Lung Cancer Screening, Towards a Multidimensional Approach: Why and How?
- PMID: 30759893
- PMCID: PMC6406662
- DOI: 10.3390/cancers11020212
Lung Cancer Screening, Towards a Multidimensional Approach: Why and How?
Abstract
Early-stage treatment improves prognosis of lung cancer and two large randomized controlled trials have shown that early detection with low-dose computed tomography (LDCT) reduces mortality. Despite this, lung cancer screening (LCS) remains challenging. In the context of a global shortage of radiologists, the high rate of false-positive LDCT results in overloading of existing lung cancer clinics and multidisciplinary teams. Thus, to provide patients with earlier access to life-saving surgical interventions, there is an urgent need to improve LDCT-based LCS and especially to reduce the false-positive rate that plagues the current detection technology. In this context, LCS can be improved in three ways: (1) by refining selection criteria (risk factor assessment), (2) by using Computer Aided Diagnosis (CAD) to make it easier to interpret chest CTs, and (3) by using biological blood signatures for early cancer detection, to both spot the optimal target population and help classify lung nodules. These three main ways of improving LCS are discussed in this review.
Keywords: artificial intelligence; lung cancer; screening.
Conflict of interest statement
The authors declare no conflict of interest.
Figures
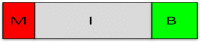
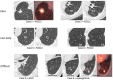
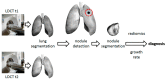
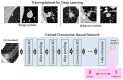
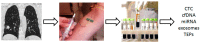
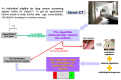
Similar articles
-
The effect of direct referral for fast CT scan in early lung cancer detection in general practice. A clinical, cluster-randomised trial.Dan Med J. 2015 Mar;62(3):B5027. Dan Med J. 2015. PMID: 25748876 Clinical Trial.
-
The Potential Role of Artificial Intelligence in Lung Cancer Screening Using Low-Dose Computed Tomography.Diagnostics (Basel). 2022 Oct 8;12(10):2435. doi: 10.3390/diagnostics12102435. Diagnostics (Basel). 2022. PMID: 36292124 Free PMC article. Review.
-
Screening for Lung Cancer: Systematic Review to Update the U.S. Preventive Services Task Force Recommendation [Internet].Rockville (MD): Agency for Healthcare Research and Quality (US); 2013 Jul. Report No.: 13-05188-EF-1. Rockville (MD): Agency for Healthcare Research and Quality (US); 2013 Jul. Report No.: 13-05188-EF-1. PMID: 24027793 Free Books & Documents. Review.
-
Radiation burden and associated cancer risk for a typical population to be screened for lung cancer with low-dose CT: A phantom study.Eur Radiol. 2018 Oct;28(10):4370-4378. doi: 10.1007/s00330-018-5373-7. Epub 2018 Apr 12. Eur Radiol. 2018. PMID: 29651767
-
Detection of Subsolid Nodules in Lung Cancer Screening: Complementary Sensitivity of Visual Reading and Computer-Aided Diagnosis.Invest Radiol. 2018 Aug;53(8):441-449. doi: 10.1097/RLI.0000000000000464. Invest Radiol. 2018. PMID: 29543693
Cited by
-
Thermal Liquid Biopsy (TLB): A Predictive Score Derived from Serum Thermograms as a Clinical Tool for Screening Lung Cancer Patients.Cancers (Basel). 2019 Jul 19;11(7):1012. doi: 10.3390/cancers11071012. Cancers (Basel). 2019. PMID: 31331013 Free PMC article.
-
Management of incidental nodules in lung cancer screening: ready for prime-time?Breathe (Sheff). 2019 Dec;15(4):346-349. doi: 10.1183/20734735.0247-2019. Breathe (Sheff). 2019. PMID: 31803272 Free PMC article.
-
Matched tissue and liquid biopsies for advanced non-small cell lung cancer patients A potentially indispensable complementary approach.Transl Oncol. 2023 Sep;35:101735. doi: 10.1016/j.tranon.2023.101735. Epub 2023 Jul 4. Transl Oncol. 2023. PMID: 37413719 Free PMC article.
-
Histopathological Imaging⁻Environment Interactions in Cancer Modeling.Cancers (Basel). 2019 Apr 24;11(4):579. doi: 10.3390/cancers11040579. Cancers (Basel). 2019. PMID: 31022926 Free PMC article.
-
Assisted versus Manual Interpretation of Low-Dose CT Scans for Lung Cancer Screening: Impact on Lung-RADS Agreement.Radiol Imaging Cancer. 2021 Sep;3(5):e200160. doi: 10.1148/rycan.2021200160. Radiol Imaging Cancer. 2021. PMID: 34559005 Free PMC article.
References
-
- National Lung Screening Trial Research Team. Aberle D.R., Adams A.M., Berg C.D., Black W.C., Clapp J.D., Fagerstrom R.M., Gareen I.F., Gatsonis C., Marcus P.M., et al. Reduced lung-cancer mortality with low-dose computed tomographic screening. N. Engl. J. Med. 2011;365:395–409. doi: 10.1056/NEJMoa1208962. - DOI - PMC - PubMed
-
- De Koning H.J., Van Der Aalst C.M., Ten Haaf K., Oudkerk M. PL02.05. Effects of Volume CT Lung Cancer Screening: Mortality Results of the NELSON Randomised-Controlled Population Based Trial. J. Thorac. Oncol. 2018;13:S185. doi: 10.1016/j.jtho.2018.08.012. - DOI
-
- CMS.gov. [(accessed on 3 February 2019)]; Available online: https://www.cms.gov/medicare-coverage-database/details/nca-decision-memo....
Publication types
Grants and funding
LinkOut - more resources
Full Text Sources
Research Materials
Miscellaneous