Fold-Change Detection of NF-κB at Target Genes with Different Transcript Outputs
- PMID: 30704857
- PMCID: PMC6382958
- DOI: 10.1016/j.bpj.2019.01.011
Fold-Change Detection of NF-κB at Target Genes with Different Transcript Outputs
Abstract
The transcription factor nuclear factor (NF)-κB promotes inflammatory and stress-responsive gene transcription across a range of cell types in response to the cytokine tumor necrosis factor (TNF). Although NF-κB signaling exhibits significant variability across single cells, some target genes supporting high levels of TNF-inducible transcription exhibit fold-change detection of NF-κB, which may buffer against stochastic variation in signaling molecules. It is unknown whether fold-change detection is maintained at NF-κB target genes with low levels of TNF-inducible transcription, for which stochastic promoter events may be more pronounced. Here, we used a microfluidic cell-trapping device to measure how TNF-induced activation of NF-κB controls transcription in single Jurkat T cells at the promoters of integrated HIV and the endogenous cytokine gene IL6, which produce only a few transcripts per cell. We tracked TNF-stimulated NF-κB RelA nuclear translocation by live-cell imaging and then quantified transcript number by RNA FISH in the same cell. We found that TNF-induced transcript abundance at 2 h for low- and high-abundance target genes correlates with similar strength with the fold change in nuclear NF-κB. A computational model of TNF-NF-κB signaling, which implements fold-change detection from competition for binding to κB motifs, could reproduce fold-change detection across the experimentally measured range of transcript outputs. However, multiple model parameters affecting transcription had to be simultaneously varied across promoters to maintain fold-change detection while also matching other trends in the single-cell data for low-abundance transcripts. Our results suggest that cells use multiple biological mechanisms to tune transcriptional output while maintaining robustness of NF-κB fold-change detection.
Copyright © 2019 Biophysical Society. Published by Elsevier Inc. All rights reserved.
Figures
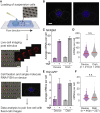
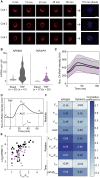
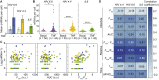
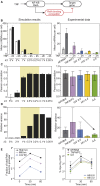
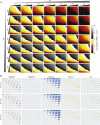
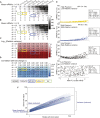
Similar articles
-
Fold change of nuclear NF-κB determines TNF-induced transcription in single cells.Mol Cell. 2014 Mar 20;53(6):867-79. doi: 10.1016/j.molcel.2014.01.026. Epub 2014 Feb 13. Mol Cell. 2014. PMID: 24530305 Free PMC article.
-
TNF-alpha-induced NF-kappaB/RelA Ser(276) phosphorylation and enhanceosome formation is mediated by an ROS-dependent PKAc pathway.Cell Signal. 2007 Jul;19(7):1419-33. doi: 10.1016/j.cellsig.2007.01.020. Epub 2007 Jan 25. Cell Signal. 2007. PMID: 17317104
-
Identification of direct genomic targets downstream of the nuclear factor-kappaB transcription factor mediating tumor necrosis factor signaling.J Biol Chem. 2005 Apr 29;280(17):17435-48. doi: 10.1074/jbc.M500437200. Epub 2005 Feb 18. J Biol Chem. 2005. PMID: 15722553
-
Binding loci of RelA-containing nuclear factor-kappaB dimers in promoter regions of PHM1-31 myometrial smooth muscle cells.Mol Hum Reprod. 2015 Nov;21(11):865-83. doi: 10.1093/molehr/gav051. Epub 2015 Sep 23. Mol Hum Reprod. 2015. PMID: 26405173
-
Monitoring the Levels of Cellular NF-κB Activation States.Cancers (Basel). 2021 Oct 26;13(21):5351. doi: 10.3390/cancers13215351. Cancers (Basel). 2021. PMID: 34771516 Free PMC article. Review.
Cited by
-
Direct observation correlates NFκB cRel in B cells with activating and terminating their proliferative program.Proc Natl Acad Sci U S A. 2024 Jul 23;121(30):e2309686121. doi: 10.1073/pnas.2309686121. Epub 2024 Jul 18. Proc Natl Acad Sci U S A. 2024. PMID: 39024115 Free PMC article.
-
TNFR1 mediates heterogeneity in single-cell NF-κB activation.iScience. 2024 Mar 11;27(4):109486. doi: 10.1016/j.isci.2024.109486. eCollection 2024 Apr 19. iScience. 2024. PMID: 38551009 Free PMC article.
-
TNF stimulation primarily modulates transcriptional burst size of NF-κB-regulated genes.Mol Syst Biol. 2021 Jul;17(7):e10127. doi: 10.15252/msb.202010127. Mol Syst Biol. 2021. PMID: 34288498 Free PMC article.
-
Techniques for Studying Decoding of Single Cell Dynamics.Front Immunol. 2019 Apr 11;10:755. doi: 10.3389/fimmu.2019.00755. eCollection 2019. Front Immunol. 2019. PMID: 31031756 Free PMC article. Review.
-
Transcriptional kinetic synergy: A complex landscape revealed by integrating modeling and synthetic biology.Cell Syst. 2023 Apr 19;14(4):324-339.e7. doi: 10.1016/j.cels.2023.02.003. Cell Syst. 2023. PMID: 37080164 Free PMC article.
References
-
- Aggarwal B.B. Signalling pathways of the TNF superfamily: a double-edged sword. Nat. Rev. Immunol. 2003;3:745–756. - PubMed
Publication types
MeSH terms
Substances
Grants and funding
LinkOut - more resources
Full Text Sources