Artificial intelligence in cancer imaging: Clinical challenges and applications
- PMID: 30720861
- PMCID: PMC6403009
- DOI: 10.3322/caac.21552
Artificial intelligence in cancer imaging: Clinical challenges and applications
Abstract
Judgement, as one of the core tenets of medicine, relies upon the integration of multilayered data with nuanced decision making. Cancer offers a unique context for medical decisions given not only its variegated forms with evolution of disease but also the need to take into account the individual condition of patients, their ability to receive treatment, and their responses to treatment. Challenges remain in the accurate detection, characterization, and monitoring of cancers despite improved technologies. Radiographic assessment of disease most commonly relies upon visual evaluations, the interpretations of which may be augmented by advanced computational analyses. In particular, artificial intelligence (AI) promises to make great strides in the qualitative interpretation of cancer imaging by expert clinicians, including volumetric delineation of tumors over time, extrapolation of the tumor genotype and biological course from its radiographic phenotype, prediction of clinical outcome, and assessment of the impact of disease and treatment on adjacent organs. AI may automate processes in the initial interpretation of images and shift the clinical workflow of radiographic detection, management decisions on whether or not to administer an intervention, and subsequent observation to a yet to be envisioned paradigm. Here, the authors review the current state of AI as applied to medical imaging of cancer and describe advances in 4 tumor types (lung, brain, breast, and prostate) to illustrate how common clinical problems are being addressed. Although most studies evaluating AI applications in oncology to date have not been vigorously validated for reproducibility and generalizability, the results do highlight increasingly concerted efforts in pushing AI technology to clinical use and to impact future directions in cancer care.
Keywords: artificial intelligence; cancer imaging; clinical challenges; deep learning; radiomics.
© 2019 American Cancer Society.
Figures
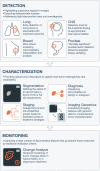
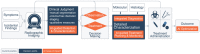
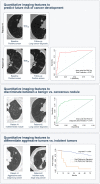
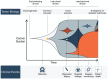
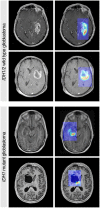
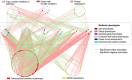
Similar articles
-
Artificial Intelligence-Driven Radiomics in Head and Neck Cancer: Current Status and Future Prospects.Int J Med Inform. 2024 Aug;188:105464. doi: 10.1016/j.ijmedinf.2024.105464. Epub 2024 Apr 23. Int J Med Inform. 2024. PMID: 38728812 Review.
-
Artificial intelligence in liver imaging: methods and applications.Hepatol Int. 2024 Apr;18(2):422-434. doi: 10.1007/s12072-023-10630-w. Epub 2024 Feb 20. Hepatol Int. 2024. PMID: 38376649 Review.
-
Artificial Intelligence in Lung Cancer: Bridging the Gap Between Computational Power and Clinical Decision-Making.Can Assoc Radiol J. 2021 Feb;72(1):86-97. doi: 10.1177/0846537120941434. Epub 2020 Jul 31. Can Assoc Radiol J. 2021. PMID: 32735493 Review.
-
Predicting gene mutation status via artificial intelligence technologies based on multimodal integration (MMI) to advance precision oncology.Semin Cancer Biol. 2023 Jun;91:1-15. doi: 10.1016/j.semcancer.2023.02.006. Epub 2023 Feb 19. Semin Cancer Biol. 2023. PMID: 36801447 Review.
-
Artificial Intelligence in Lung Imaging.Semin Respir Crit Care Med. 2022 Dec;43(6):946-960. doi: 10.1055/s-0042-1755571. Epub 2022 Sep 29. Semin Respir Crit Care Med. 2022. PMID: 36174647 Review.
Cited by
-
Role of Artificial intelligence model in prediction of low back pain using T2 weighted MRI of Lumbar spine.F1000Res. 2024 Oct 10;13:1035. doi: 10.12688/f1000research.154680.2. eCollection 2024. F1000Res. 2024. PMID: 39483709 Free PMC article.
-
A review on optimization of Wilms tumour management using radiomics.BJR Open. 2024 Oct 8;6(1):tzae034. doi: 10.1093/bjro/tzae034. eCollection 2024 Jan. BJR Open. 2024. PMID: 39483333 Free PMC article. Review.
-
Enhanced WGAN Model for Diagnosing Laryngeal Carcinoma.Cancers (Basel). 2024 Oct 14;16(20):3482. doi: 10.3390/cancers16203482. Cancers (Basel). 2024. PMID: 39456576 Free PMC article.
-
Role of Radiology in the Diagnosis and Treatment of Breast Cancer in Women: A Comprehensive Review.Cureus. 2024 Sep 24;16(9):e70097. doi: 10.7759/cureus.70097. eCollection 2024 Sep. Cureus. 2024. PMID: 39449897 Free PMC article. Review.
-
Future implications of artificial intelligence in lung cancer screening: a systematic review.BJR Open. 2024 Oct 15;6(1):tzae035. doi: 10.1093/bjro/tzae035. eCollection 2024 Jan. BJR Open. 2024. PMID: 39444460 Free PMC article.
References
-
- LeCun Y, Bengio Y, Hinton G. Deep learning. Nature. 2015;521;436‐444. - PubMed
-
- Rolnick D, Veit A, Belongie S, Shavit N. Deep learning is robust to massive label noise. arXiv:1705.10694 [cs.LG]; 2017. arxiv.org/abs/1705.10694. Accessed May 28, 2018
-
- Moeskops P, Wolterink JM, van der Velden BHM, et al. Deep learning for multi‐task medical image segmentation in multiple modalities In: Ourselin S, Joskowicz L, Sabuncu MR, Unal G, Wells W, eds. Medical Image Computing and Computer‐Assisted Intervention‐MICCAI 2016. 19th International Conference, Athens, Greece, October 17–21, 2016, Proceedings. Cham, Switzerland: Springer International Publishing AG; 2016:478‐486.
Publication types
MeSH terms
Grants and funding
- FC001202/MRC_/Medical Research Council/United Kingdom
- DH_/Department of Health/United Kingdom
- FC001202/WT_/Wellcome Trust/United Kingdom
- U01 CA143062/CA/NCI NIH HHS/United States
- U01 CA189240/CA/NCI NIH HHS/United States
- FC001169/MRC_/Medical Research Council/United Kingdom
- WT_/Wellcome Trust/United Kingdom
- U01 CA195564/CA/NCI NIH HHS/United States
- U01 CA196405/CA/NCI NIH HHS/United States
- P41 EB015898/EB/NIBIB NIH HHS/United States
- R01 CA166945/CA/NCI NIH HHS/United States
- CRUK_/Cancer Research UK/United Kingdom
- FC001169/WT_/Wellcome Trust/United Kingdom
- FC001202/ARC_/Arthritis Research UK/United Kingdom
- U01 CA186145/CA/NCI NIH HHS/United States
- U01 CA200464/CA/NCI NIH HHS/United States
- U01 CA190234/CA/NCI NIH HHS/United States
- U24 CA194354/CA/NCI NIH HHS/United States
LinkOut - more resources
Full Text Sources
Medical