Cell Signaling Pathways in Mammary Carcinoma Induced in Rats with Low versus High Inherent Aerobic Capacity
- PMID: 30917509
- PMCID: PMC6470785
- DOI: 10.3390/ijms20061506
Cell Signaling Pathways in Mammary Carcinoma Induced in Rats with Low versus High Inherent Aerobic Capacity
Abstract
An inverse association exists between physical activity and breast cancer incidence and outcomes. An objective indicator of an individual's recent physical activity exposure is aerobic capacity. We took advantage of the fact that there is an inherited as well as inducible component of aerobic capacity to show that experimentally induced mammary cancer is inversely related to inherent aerobic capacity (IAC). The objective of this study was to determine whether cell signaling pathways involved in the development of mammary cancer differed in rats with low inherent aerobic capacity (LIAC, n = 55) versus high inherent aerobic capacity (HIAC, n = 57). Cancer burden was 0.21 ± 0.16 g/rat in HIAC versus 1.14 ± 0.45 in LIAC, p < 0.001. Based on protein expression, cancer in LIAC animals was associated with upregulated glucose utilization, and protein and fatty acid synthesis. Signaling in cancers from HIAC rats was associated with energy sensing, fatty acid oxidation and cell cycle arrest. These findings support the thesis that pro-glycolytic, metabolic inflexibility in LIAC favors not only insulin resistance and obesity but also tumor development and growth. This provides an unappreciated framework for understanding how obesity and low aerobic fitness, hallmarks of physical inactivity, are associated with higher cancer risk and poorer prognosis.
Keywords: cell signaling; inherent aerobic capacity; mammary cancer.
Conflict of interest statement
The authors declare no conflict of interest.
Figures
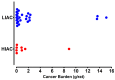
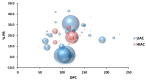
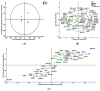
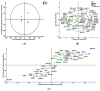
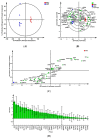
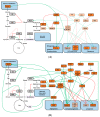
Similar articles
-
Inherent aerobic capacity-dependent differences in breast carcinogenesis.Carcinogenesis. 2017 Sep 1;38(9):920-928. doi: 10.1093/carcin/bgx066. Carcinogenesis. 2017. PMID: 28911004 Free PMC article.
-
Wheel running, skeletal muscle aerobic capacity and 1-methyl-1-nitrosourea induced mammary carcinogenesis in the rat.Carcinogenesis. 2010 Jul;31(7):1279-83. doi: 10.1093/carcin/bgq063. Epub 2010 Mar 18. Carcinogenesis. 2010. PMID: 20299525 Free PMC article.
-
Elevated expression of DecR1 impairs ErbB2/Neu-induced mammary tumor development.Mol Cell Biol. 2007 Sep;27(18):6361-71. doi: 10.1128/MCB.00686-07. Epub 2007 Jul 16. Mol Cell Biol. 2007. PMID: 17636013 Free PMC article.
-
Tumor microenvironment and metabolic synergy in breast cancers: critical importance of mitochondrial fuels and function.Semin Oncol. 2014 Apr;41(2):195-216. doi: 10.1053/j.seminoncol.2014.03.002. Epub 2014 Mar 5. Semin Oncol. 2014. PMID: 24787293 Review.
-
Magnetic fields and mammary cancer in rodents: a critical review and evaluation of published literature.Radiat Res. 2000 May;153(5 Pt 2):617-26. doi: 10.1667/0033-7587(2000)153[0617:mfamci]2.0.co;2. Radiat Res. 2000. PMID: 10790284 Review.
References
-
- Brenner D.R., Brockton N.T., Kotsopoulos J., Cotterchio M., Boucher B.A., Courneya K.S., Knight J.A., Olivotto I.A., Quan M.L., Friedenreich C.M. Breast cancer survival among young women: A review of the role of modifiable lifestyle factors. Cancer Causes Control. 2016;27:459–472. doi: 10.1007/s10552-016-0726-5. - DOI - PMC - PubMed
-
- Friedenreich C.M. Physical activity and breast cancer: Review of the epidemiologic evidence and biologic mechanisms. Recent Results Cancer Res. 2011;188:125–139. - PubMed
MeSH terms
Substances
Grants and funding
LinkOut - more resources
Full Text Sources