Unravelling Intratumoral Heterogeneity through High-Sensitivity Single-Cell Mutational Analysis and Parallel RNA Sequencing
- PMID: 30765193
- PMCID: PMC6436961
- DOI: 10.1016/j.molcel.2019.01.009
Unravelling Intratumoral Heterogeneity through High-Sensitivity Single-Cell Mutational Analysis and Parallel RNA Sequencing
Abstract
Single-cell RNA sequencing (scRNA-seq) has emerged as a powerful tool for resolving transcriptional heterogeneity. However, its application to studying cancerous tissues is currently hampered by the lack of coverage across key mutation hotspots in the vast majority of cells; this lack of coverage prevents the correlation of genetic and transcriptional readouts from the same single cell. To overcome this, we developed TARGET-seq, a method for the high-sensitivity detection of multiple mutations within single cells from both genomic and coding DNA, in parallel with unbiased whole-transcriptome analysis. Applying TARGET-seq to 4,559 single cells, we demonstrate how this technique uniquely resolves transcriptional and genetic tumor heterogeneity in myeloproliferative neoplasms (MPN) stem and progenitor cells, providing insights into deregulated pathways of mutant and non-mutant cells. TARGET-seq is a powerful tool for resolving the molecular signatures of genetically distinct subclones of cancer cells.
Keywords: Heterogeneity; TARGET-seq; cancer; hematopoiesis; leukemia; mutations; myeloproliferative neoplasm; sequencing; single-cell; transcriptomics.
Copyright © 2019 The Author(s). Published by Elsevier Inc. All rights reserved.
Figures
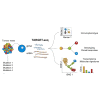
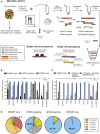
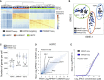
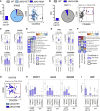
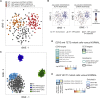
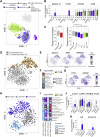
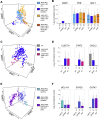
Comment in
-
TARGET-seq Takes Aim at Cancer Evolution through Multi-omics Single-Cell Genotyping and Transcriptomics.Mol Cell. 2019 Mar 21;73(6):1092-1094. doi: 10.1016/j.molcel.2019.03.009. Mol Cell. 2019. PMID: 30901562
Similar articles
-
TARGET-Seq: A Protocol for High-Sensitivity Single-Cell Mutational Analysis and Parallel RNA Sequencing.STAR Protoc. 2020 Oct 21;1(3):100125. doi: 10.1016/j.xpro.2020.100125. eCollection 2020 Dec 18. STAR Protoc. 2020. PMID: 33377019 Free PMC article.
-
Ultra-Sensitive Mutation Detection and Genome-Wide DNA Copy Number Reconstruction by Error-Corrected Circulating Tumor DNA Sequencing.Clin Chem. 2018 Nov;64(11):1626-1635. doi: 10.1373/clinchem.2018.289629. Epub 2018 Aug 27. Clin Chem. 2018. PMID: 30150316 Free PMC article. Clinical Trial.
-
Hydro-Seq enables contamination-free high-throughput single-cell RNA-sequencing for circulating tumor cells.Nat Commun. 2019 May 15;10(1):2163. doi: 10.1038/s41467-019-10122-2. Nat Commun. 2019. PMID: 31092822 Free PMC article.
-
Deciphering cell-cell interactions and communication in the tumor microenvironment and unraveling intratumoral genetic heterogeneity via single-cell genomic sequencing.Bioengineered. 2022 Jul-Dec;13(7-12):14974-14986. doi: 10.1080/21655979.2023.2185434. Bioengineered. 2022. PMID: 37105769 Free PMC article. Review.
-
Single-cell RNA sequencing in breast cancer: Understanding tumor heterogeneity and paving roads to individualized therapy.Cancer Commun (Lond). 2020 Aug;40(8):329-344. doi: 10.1002/cac2.12078. Epub 2020 Jul 12. Cancer Commun (Lond). 2020. PMID: 32654419 Free PMC article. Review.
Cited by
-
Exploring long non-coding RNA networks from single cell omics data.Comput Struct Biotechnol J. 2022 Aug 4;20:4381-4389. doi: 10.1016/j.csbj.2022.08.003. eCollection 2022. Comput Struct Biotechnol J. 2022. PMID: 36051880 Free PMC article. Review.
-
Deconstructing Intratumoral Heterogeneity through Multiomic and Multiscale Analysis of Serial Sections.Cancers (Basel). 2024 Jul 1;16(13):2429. doi: 10.3390/cancers16132429. Cancers (Basel). 2024. PMID: 39001492 Free PMC article.
-
Single Cell RNA-Sequencing for the Study of Atherosclerosis.J Lipid Atheroscler. 2019 Sep;8(2):152-161. doi: 10.12997/jla.2019.8.2.152. Epub 2019 Jul 30. J Lipid Atheroscler. 2019. PMID: 32821705 Free PMC article. Review.
-
Early activation of inflammatory pathways in UBA1-mutated hematopoietic stem and progenitor cells in VEXAS.Cell Rep Med. 2023 Aug 15;4(8):101160. doi: 10.1016/j.xcrm.2023.101160. Cell Rep Med. 2023. PMID: 37586319 Free PMC article.
-
Spatial transcriptomics: recent developments and insights in respiratory research.Mil Med Res. 2023 Aug 17;10(1):38. doi: 10.1186/s40779-023-00471-x. Mil Med Res. 2023. PMID: 37592342 Free PMC article. Review.
References
-
- Cheow L.F., Courtois E.T., Tan Y., Viswanathan R., Xing Q., Tan R.Z., Tan D.S., Robson P., Loh Y.H., Quake S.R., Burkholder W.F. Single-cell multimodal profiling reveals cellular epigenetic heterogeneity. Nat. Methods. 2016;13:833–836. - PubMed
-
- Clevers H. The cancer stem cell: premises, promises and challenges. Nat. Med. 2011;17:313–319. - PubMed
Publication types
MeSH terms
Substances
Grants and funding
- 27125/CRUK_/Cancer Research UK/United Kingdom
- MC_UU_12009/5/MRC_/Medical Research Council/United Kingdom
- G0902418/MRC_/Medical Research Council/United Kingdom
- MR/L006340/1/MRC_/Medical Research Council/United Kingdom
- MC_PC_12020/MRC_/Medical Research Council/United Kingdom
- G84/6443/MRC_/Medical Research Council/United Kingdom
- G0501838/MRC_/Medical Research Council/United Kingdom
- MC_UU_00016/15/MRC_/Medical Research Council/United Kingdom
- MC_UU_12009/16/MRC_/Medical Research Council/United Kingdom
- 29034/CRUK_/Cancer Research UK/United Kingdom
- MC_UU_00016/5/MRC_/Medical Research Council/United Kingdom
- G0801073/MRC_/Medical Research Council/United Kingdom
- 27723/CRUK_/Cancer Research UK/United Kingdom
- 26988/CRUK_/Cancer Research UK/United Kingdom
- 28051/CRUK_/Cancer Research UK/United Kingdom
- MR/M00919X/1/MRC_/Medical Research Council/United Kingdom
LinkOut - more resources
Full Text Sources
Other Literature Sources
Medical
Molecular Biology Databases
Miscellaneous