Associations between High Temperature, Heavy Rainfall, and Diarrhea among Young Children in Rural Tamil Nadu, India: A Prospective Cohort Study
- PMID: 30986088
- PMCID: PMC6785227
- DOI: 10.1289/EHP3711
Associations between High Temperature, Heavy Rainfall, and Diarrhea among Young Children in Rural Tamil Nadu, India: A Prospective Cohort Study
Abstract
Background: The effects of weather on diarrhea could influence the health impacts of climate change. Children have the highest diarrhea incidence, especially in India, where many lack safe water and sanitation.
Objectives: In a prospective cohort of 1,284 children under 5 y of age from 900 households across 25 villages in rural Tamil Nadu, India, we examined whether high temperature and heavy rainfall was associated with increased all-cause diarrhea and water contamination.
Methods: Seven-day prevalence of diarrhea was assessed monthly for up to 12 visits from January 2008 to April 2009, and hydrogen sulfide ([Formula: see text]) presence in drinking water, a fecal contamination indicator, was tested in a subset of households. We estimated associations between temperature and rainfall exposures and diarrhea and [Formula: see text] using binomial regressions, adjusting for potential confounders, random effects for village, and autoregressive-1 error terms for study week.
Results: There were 259 cases of diarrhea. The prevalence of diarrhea during the 7 d before visits was 2.95 times higher (95% CI: 1.99, 4.39) when mean temperature in the week before the 7-d recall was in the hottest versus the coolest quartile of weekly mean temperature during 1 December 2007 to 15 April 2009. Diarrhea prevalence was 1.50 times higher when the 3 weeks before the diarrhea recall period included [Formula: see text] (vs. 0 d) with rainfall of [Formula: see text] (95% CI: 1.12, 2.02), and 2.60 times higher (95% CI: 1.55, 4.36) for heavy rain weeks following a 60-d dry period. The [Formula: see text] prevalence in household water was not associated with heavy rain prior to sample collection.
Conclusions: The results suggest that, in rural Tamil Nadu, heavy rainfall may wash pathogens that accumulate during dry weather into child contact. Higher temperatures were positively associated with diarrhea 1-3 weeks later. Our findings suggest that diarrhea morbidity could worsen under climate change without interventions to reduce enteric pathogen transmission through multiple pathways. https://doi.org/10.1289/EHP3711.
Figures
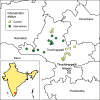
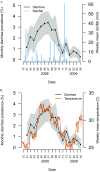
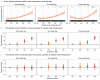
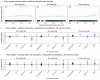
Comment in
-
Before the Storm: A Potential Role for Dry Weather in Children's Risk of Diarrhea.Environ Health Perspect. 2019 Dec;127(12):124003. doi: 10.1289/EHP6135. Epub 2019 Dec 24. Environ Health Perspect. 2019. PMID: 31873043 Free PMC article.
Similar articles
-
Heavy rainfall events and diarrhea incidence: the role of social and environmental factors.Am J Epidemiol. 2014 Feb 1;179(3):344-52. doi: 10.1093/aje/kwt279. Epub 2013 Nov 19. Am J Epidemiol. 2014. PMID: 24256618 Free PMC article.
-
Influence of Temperature and Precipitation on the Effectiveness of Water, Sanitation, and Handwashing Interventions against Childhood Diarrheal Disease in Rural Bangladesh: A Reanalysis of the WASH Benefits Bangladesh Trial.Environ Health Perspect. 2024 Apr;132(4):47006. doi: 10.1289/EHP13807. Epub 2024 Apr 11. Environ Health Perspect. 2024. PMID: 38602833 Free PMC article. Clinical Trial.
-
The effect of India's total sanitation campaign on defecation behaviors and child health in rural Madhya Pradesh: a cluster randomized controlled trial.PLoS Med. 2014 Aug 26;11(8):e1001709. doi: 10.1371/journal.pmed.1001709. eCollection 2014 Aug. PLoS Med. 2014. PMID: 25157929 Free PMC article. Clinical Trial.
-
Effect of household-based drinking water chlorination on diarrhoea among children under five in Orissa, India: a double-blind randomised placebo-controlled trial.PLoS Med. 2013 Aug;10(8):e1001497. doi: 10.1371/journal.pmed.1001497. Epub 2013 Aug 20. PLoS Med. 2013. PMID: 23976883 Free PMC article. Clinical Trial.
-
Folic acid supplementation and malaria susceptibility and severity among people taking antifolate antimalarial drugs in endemic areas.Cochrane Database Syst Rev. 2022 Feb 1;2(2022):CD014217. doi: 10.1002/14651858.CD014217. Cochrane Database Syst Rev. 2022. PMID: 36321557 Free PMC article.
Cited by
-
Systems Science Approaches for Global Environmental Health Research: Enhancing Intervention Design and Implementation for Household Air Pollution (HAP) and Water, Sanitation, and Hygiene (WASH) Programs.Environ Health Perspect. 2020 Oct;128(10):105001. doi: 10.1289/EHP7010. Epub 2020 Oct 9. Environ Health Perspect. 2020. PMID: 33035121 Free PMC article.
-
Before the Storm: A Potential Role for Dry Weather in Children's Risk of Diarrhea.Environ Health Perspect. 2019 Dec;127(12):124003. doi: 10.1289/EHP6135. Epub 2019 Dec 24. Environ Health Perspect. 2019. PMID: 31873043 Free PMC article.
-
The Association between Anthropometric Failure and Toilet Types: A Cross-Sectional Study from India.Am J Trop Med Hyg. 2023 Feb 13;108(4):811-819. doi: 10.4269/ajtmh.22-0138. Print 2023 Apr 5. Am J Trop Med Hyg. 2023. PMID: 36780894 Free PMC article.
-
Poultry Ownership Associated with Increased Risk of Child Diarrhea: Cross-Sectional Evidence from Uganda.Am J Trop Med Hyg. 2020 Mar;102(3):526-533. doi: 10.4269/ajtmh.19-0012. Am J Trop Med Hyg. 2020. PMID: 31971130 Free PMC article.
-
Effects of High Temperature and Heavy Precipitation on Drinking Water Quality and Child Hand Contamination Levels in Rural Kenya.Environ Sci Technol. 2023 May 2;57(17):6975-6988. doi: 10.1021/acs.est.2c07284. Epub 2023 Apr 18. Environ Sci Technol. 2023. PMID: 37071701 Free PMC article.
References
Publication types
MeSH terms
LinkOut - more resources
Full Text Sources
Medical
Miscellaneous