Determining cell type abundance and expression from bulk tissues with digital cytometry
- PMID: 31061481
- PMCID: PMC6610714
- DOI: 10.1038/s41587-019-0114-2
Determining cell type abundance and expression from bulk tissues with digital cytometry
Abstract
Single-cell RNA-sequencing has emerged as a powerful technique for characterizing cellular heterogeneity, but it is currently impractical on large sample cohorts and cannot be applied to fixed specimens collected as part of routine clinical care. We previously developed an approach for digital cytometry, called CIBERSORT, that enables estimation of cell type abundances from bulk tissue transcriptomes. We now introduce CIBERSORTx, a machine learning method that extends this framework to infer cell-type-specific gene expression profiles without physical cell isolation. By minimizing platform-specific variation, CIBERSORTx also allows the use of single-cell RNA-sequencing data for large-scale tissue dissection. We evaluated the utility of CIBERSORTx in multiple tumor types, including melanoma, where single-cell reference profiles were used to dissect bulk clinical specimens, revealing cell-type-specific phenotypic states linked to distinct driver mutations and response to immune checkpoint blockade. We anticipate that digital cytometry will augment single-cell profiling efforts, enabling cost-effective, high-throughput tissue characterization without the need for antibodies, disaggregation or viable cells.
Conflict of interest statement
Competing Interests
A.M.N. has patent filings related to expression deconvolution and cancer biomarkers and has served as a consultant for Roche, Merck, and CiberMed. A.A.A. has patent filings related to expression deconvolution and cancer biomarkers and has served as a consultant or advisor for Roche, Genentech, Janssen, CiberMed, Pharmacyclics, Gilead, and Celgene. M.D. has patent filings related to cancer biomarkers and has served as a consultant for Roche, Novartis, CiberMed, and Quanticel Pharmaceuticals. No potential conflicts of interest were disclosed by the other authors.
Figures
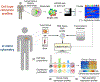
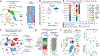
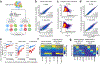
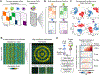
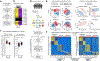
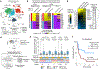
Comment in
-
Expanded CIBERSORTx.Nat Methods. 2019 Jul;16(7):577. doi: 10.1038/s41592-019-0486-8. Nat Methods. 2019. PMID: 31249418 No abstract available.
Similar articles
-
Profiling Cell Type Abundance and Expression in Bulk Tissues with CIBERSORTx.Methods Mol Biol. 2020;2117:135-157. doi: 10.1007/978-1-0716-0301-7_7. Methods Mol Biol. 2020. PMID: 31960376 Free PMC article.
-
Deconvolution analysis of cell-type expression from bulk tissues by integrating with single-cell expression reference.Genet Epidemiol. 2022 Dec;46(8):615-628. doi: 10.1002/gepi.22494. Epub 2022 Jul 5. Genet Epidemiol. 2022. PMID: 35788983 Free PMC article.
-
Approximate estimation of cell-type resolution transcriptome in bulk tissue through matrix completion.Brief Bioinform. 2023 Sep 20;24(5):bbad273. doi: 10.1093/bib/bbad273. Brief Bioinform. 2023. PMID: 37529921
-
A review of digital cytometry methods: estimating the relative abundance of cell types in a bulk of cells.Brief Bioinform. 2021 Jul 20;22(4):bbaa219. doi: 10.1093/bib/bbaa219. Brief Bioinform. 2021. PMID: 33003193 Free PMC article. Review.
-
Machine learning and statistical methods for clustering single-cell RNA-sequencing data.Brief Bioinform. 2020 Jul 15;21(4):1209-1223. doi: 10.1093/bib/bbz063. Brief Bioinform. 2020. PMID: 31243426 Review.
Cited by
-
Network Analysis of Dysregulated Immune Response to COVID-19 mRNA Vaccination in Hemodialysis Patients.Vaccines (Basel). 2024 Oct 7;12(10):1146. doi: 10.3390/vaccines12101146. Vaccines (Basel). 2024. PMID: 39460313 Free PMC article.
-
Ulcerative colitis immune cell landscapes and differentially expressed gene signatures determine novel regulators and predict clinical response to biologic therapy.Sci Rep. 2021 Apr 27;11(1):9010. doi: 10.1038/s41598-021-88489-w. Sci Rep. 2021. PMID: 33907256 Free PMC article.
-
Micro-biopsy for detection of gene expression changes in ischemic swine myocardium: A pilot study.PLoS One. 2021 Apr 28;16(4):e0250582. doi: 10.1371/journal.pone.0250582. eCollection 2021. PLoS One. 2021. PMID: 33909677 Free PMC article.
-
Deep learning-based cell composition analysis from tissue expression profiles.Sci Adv. 2020 Jul 22;6(30):eaba2619. doi: 10.1126/sciadv.aba2619. eCollection 2020 Jul. Sci Adv. 2020. PMID: 32832661 Free PMC article.
-
MuSiC2: cell-type deconvolution for multi-condition bulk RNA-seq data.Brief Bioinform. 2022 Nov 19;23(6):bbac430. doi: 10.1093/bib/bbac430. Brief Bioinform. 2022. PMID: 36208175 Free PMC article.
References
Publication types
MeSH terms
Substances
Grants and funding
LinkOut - more resources
Full Text Sources
Other Literature Sources
Molecular Biology Databases