Complex Trait Prediction from Genome Data: Contrasting EBV in Livestock to PRS in Humans: Genomic Prediction
- PMID: 30967442
- PMCID: PMC6456317
- DOI: 10.1534/genetics.119.301859
Complex Trait Prediction from Genome Data: Contrasting EBV in Livestock to PRS in Humans: Genomic Prediction
Abstract
In this Review, we focus on the similarity of the concepts underlying prediction of estimated breeding values (EBVs) in livestock and polygenic risk scores (PRS) in humans. Our research spans both fields and so we recognize factors that are very obvious for those in one field, but less so for those in the other. Differences in family size between species is the wedge that drives the different viewpoints and approaches. Large family size achievable in nonhuman species accompanied by selection generates a smaller effective population size, increased linkage disequilibrium and a higher average genetic relationship between individuals within a population. In human genetic analyses, we select individuals unrelated in the classical sense (coefficient of relationship <0.05) to estimate heritability captured by common SNPs. In livestock data, all animals within a breed are to some extent "related," and so it is not possible to select unrelated individuals and retain a data set of sufficient size to analyze. These differences directly or indirectly impact the way data analyses are undertaken. In livestock, genetic segregation variance exposed through samplings of parental genomes within families is directly observable and taken for granted. In humans, this genomic variation is under-recognized for its contribution to variation in polygenic risk of common disease, in both those with and without family history of disease. We explore the equation that predicts the expected proportion of variance explained using PRS, and quantify how GWAS sample size is the key factor for maximizing accuracy of prediction in both humans and livestock. Last, we bring together the concepts discussed to address some frequently asked questions.
Keywords: EBV; GenPred; Genomic Prediction; PRS; estimated breeding values; polygenic risk score; segregation variance; within family variance.
Copyright © 2019 by the Genetics Society of America.
Figures
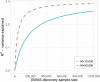
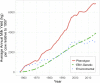
Similar articles
-
Prediction of genomic breeding values based on pre-selected SNPs using ssGBLUP, WssGBLUP and BayesB for Edwardsiellosis resistance in Japanese flounder.Genet Sel Evol. 2020 Aug 18;52(1):49. doi: 10.1186/s12711-020-00566-2. Genet Sel Evol. 2020. PMID: 32811444 Free PMC article.
-
The importance of information on relatives for the prediction of genomic breeding values and the implications for the makeup of reference data sets in livestock breeding schemes.Genet Sel Evol. 2012 Feb 9;44(1):4. doi: 10.1186/1297-9686-44-4. Genet Sel Evol. 2012. PMID: 22321529 Free PMC article.
-
Model Comparison of Heritability Enrichment Analysis in Livestock Population.Genes (Basel). 2022 Sep 13;13(9):1644. doi: 10.3390/genes13091644. Genes (Basel). 2022. PMID: 36140810 Free PMC article.
-
Polygenic Risk Score in African populations: progress and challenges.F1000Res. 2023 Apr 11;11:175. doi: 10.12688/f1000research.76218.2. eCollection 2022. F1000Res. 2023. PMID: 37273966 Free PMC article. Review.
-
Methods for Polygenic Traits.Methods Mol Biol. 2018;1793:145-156. doi: 10.1007/978-1-4939-7868-7_10. Methods Mol Biol. 2018. PMID: 29876896 Review.
Cited by
-
A Bayesian model for genomic prediction using metabolic networks.Bioinform Adv. 2023 Aug 11;3(1):vbad106. doi: 10.1093/bioadv/vbad106. eCollection 2023. Bioinform Adv. 2023. PMID: 39131740 Free PMC article.
-
Polygenic Indices (a.k.a. Polygenic Scores) in Social Science: A Guide for Interpretation and Evaluation.Sociol Methodol. 2024 Aug;54(2):300-350. doi: 10.1177/00811750241236482. Epub 2024 Mar 21. Sociol Methodol. 2024. PMID: 39091537 Free PMC article.
-
Screening embryos for polygenic disease risk: a review of epidemiological, clinical, and ethical considerations.Hum Reprod Update. 2024 Oct 1;30(5):529-557. doi: 10.1093/humupd/dmae012. Hum Reprod Update. 2024. PMID: 38805697 Free PMC article. Review.
-
The genetics of neuroticism: Insights from the Maudsley rat model and human studies.Personal Neurosci. 2023 Aug 4;6:e6. doi: 10.1017/pen.2023.4. eCollection 2023. Personal Neurosci. 2023. PMID: 38107782 Free PMC article. Review.
-
Editorial: Clinical implementation of genetic scores in cardiovascular medicine.Front Cardiovasc Med. 2023 Nov 24;10:1292116. doi: 10.3389/fcvm.2023.1292116. eCollection 2023. Front Cardiovasc Med. 2023. PMID: 38075967 Free PMC article. No abstract available.
References
-
- Anon2019. Chickenomics: how chicken became the rich world’s most popular meat. Economist Print edition, January 19, 2019.
-
- Beaumont R. N., Warrington N. M., Cavadino A., Tyrrell J., Nodzenski M., et al. , 2018. Genome-wide association study of offspring birth weight in 86 577 women identifies five novel loci and highlights maternal genetic effects that are independent of fetal genetics. Hum. Mol. Genet. 27: 742–756. 10.1093/hmg/ddx429 - DOI - PMC - PubMed
Publication types
MeSH terms
LinkOut - more resources
Full Text Sources