMC article.
-
Exploring genetic interactions and networks with yeast.Nat Rev Genet. 2007 Jun;8(6):437-49. doi: 10.1038/nrg2085. Nat Rev Genet. 2007. PMID: 17510664 Review.
-
Gene-gene and gene-environment interactions in complex traits in yeast.Yeast. 2018 Jun;35(6):403-416. doi: 10.1002/yea.3304. Epub 2018 Feb 22. Yeast. 2018. PMID: 29322552 Review.
Cited by
-
The PAC-3 transcription factor critically regulates phenotype-associated genes in Neurospora crassa.Genet Mol Biol. 2020 Jun 22;43(3):e20190374. doi: 10.1590/1678-4685-GMB-2019-0374. eCollection 2020. Genet Mol Biol. 2020. PMID: 32584919 Free PMC article.
-
Global Molecular Response of Paracoccidioides brasiliensis to Zinc Deprivation: Analyses at Transcript, Protein and MicroRNA Levels.J Fungi (Basel). 2023 Feb 21;9(3):281. doi: 10.3390/jof9030281. J Fungi (Basel). 2023. PMID: 36983449 Free PMC article.
-
Random epigenetic modulation of CHO cells by repeated knockdown of DNA methyltransferases increases population diversity and enables sorting of cells with higher production capacities.Biotechnol Bioeng. 2020 Nov;117(11):3435-3447. doi: 10.1002/bit.27493. Epub 2020 Jul 24. Biotechnol Bioeng. 2020. PMID: 32662873 Free PMC article.
References
Publication types
MeSH terms
Substances
Grants and funding
LinkOut - more resources
Full Text Sources
Molecular Biology Databases