Role of tumor-associated neutrophils in regulation of tumor growth in lung cancer development: A mathematical model
- PMID: 30689655
- PMCID: PMC6349324
- DOI: 10.1371/journal.pone.0211041
Role of tumor-associated neutrophils in regulation of tumor growth in lung cancer development: A mathematical model
Abstract
Neutrophils display rapid and potent innate immune responses in various diseases. Tumor-associated neutrophils (TANs) however either induce or overcome immunosuppressive functions of the tumor microenvironment through complex tumor-stroma crosstalk. We developed a mathematical model to address the question of how phenotypic alterations between tumor suppressive N1 TANS, and tumor promoting N2 TANs affect nonlinear tumor growth in a complex tumor microenvironment. The model provides a visual display of the complex behavior of populations of TANs and tumors in response to various TGF-β and IFN-β stimuli. In addition, the effect of anti-tumor drug administration is incorporated in the model in an effort to achieve optimal anti-tumor efficacy. The simulation results from the mathematical model were in good agreement with experimental data. We found that the N2-to-N1 ratio (N21R) index is positively correlated with aggressive tumor growth, suggesting that this may be a good prognostic factor. We also found that the antitumor efficacy increases when the relative ratio (Dap) of delayed apoptotic cell death of N1 and N2 TANs is either very small or relatively large, providing a basis for therapeutically targeting prometastatic N2 TANs.
Conflict of interest statement
The authors have declared that no competing interests exist.
Figures
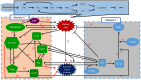
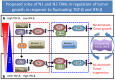
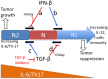
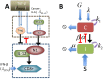
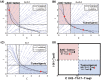
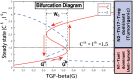
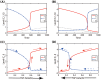
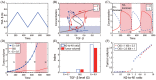
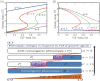
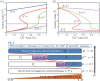
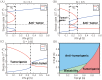
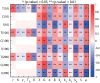
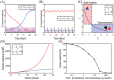
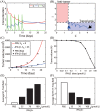
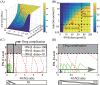
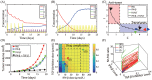
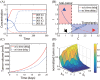
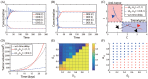
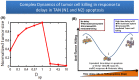
Similar articles
-
The Role of Tumor-Associated Neutrophils in Colorectal Cancer.Int J Mol Sci. 2019 Jan 27;20(3):529. doi: 10.3390/ijms20030529. Int J Mol Sci. 2019. PMID: 30691207 Free PMC article. Review.
-
Smad3 is essential for polarization of tumor-associated neutrophils in non-small cell lung carcinoma.Nat Commun. 2023 Mar 31;14(1):1794. doi: 10.1038/s41467-023-37515-8. Nat Commun. 2023. PMID: 37002229 Free PMC article.
-
Tumor-associated neutrophils display a distinct N1 profile following TGFβ modulation: A transcriptomics analysis of pro- vs. antitumor TANs.Oncoimmunology. 2016 Sep 13;5(11):e1232221. doi: 10.1080/2162402X.2016.1232221. eCollection 2016. Oncoimmunology. 2016. PMID: 27999744 Free PMC article.
-
Tissue-infiltrating neutrophils constitute the major in vivo source of angiogenesis-inducing MMP-9 in the tumor microenvironment.Neoplasia. 2014 Oct 23;16(10):771-88. doi: 10.1016/j.neo.2014.08.013. eCollection 2014 Oct. Neoplasia. 2014. PMID: 25379015 Free PMC article.
-
Rational targeting of immunosuppressive neutrophils in cancer.Pharmacol Ther. 2020 Aug;212:107556. doi: 10.1016/j.pharmthera.2020.107556. Epub 2020 Apr 25. Pharmacol Ther. 2020. PMID: 32343986 Review.
Cited by
-
Immune microenvironment composition in non-small cell lung cancer and its association with survival.Clin Transl Immunology. 2020 Jun 12;9(6):e1142. doi: 10.1002/cti2.1142. eCollection 2020. Clin Transl Immunology. 2020. PMID: 32547744 Free PMC article.
-
Role of neutrophil extracellular traps in regulation of lung cancer invasion and metastasis: Structural insights from a computational model.PLoS Comput Biol. 2021 Feb 17;17(2):e1008257. doi: 10.1371/journal.pcbi.1008257. eCollection 2021 Feb. PLoS Comput Biol. 2021. PMID: 33596197 Free PMC article.
-
The oncolytic bacteria-mediated delivery system of CCDC25 nucleic acid drug inhibits neutrophil extracellular traps induced tumor metastasis.J Nanobiotechnology. 2024 Feb 19;22(1):69. doi: 10.1186/s12951-024-02335-5. J Nanobiotechnology. 2024. PMID: 38369519 Free PMC article.
-
Two-Faced Roles of Tumor-Associated Neutrophils in Cancer Development and Progression.Int J Mol Sci. 2020 May 14;21(10):3457. doi: 10.3390/ijms21103457. Int J Mol Sci. 2020. PMID: 32422991 Free PMC article. Review.
-
The role of innate immune cells in the tumor microenvironment and research progress in anti-tumor therapy.Front Immunol. 2023 Jan 19;13:1039260. doi: 10.3389/fimmu.2022.1039260. eCollection 2022. Front Immunol. 2023. PMID: 36741415 Free PMC article. Review.
References
Publication types
MeSH terms
Substances
Grants and funding
LinkOut - more resources
Full Text Sources
Medical