In silico learning of tumor evolution through mutational time series
- PMID: 31015295
- PMCID: PMC6510994
- DOI: 10.1073/pnas.1901695116
In silico learning of tumor evolution through mutational time series
Abstract
Cancer arises through the accumulation of somatic mutations over time. Understanding the sequence of mutation occurrence during cancer progression can assist early and accurate diagnosis and improve clinical decision-making. Here we employ long short-term memory (LSTM) networks, a class of recurrent neural network, to learn the evolution of a tumor through an ordered sequence of mutations. We demonstrate the capacity of LSTMs to learn complex dynamics of the mutational time series governing tumor progression, allowing accurate prediction of the mutational burden and the occurrence of mutations in the sequence. Using the probabilities learned by the LSTM, we simulate mutational data and show that the simulation results are statistically indistinguishable from the empirical data. We identify passenger mutations that are significantly associated with established cancer drivers in the sequence and demonstrate that the genes carrying these mutations are substantially enriched in interactions with the corresponding driver genes. Breaking the network into modules consisting of driver genes and their interactors, we show that these interactions are associated with poor patient prognosis, thus likely conferring growth advantage for tumor progression. Thus, application of LSTM provides for prediction of numerous additional conditional drivers and reveals hitherto unknown aspects of cancer evolution.
Keywords: cancer progression; driver mutations; machine learning; neural networks; passenger mutations.
Copyright © 2019 the Author(s). Published by PNAS.
Conflict of interest statement
The authors declare no conflict of interest.
Figures
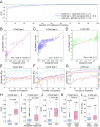
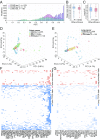
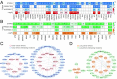
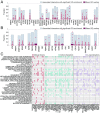
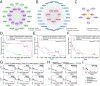
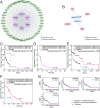
Similar articles
-
Machine Learning Classification and Structure-Functional Analysis of Cancer Mutations Reveal Unique Dynamic and Network Signatures of Driver Sites in Oncogenes and Tumor Suppressor Genes.J Chem Inf Model. 2018 Oct 22;58(10):2131-2150. doi: 10.1021/acs.jcim.8b00414. Epub 2018 Oct 3. J Chem Inf Model. 2018. PMID: 30253099
-
A new LSTM-based gene expression prediction model: L-GEPM.J Bioinform Comput Biol. 2019 Aug;17(4):1950022. doi: 10.1142/S0219720019500227. J Bioinform Comput Biol. 2019. PMID: 31617459
-
Deep convolutional neural networks for accurate somatic mutation detection.Nat Commun. 2019 Mar 4;10(1):1041. doi: 10.1038/s41467-019-09027-x. Nat Commun. 2019. PMID: 30833567 Free PMC article.
-
Identifying driver mutations from sequencing data of heterogeneous tumors in the era of personalized genome sequencing.Brief Bioinform. 2014 Mar;15(2):244-55. doi: 10.1093/bib/bbt042. Epub 2013 Jul 1. Brief Bioinform. 2014. PMID: 23818492 Review.
-
Whole-exome sequencing reveals recurrent somatic mutation networks in cancer.Cancer Lett. 2013 Nov 1;340(2):270-6. doi: 10.1016/j.canlet.2012.11.002. Epub 2012 Nov 12. Cancer Lett. 2013. PMID: 23153794 Review.
Cited by
-
Autoformer-Based Model for Predicting and Assessing Wheat Quality Changes of Pesticide Residues during Storage.Foods. 2023 Apr 28;12(9):1833. doi: 10.3390/foods12091833. Foods. 2023. PMID: 37174371 Free PMC article.
-
Computational Methods Summarizing Mutational Patterns in Cancer: Promise and Limitations for Clinical Applications.Cancers (Basel). 2023 Mar 24;15(7):1958. doi: 10.3390/cancers15071958. Cancers (Basel). 2023. PMID: 37046619 Free PMC article. Review.
-
Ectopic expression of meiotic cohesin generates chromosome instability in cancer cell line.Proc Natl Acad Sci U S A. 2022 Oct 4;119(40):e2204071119. doi: 10.1073/pnas.2204071119. Epub 2022 Sep 30. Proc Natl Acad Sci U S A. 2022. PMID: 36179046 Free PMC article.
-
Over a century of cancer research: Inconvenient truths and promising leads.PLoS Biol. 2020 Apr 1;18(4):e3000670. doi: 10.1371/journal.pbio.3000670. eCollection 2020 Apr. PLoS Biol. 2020. PMID: 32236102 Free PMC article.
-
DEGBOE: Discrete time Evolution modeling of Gene mutation through Bayesian inference using qualitative Observation of mutation Events.J Biomed Inform. 2022 Oct;134:104197. doi: 10.1016/j.jbi.2022.104197. Epub 2022 Sep 6. J Biomed Inform. 2022. PMID: 36084801 Free PMC article.
References
Publication types
MeSH terms
LinkOut - more resources
Full Text Sources
Other Literature Sources