A Bayesian model of acquisition and clearance of bacterial colonization incorporating within-host variation
- PMID: 31009452
- PMCID: PMC6497309
- DOI: 10.1371/journal.pcbi.1006534
A Bayesian model of acquisition and clearance of bacterial colonization incorporating within-host variation
Abstract
Bacterial populations that colonize a host can play important roles in host health, including serving as a reservoir that transmits to other hosts and from which invasive strains emerge, thus emphasizing the importance of understanding rates of acquisition and clearance of colonizing populations. Studies of colonization dynamics have been based on assessment of whether serial samples represent a single population or distinct colonization events. With the use of whole genome sequencing to determine genetic distance between isolates, a common solution to estimate acquisition and clearance rates has been to assume a fixed genetic distance threshold below which isolates are considered to represent the same strain. However, this approach is often inadequate to account for the diversity of the underlying within-host evolving population, the time intervals between consecutive measurements, and the uncertainty in the estimated acquisition and clearance rates. Here, we present a fully Bayesian model that provides probabilities of whether two strains should be considered the same, allowing us to determine bacterial clearance and acquisition from genomes sampled over time. Our method explicitly models the within-host variation using population genetic simulation, and the inference is done using a combination of Approximate Bayesian Computation (ABC) and Markov Chain Monte Carlo (MCMC). We validate the method with multiple carefully conducted simulations and demonstrate its use in practice by analyzing a collection of methicillin resistant Staphylococcus aureus (MRSA) isolates from a large recently completed longitudinal clinical study. An R-code implementation of the method is freely available at: https://github.com/mjarvenpaa/bacterial-colonization-model.
Conflict of interest statement
The authors have declared that no relevant competing interests exist.
Figures
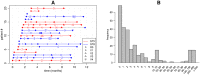
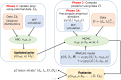
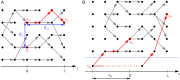
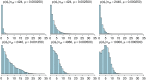
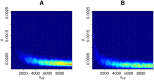
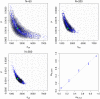
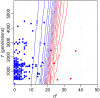
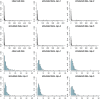
Similar articles
-
Natural history of colonization with methicillin-resistant Staphylococcus aureus (MRSA) and vancomycin-resistant Enterococcus (VRE): a systematic review.BMC Infect Dis. 2014 Mar 31;14:177. doi: 10.1186/1471-2334-14-177. BMC Infect Dis. 2014. PMID: 24678646 Free PMC article. Review.
-
Longitudinal evaluation of clinical and colonization methicillin-resistant Staphylococcus aureus isolates among veterans.Infect Control Hosp Epidemiol. 2015 May;36(5):587-9. doi: 10.1017/ice.2015.14. Epub 2015 Feb 5. Infect Control Hosp Epidemiol. 2015. PMID: 25652133
-
Experimental colonization of pigs with methicillin-resistant Staphylococcus aureus (MRSA): insights into the colonization and transmission of livestock-associated MRSA.Epidemiol Infect. 2011 Oct;139(10):1594-600. doi: 10.1017/S0950268810002888. Epub 2010 Dec 15. Epidemiol Infect. 2011. PMID: 21156101
-
Longitudinal genetic analyses of Staphylococcus aureus nasal carriage dynamics in a diverse population.BMC Infect Dis. 2013 May 16;13:221. doi: 10.1186/1471-2334-13-221. BMC Infect Dis. 2013. PMID: 23679038 Free PMC article.
-
Host-Bacterial Crosstalk Determines Staphylococcus aureus Nasal Colonization.Trends Microbiol. 2016 Nov;24(11):872-886. doi: 10.1016/j.tim.2016.06.012. Epub 2016 Jul 26. Trends Microbiol. 2016. PMID: 27474529 Review.
Cited by
-
Bayesian modeling of the impact of antibiotic resistance on the efficiency of MRSA decolonization.PLoS Comput Biol. 2023 Oct 26;19(10):e1010898. doi: 10.1371/journal.pcbi.1010898. eCollection 2023 Oct. PLoS Comput Biol. 2023. PMID: 37883601 Free PMC article.
-
Genomic Analysis of Community Transmission Networks for MRSA Among Females Entering a Large Inner-city Jail.Open Forum Infect Dis. 2022 Jan 31;9(3):ofac049. doi: 10.1093/ofid/ofac049. eCollection 2022 Mar. Open Forum Infect Dis. 2022. PMID: 35211635 Free PMC article.
-
On predictive inference for intractable models via approximate Bayesian computation.Stat Comput. 2023;33(2):42. doi: 10.1007/s11222-022-10163-6. Epub 2023 Feb 9. Stat Comput. 2023. PMID: 36785730 Free PMC article.
-
Model-based inference of a plant-specific dual role for HOPS in regulating guard cell vacuole fusion.bioRxiv [Preprint]. 2023 Nov 9:2023.11.07.565947. doi: 10.1101/2023.11.07.565947. bioRxiv. 2023. Update in: In Silico Plants. 2024 Aug 30;6(2):diae015. doi: 10.1093/insilicoplants/diae015. PMID: 37986942 Free PMC article. Updated. Preprint.
-
Genomic Epidemiology Suggests Community Origins of Healthcare-Associated USA300 Methicillin-Resistant Staphylococcus aureus.J Infect Dis. 2022 Aug 12;226(1):157-166. doi: 10.1093/infdis/jiac056. J Infect Dis. 2022. PMID: 35172338 Free PMC article.